Model Compression
Q3'24: Technology Update – Low Precision and Model Optimization
Authors
Alexander Kozlov, Nikita Savelyev, Vui Seng Chua, Souvikk Kundu, Nikolay Lyalyushkin, Andrey Anufriev, Pablo Munoz, Alexander Suslov, Liubov Talamanova, Yury Gorbachev, Nilesh Jain, Maxim Proshin
Summary
This quarter, we continue observing the trendon the optimization of LLM-based pipelines. Besides a high interest in weight quantizationto precisions beyond 4-bits, we see a lot of effort in the optimization of usageof KV-cache during the ScaledDotProduct computation: from KV-cache quantizationand decomposition to sparse attention where only a part of KV-cache is used topredict the next token. This gives the opportunity to design more efficientinference pipelines with heterogeneous execution (see RetrievalAttention work).
Highlights
- SpinQuant: LLM Quantizationwith Learned Rotations by Meta (https://arxiv.org/abs/2405.16406). Develop the idea of rotation by a random orthogonal matrix from QuIP, QuIP#, and QuaRotto reduce outliers in the LLMs and obtain better quality of W4A4KV4 quantization. The authors found that not all rotations help equally, and random rotations produce a significant variance in quantized models. Therefore, it is proposed to search for “good” rotation matrices using optimization with Cayley optimization. The matrix optimization procedure takes a little over an hour on smaller representatives of the LLama family on 8 A100 and half a day for 70B models. Regarding quality, they are ahead of baselines (the closest QuaRot is about 1% on average). Adding a rotation inside FFN gives the most significant gain. Code is available: https://github.com/facebookresearch/SpinQuant.

- ACCURATE COMPRESSION OFTEXT-TO-IMAGE DIFFUSION MODELS VIA VECTOR QUANTIZATION by Yandex Research, HSE University, Skoltech, MIPT, Neural Magic, IST Austria (https://arxiv.org/pdf/2409.00492).The authors explore vector-based PTQ strategies for text-to-image diffusion models and demonstrate that the compressed models yield higher quality text-to-image generation than the scalar alternatives under the same bit-widths. They describe an effective fine-tuning technique that further closes the gap between the full-precision and compressed models, leveraging the flexibility of the vector quantized representation. To showcase the method, they compress the weights of SDXL down to 3 bits per parameter. Extensive human evaluation and automated metrics confirm the superiority of our approach over previous diffusion compression methods under the same bit-widths. The authors illustrate that the approach can be effectively applied to distilled diffusion models, such as SDXL, which achieve nearly lossless 4-bit compression. Code is available at https://github.com/yandex-research/vqdm.
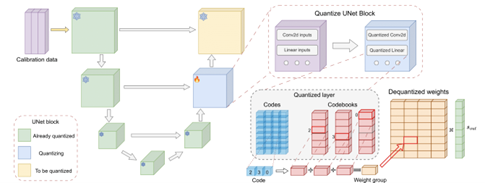
- Sparse Refinement for Efficient High-Resolution Semantic Segmentation by MIT, NVIDIA, Tsinghua University, University of Toronto, UC Berkeley (https://arxiv.org/pdf/2407.19014). Authors introduce a novel approach that enhances dense low-resolution predictions with sparse high-resolution refinements. Based on coarse low-resolution outputs, the method first uses an entropy selector to identify a sparse set of pixels with high entropy. It then employs a sparse feature extractor to generate the refinements for those pixels of interest. Finally, it leverages a gated ensembler to apply these sparse refinements to the initial coarse predictions. The method can be seamlessly integrated into any existing semantic segmentation model, regardless of CNN- or ViT-based. SparseRefine achieves significant speedup: 1.5 to 3.7 times when applied to HRNet-W48, SegFormer-B5, Mask2Former-T/L and SegNeXt-L on Cityscapes, with negligible to no loss of accuracy.

- RetrievalAttention: Accelerating Long-Context LLM Inference via Vector Retrieval by Microsoft Research, Shanghai Jiao Tong University, Fudan University (https://arxiv.org/pdf/2409.10516). Authors employ dynamic sparse attention during token generation, allowing the most critical tokens to emerge from the extensive context data. To address theOOD issue, the method constructs a vector index tailored for the attention mechanism, focusing on the distribution of queries rather than key similarities. This approach allows for traversal of only a small subset of key vectors (1% to 3%), effectively identifying the most relevant tokens to achieve accurate attention scores and results. To optimize resource utilization, RetrievalAttention retains KV vectors in the GPU memory following static patterns while offloading the majority of KV vectors to CPU memory for index construction. This strategy enables RetrievalAttention to perform attention computation with reduced latency and minimal GPU memory utilization. The method shows SOTA results in terms of latency-performance.

Papers with notable results
Quantization
- ADFQ-ViT: Activation-Distribution-Friendly Post-Training Quantization for Vision Transformers by Chinese universities (https://arxiv.org/pdf/2407.02763). Authors design the Per-Patch Outlier-aware Quantizer and the Shift-Log2 Quantizer, which addresses the challenges of outliers and irregular distributions in post-LayerNorm activations and the non-uniform distribution of positive and negative values in post-GELU activations. They also introduce the attention-score enhanced module-wise optimization, which optimizes the parameters of the weight and activation quantizer to reduce errors before and after quantization. The method shows very good results for various Vision Transformer models and use cases at W4A4 and W6A6 setups.
