Q1'23: Technology update – low precision and model optimization
Authors
Alexander Kozlov, Nikolay Lyalyushkin, Pablo Munoz, Vui Seng Chua, Alexander Suslov, Yury Gorbachev, Nilesh Jain
Summary
We continue following the trends and reviewing papers and posts for your convenience. This quarter we observed quite a lot of new methods, and one of the main focuses is the optimization of Large Language Models which are started being adopted by the industry. Please pay attention to Token Merging, GPTQ, and FlexGen works which introduce interesting methods and show very promising results.
Papers with notable results
Quantization
- CSMPQ: CLASS SEPARABILITYBASED MIXED-PRECISION QUANTIZATION by universities of China (https://arxiv.org/pdf/2212.10220.pdf). The paper introduces the class separability of layer-wise feature maps to search for optimal quantization bit-width. Essentially, authors leverage the TF-IDF metric from NLP to measure the class separability of layer-wise feature maps that are averaged across spatial dimensions. The method can be applied on top of the existing quantization algorithms, such as BRECQ and delivers good results, e.g., 71.30% top-1 acc with only 1.5Mb on MobileNetV2.
- Understanding INT4 Quantization for Transformer Models: Latency Speedup, Composability, and Failure Cases by Microsoft (https://arxiv.org/abs/2301.12017). Show that INT4 quantization for LM does not greatly reduce the quality of encoder-only and encoder-decoder models (e.g. BERT, BART). Even with 50%sparsity accuracy drop is within 1% on MNLI. The authors provide an analysis of problems with decoder-only models (e.g., GPT). The method will be part of DeepSpeed.
- A Practical Mixed Precision Algorithm for Post-Training Quantization by Qualcomm AI Research (https://arxiv.org/pdf/2302.05397.pdf). In this paper, authors propose two-phase algorithm to solve the problem of mixed precision quantization in the post-training quantization setting. In the first phase, they create a per-layer sensitivity list by measuring the loss(SQNR) of the entire network with different quantization options for each layer. The second phase of the algorithm starts with the entire network quantized to the highest possible bitwidth, after which based on the sensitivity list created in phase 1, they iteratively flip the least sensitive quantizers to lower bit-width options until the performance budget is met or our accuracy requirement gets violated. The method shows comparable results for various models including CV and NLP.
- LUT-NN: Towards Unified Neural Network Inference by Table Lookup by Microsoft Research, Chinese Universities (https://arxiv.org/abs/2302.03213). Development of the idea of product quantization "multiplications without multiplications" – pre-calculate multiplications of "typical" numbers and in runtime, instead of multiplication and addition they do a lookup in the table. The accuracy is lower than the baseline networks, but way better than in previous methods. Latency-wise, the real speedup of LUT-NN is up to 7x for BERT and 2x for ResNet on CPU.
- Oscillation-free Quantization for Low-bit Vision Transformers by Hong Kong University of Science and Technology and Reality Labs, Meta (https://arxiv.org/pdf/2302.02210.pdf). In this work, authors are aiming at ultra-low-bit quantization of vision transformer models. They propose three techniques to address the problem of weight oscillation when quantizing to low-bits: statistical weight quantization to improve quantization robustness compared to the prevalent learnable-scale-based method; confidence-guided annealing that freeze sthe weights with high confidence and calms the oscillating weights; and query-key reparameterization to resolve the query-key intertwined oscillation and mitigate the resulting gradient misestimation. The method shows state-of-the-art results when quantizing DeiT-T/DeiT-S models to 2 and 4 bits.
- Mixed Precision Post Training Quantization of Neural Networks with Sensitivity Guided Search by University of Notre Dame and Google (https://arxiv.org/pdf/2302.01382.pdf). Authors are aiming at building an optimal bitwidth search algorithm. They conduct an analysis of metrics to of quantization error as well as two sensitivity-guided search algorithms. They found that a combination of Hessian trace + Gready search gives the best results in their setup. Experimental results show latency reductions of up to 27.59% (ResNet50) and 34.31% (BERT).
- Teacher Intervention: Improving Convergence of Quantization Aware Training for Ultra-Low Precision Transformers by Hanyang University and Seoul National Universities (https://arxiv.org/pdf/2302.11812.pdf).One more paper that claims benefits from knowledge distillation between intermediate layers of Transformer models during optimization. In this case, authors apply Quantization-aware Training at ultra-low bit width setup (e.g. ternary quantization). They perform an extensive analysis of KD on the training stability and convergence at multiple settings and do evaluation both on NLP and CV Transformer models.
- POWERQUANT: AUTOMORPHISMSEARCH FOR NONUNIFORM QUANTIZATION by Sorbonne University and Datakalab (https://arxiv.org/pdf/2301.09858.pdf). The paper proposes a non-uniform data-free quantization method that is, essentially, a modification of uniform quantization with exponent parameter alpha that is tuned during the quantization process. The method shows its effectiveness when applying 8 and 4 bits quantization to various types of models including Conv, RNN and Transformer models.
- GPTQ: Accurate Post-Training Quantization for Generative Pre-trained Transformers by IST Austria, ETH Zurich, and Neural Magic (https://arxiv.org/abs/2210.17323). Authors argue that contemporary PTQ methods such as AdaRound, BRECQ, ZeroQuant are too costly to quantize massive-scale LLM. GPTQ is an extension of Hessian-based post-training quantization method, Optimal Brain Quantization(OBQ) to scale up the process efficiently for billion parameters LLM which takes only minutes to quantize weight of 3 billion GPT and 4 hours for OPT-175Bon a single A100 GPU. The papers show that 3-bit weight quantized OPT-175B can be fit into a single 80GB A100 which would otherwise require 5xA100 for FP16,4xA100 for Int8 (SmoothQuant).The optimized model achieves >3X latency improvement with a custom dequantization kernel for FP16 inference. Although the work does not map to Int8 engine, it is a strong indication that mix low-bit weight (<8bit) and8-bit activation could further alleviate the memory footprint and bandwidth bottleneck in LLM by incurring a low-overhead weight dequantization. Code is available at: https://github.com/IST-DASLab/gptq.
Pruning
- SparseGPT: Massive Language Models Can Be Accurately Pruned In One-shot by IST Austria and Neural Magic (https://arxiv.org/abs/2301.00774). The layer-wise pruning decisions are based on series of careful approximations of the inverse Hessian of the data. LLM can be pruned to at least 50% sparsity in one-shot, without any retraining, at minimal loss of accuracy for LLM. SparseGPT generalizes to semi-structured (2:4 and 4:8) patterns and is compatible with weight quantization approaches.
- ZipLM: Hardware-Aware Structured Pruning of Language Models by IST Austria and Neural Magic (https://arxiv.org/pdf/2302.04089.pdf). The idea is to prune gradually based on measured latency for different number of attention heads and FFN shapes. The pruning decisions are based on estimation of the inverse Hessian of the data. Using it they obtain the optimal layer-wise mask and weight update to preserve original intermediate outputs. To recover accuracy after pruning they tune with 2 distillation losses: with teacher outputs and with intermediate token representations across the entire model. 2x faster BERT-large than the Block Movement Pruning algorithm for the same accuracy. ZipLM can match the performance of highly optimized MobileBERT model by simply compressing the baseline BERT architecture. Authors plan to open-source the framework as part of SparseML.
- R-TOSS: A Framework for Real-Time Object Detection using Semi-Structured Pruning by Colorado State University (https://arxiv.org/ftp/arxiv/papers/2303/2303.02191.pdf). A practical study on semi-structured pruning of ConvNets. Authors propose a method that generates a set of sparse patterns for the model and applies them to introduce the sparsity during the training. The same set is passed to the runtime to precompile the sparse kernels. They also propose a way how to spread the same idea to 1x1 Convs that are dominant in contemporary architectures. The method is applied to YOLOv5 and RetinaNet models and its efficiency is evaluated on Jetson TX2 platform.
- Dynamic Structure Pruning for Compressing CNNs by Korea University (https://arxiv.org/pdf/2303.09736.pdf). Interesting work on the structured pruning where all the filters of each operation are being split into the groups and each group is pruned independently along the input channel dimension. One can imagine that each operation is being split into several operations and each operates on its own portion of input channels (ala grouped convolution). Authors also propose a differentiable group learning method that can optimize filter groups using gradient-based methods during training. The method shows better efficiency compared to Filter pruning methods. Code is available at https://github.com/irishev/DSP.