- How Does Quantization Affect Multilingual LLMs? by Cohere (https://arxiv.org/pdf/2407.03211). The authors investigate the problem of LLM accuracy degradation after quantization. They use automatic benchmarks, LLM-as-a-Judge methods, and human evaluation, finding that (1) harmful effects of quantization are apparent in human evaluation, and automatic metrics severely underestimate the detriment: a 1.7%average drop in Japanese across automatic tasks corresponds to a 16.0% drop reported by human evaluators on realistic prompts; (2) languages are disparately affected by quantization, with non-Latin script languages impacted worst; and (3) challenging tasks such as mathematical reasoning degrade fastest.
- CLAMP-ViT: Contrastive Data-Free Learning for Adaptive Post-Training Quantization of ViTs by Georgia Institute of Technology and Intel Labs (https://arxiv.org/pdf/2407.05266). The authors incorporate a patch-level contrastive learning scheme to generate richer, semantically meaningful data. Furthermore, they leverage contrastive learning in layer-wise evolutionary search for fixed- and mixed-precision quantization to identify optimal quantization parameters while mitigating the effects of a non-smooth loss landscape. Evaluations across various vision tasks demonstrate the superiority of CLAMP-ViT, with performance improvements of up to 3% in top-1 accuracy for classification, 0.6 mAP for object detection, and 1.5 mIoU for segmentation at a similar or better compression ratio over existing alternatives. The code is available at https://github.com/georgia-tech-synergy-lab/CLAMP-ViT.git.
- RoLoRA: Fine-tuning Rotated Outlier-free LLMs for Effective Weight-Activation Quantization by Hong Kong University of Science and Technology and Meta Reality Labs (https://arxiv.org/pdf/2407.08044).The paper proposes RoLoRA, the scheme for weight-activation quantization. RoLoRA utilizes rotation for outlier elimination and proposes rotation-aware fine-tuning to preserve the outlier-free characteristics in rotated LLMs. Experimental results show RoLoRA consistently improves low-bit LoRA convergence and post-training quantization robustness in weight-activation settings. The code is supposed to be available at https://github.com/HuangOwen/RoLoRA.
- LRQ: Optimizing Post-Training Quantization for Large Language Models by Learning Low-Rank Weight-Scaling Matrices by NAVER Cloud, KAIST AI, AITRICS, SNU AI Center (https://arxiv.org/pdf/2407.11534). The authors propose a post-training weight quantization method for LLMs that reconstructs the outputs of an intermediate Transformer block by leveraging low-rank weight-scaling matrices, replacing the conventional full weight-scaling matrices that entail as many learnable scales as their associated weights. Thanks to parameter sharing via low-rank structure, the method only needs to learn significantly fewer parameters while enabling the individual scaling of weights, thus boosting the generalization capability of quantized LLMs. Authors show the superiority of the method over prior LLM PTQ works under (i) 8-bit weight and per-tensor activation quantization, (ii) 4-bitweight and 8-bit per-token activation quantization, and (iii) low-bitweight-only quantization schemes. The code is available at https://github.com/onliwad101/FlexRound_LRQ.
- AdaLog: Post-Training Quantization for Vision Transformers with Adaptive Logarithm Quantizer by Beihang University (https://arxiv.org/pdf/2407.12951). The paper proposes a non-uniform quantizer that optimizes the logarithmic base to accommodate the power-law-like distribution of activations while simultaneously allowing for hardware-friendly quantization and dequantization. By employing the bias reparameterization, the quantizer is applicable to both the post-Softmax and post-GELU activations. The authors also develop an efficient Fast Progressive Combining Search (FPCS) strategy to determine the optimal logarithm base, as well as the scaling factors and zero points for the uniform quantizers. Experimental results on public benchmarks demonstrate promising results for various ViT-based architectures and vision tasks, especially in the W6A6setup. The code is available at https://github.com/GoatWu/AdaLog.
- RECLAIMING RESIDUAL KNOWLEDGE: A NOVEL PARADIGM TO LOW-BITQUANTIZATION by Irish Universities (https://arxiv.org/pdf/2408.00923). The authors present an efficient, low-bit, and PTQ framework for ConvNets by framing optimal quantization as an architecture search problem to re-capture quantization residual knowledge with low-rank adapters. They introduce a differentiable neural combinatorial optimization approach, searching for the optimal low-rank adapters using a smooth, high-order normalized Butterworth kernel. They also show a result, converting the weights of existing high-rank quantization residual convolutional operators to low-rank adapters without training. The method achieves good 4-bit and 3-bit quantization results by using less than 250 iterations on a small calibration set with 1600 images. Code will be open-sourced.