- Automatic Attention Pruning: Improving and Automating Model Pruning using Attentions by Arizona State University and Meta (https://arxiv.org/pdf/2201.10520.pdf). Authors propose an iterative, structured pruning approach for finding the “winning ticket” models that are hardware efficient. They also implement an attention-based mechanism for accurately identifying unimportant filters for pruning, which is much more effective than existing methods as well as an adaptive pruning method that can automatically optimize the pruning process according to diverse real-world scenarios. Method shows comparable results for a variety of CV model architectures. Code is at: https://github.com/kaiqi123/Automatic-Attention-Pruning.git.
- Efficient Spatially Sparse Inference for Conditional GANs and Diffusion Models by CMU, MITand Stanford University. Motivated by the high unedited region during interactive image editing that translates to activation sparsity relative to previous generation, the authors propose Sparse Incremental Generative Engine (SIGE). SIGE employs tile-based sparse convolution to compute modified region in input activation and update to the cached output activation of the previous generation. SIGE is intelligently designed with joint Scatter-Gather kernel to avoid memory overheads and fuses element-wise operations. The paper shows superior synthesis fidelity (PSNR,LPIPS, FID) for the task of incremental inpainting as compared to weight pruning at similar MAC reduction ratio. The authors also extensively benchmark latency of SIGE applied to DDIM, PD, GauGan on Nvidia RTXs, Apple M1 Pro and Intel i9 workstation. Speedup can be up to 14X depending on percentage of edited region. Code: https://github.com/lmxyy/sige.
- Token Merging: Your ViT but faster by Georgia Tech and Meta AI (https://arxiv.org/pdf/2210.09461.pdf). As opposed to token pruning, the authors unveil runtime token merging (ToMe) modules inserted between attention and feed forward layer in vision transformer (ViT) which reduce number of tokens successively in every transformer block up to 98% tokens in final block, easily achieve substantial acceleration up to 2X without the need to train. During runtime, ToMe employs bipartite soft matching algorithm to merge similar tokens and is as lightweight as randomly dropping tokens. When accuracy degradation is high, authors devise a training mechanism for ToMe by mapping its backpropagation like average pooling. Its training efficiency improves considerably, 1.5X as compared to learning-based token pruning. The paper shows thorough ablation on design choices of matching algorithm, token merging schedule etc. and a plethora of accuracy-speedup results on off-the-shelf ViT trained with different supervised/self-supervision for image, video, and audio data. The work is featured in Meta Research blog and claimed to accelerate Stable Diffusion’s text-to-image generation by 1.7X without loss of visual quality. Code: https://github.com/facebookresearch/ToMe.
Neural Architecture Search
- Neural Architecture Search: Insights from 1000 Papers by Universities and Abacus AI (https://arxiv.org/pdf/2301.08727.pdf). A big survey of the many recent NAS methods. The document provides a good organization of various approaches and nice illustrations of different techniques.
- Enhancing Once-For-All: A Study on Parallel Blocks, Skip Connections and Early Exits by DEIB, Politecnico di Milano (https://arxiv.org/abs/2302.01888). The authors propose OFAv2, an extension of OFA aimed at improving its performance. The extension to the original OFA includes early exits, parallel blocks and dense skip connections. The training phase is extended with two new phases: Elastic Level and Elastic Height. The authors also include a new Knowledge Distillation technique to handle multi-output networks. The results are quite impressive. In OFAMobileNetV3, OFAv2 reaches up to 12.07% improvement in accuracy compared to the original OFA.
- DDPNAS: Efficient Neural Architecture Search via Dynamic Distribution Pruning by Xiamen University and Tencent(https://link.springer.com/article/10.1007/s11263-023-01753-6). The authors propose a framework, DDPNAS, that is used to dynamically prune the search space, and accelerate the search stage. However, this acceleration requires a more complex training stage, in which to find the optimal probability distribution of possible architectures, the approach samples a set of architectures that are trained and validated, and once the distribution has been updated, the operations with the lowest probability are pruned from these arch space.
- DetOFA: Efficient Training of Once-for-All Networks for Object Detection by Using Pre-trained Supernet and Path Filter by Sony Group Corporation (https://arxiv.org/pdf/2303.13121v1.pdf).The authors propose a new performance predictor called path filter. This predictor can accurately predict the relative performance of models in the same resource bucket. Using the information obtained from path filter, DetOFA prunes the search space and reduce the computational cost of identifying good subnetworks. This approach produces better-performing super-networks for object detection and a reduction in the cost of >30% compared with the vanilla once-for-all approach.
Other
- NarrowBERT: Accelerating Masked Language Model Pretraining and Inference by University of Washington (https://arxiv.org/pdf/2301.04761.pdf). Propose two simple methods to accelerate training/inference of transformers. Utilize the idea that training prediction occurs only for masked tokens, and on inference in many problems, representation is used only for the [CLS] token. In the first approach they calculate the attention (s) on all tokens only at the beginning of the network, and then perform linear layers (f) only for the desired tokens (masked or CLS). In the second - calculate the attention (s) on all tokens only at the beginning of the network, and then generate an attention only for the necessary tokens. Shows 3.5x boost on MNLI inference.
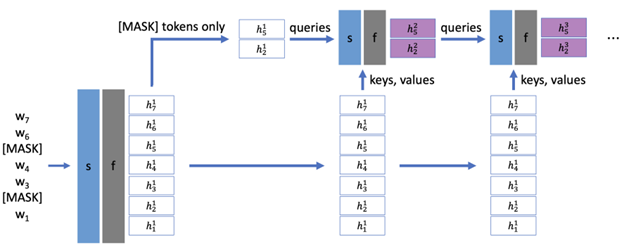
- TAILOR: Altering Skip Connections for Resource-Efficient Inference by UC San Diego, MIT and AMD (https://arxiv.org/abs/2301.07247). Continuation of the ideas of RepVGG - they remove or at least shorten the skip connection for more efficient inference: they do not store intermediate activations and save on memory. The model with the removed skip connections is distilled with a float version of itself to roughly preserve the original accuracy. The optimized hardware designs improve resource utilization by up to34% for BRAMs, 13% for FFs, and 16% for LUTs.
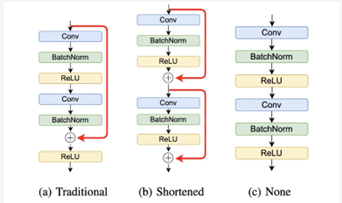
- Offsite-Tuning: Transfer Learning without Full Model by Massachusetts Institute of Technology (https://arxiv.org/pdf/2302.04870v1.pdf). In this paper, authors propose a transfer learning framework that can adapt large foundation models to downstream data without access to the full model. The setup assumes that the model owner sends a lightweight adapter and a lossy compressed emulator to the data owner, who then fine-tunes the adapter on the down stream data with the emulator’s assistance. The fine-tuned adapter is then returned to the model owner, who plugs it into the full model to create an adapted foundation model. The method can achieve comparable accuracy as full model fine-tuning while being privacy-preserving and efficient, achieving 6.5×speedup and 5.6× memory reduction. Code is available at: https://github.com/mit-han-lab/offsite-tuning.
- High-throughput Generative Inference of Large Language Models with a Single GPU by Stanford University, UC Berkeley, ETH Zurich, Yandex, HSE University, Meta, Carnegie Mellon University (https://arxiv.org/pdf/2303.06865.pdf). The paper introduces FlexGen, a high-throughput generation engine for running LLMs with limited GPU memory. It can be flexibly configured under various hardware resource constraints by aggregating memory and computation from the GPU, CPU, and disk. Through a linear programming optimizer, it searches for the best pattern to store and access the tensors, including weights, activations, and attention key/value (KV) cache. FlexGen further compresses both weights and KV cache to 4 bits with negligible accuracy loss. It achieves up to 100× higher throughput compared to state-of-the-art offloading systems. The FlexGen library runs OPT-175B up to 100× faster on a single 16GB GPU. Faster than deepspeed offloading. Code is available here: https://github.com/FMInference/FlexGen