- VQ4DiT: Efficient Post-Training Vector Quantization for Diffusion Transformers by Zhejiang University and vivo Mobile Communication (https://arxiv.org/pdf/2408.17131). The authors explore the Vector Quantization methods for extremely low bit-width DiTs and introduce DiT-specific improvements for better quantization. They calibrate both the codebook and the assignments of each layer simultaneously. The proposed method calculates the candidate assignment set for each weight sub-vector based on Euclidean distance and reconstructs the sub-vector based on the weighted average. Then, using the zero-data and block-wise calibration method, the optimal assignment from the set is efficiently selected while calibrating the codebook. The method achieves competitive evaluation results compared to full-precision models on the ImageNet.
- MobileQuant: Mobile-friendly Quantization for On-device Language Models by Samsung AI Center, Cambridge (https://arxiv.org/pdf/2408.13933). The authors introduce a post-training quantization approach for LLMs that is supported by current mobile hardware implementations (i.e., DSP, NPU), thus being directly deployable on real-edge devices. The method improves upon prior works through simple yet effective methodological extensions that enable us to effectively quantize most activations to a lower bit-width (i.e., 8-bit) with near-lossless performance. They conduct an on-device evaluation of model accuracy, inference latency, and energy consumption. The results indicate that the proposed method reduces inference latency and energy usage by 20%-50% while still maintaining accuracy compared to models using 16-bit activations.
- Low-Bit width Floating Point Quantization for Efficient High-Quality Diffusion Models by the University of Toronto & Vector Institute (https://arxiv.org/pdf/2408.06995).The authors propose a floating-point quantization method for diffusion models that provides better image quality compared to integer quantization methods. They employ a floating-point quantization method by integrating weight rounding learning during the mapping of the full-precision values to the quantized values in the quantization process. The authors also study integer and floating-point quantization methods in state-of-the-art diffusion models. Additionally, they introduce a methodology to evaluate quantization effects, highlighting shortcomings with existing output quality metrics and experimental methodologies. Finally, their floating-point quantization method increases model sparsity by an order of magnitude, enabling further optimization opportunities.
- DopQ-ViT: Towards Distribution-Friendly and Outlier-Aware Post-Training Quantization for Vision Transformers by Institute of Automation and School of Artificial Intelligence of Chinese Academy of Sciences (https://arxiv.org/pdf/2408.03291v2).The paper focuses on the full quantization of Vision Transformers. The authors propose using the Tan Quantizer, which focuses more on values near 1, thereby better fitting the distribution of post-Softmax activations in Transformer layers. Besides, the method selects the median as the optimal scaling factor, effectively addressing the accuracy degradation issue that occurs after parametrizing post-LayerNorm activations. The method achieves very accurate results especially in W6/A6 for various tasks such as ImageNet or MS COCO.
- Differentiable Product Quantization for Memory Efficient Camera Relocalization by Czech Technical University in Prague, Aalto University, University of Oulu (https://arxiv.org/pdf/2407.15540).The authors introduce a simple and standalone metric learning for Differentiable Product Quantization for 3D scene compression that preserves matching properties of the descriptors and the final camera localization performance; ii) the proposed hybrid method enables a better tradeoff between memory complexity and localization; iii) they analyze the tradeoffs between description and map compression and show how localization is more tolerant to description compression on outdoor and indoor datasets. The code will be publicly available at https://github.com/AaltoVision/dpqe.
- Advancing Multimodal Large Language Models with Quantization-Aware Scale Learning for Efficient Adaptation by Xiamen University and SkyWork AI (https://arxiv.org/pdf/2408.03735).The paper introduces a Quantization-aware scale Learning method based on multimodal warmup. This method is grounded in two key innovations: (1) The learning of group-wise scale factors for quantized LLM weights to mitigate the quantization error arising from activation outliers and achieve more effective vision-language instruction tuning; (2) The implementation of a multimodal warmup that progressively integrates linguistic and multimodal training samples, thereby preventing overfitting of the quantized model to multimodal data while ensuring stable adaptation of multimodal large language models to downstream vision-language tasks. The code is supposed to be available at https://github.com/xjjxmu/QSLAW.
- Mamba-PTQ: Outlier Channels in Recurrent Large Language Models by Intel Labs (https://arxiv.org/pdf/2407.12397).This workshop paper is among the first to study post-training quantization on the Mamba architecture. Similar to Transformer models, it observed the presence of outlier channels in activations (those with absolute maximum values exceeding 6 standard deviations from the layer mean) and found that downstream task performance degrades substantially when these channels are removed. The study presents zero-shot results of naïve symmetrical per-tensor quantization of weights and activations across Mamba1 models, ranging from 130M to 2.8B parameters, providing a baseline for future quantization research on this emerging architecture.
- Foundation of Large Language Model Compression – Part 1: Weight Quantization by CSAIL MIT (https://arxiv.org/pdf/2409.02026).This work introduces CVXQ, a post-training weight quantization framework that assigns varying bit widths down to the per-group level, constrained by a target average bit rate per weight element. Formulated through the lens of Lagrangian convex optimization, the framework leads to a dual-ascent methods that alternately update the bit width and the tradeoff variable until all optimality conditions are met. To overcome the non-differentiability arising from discrete bit widths and considering that weight distributions are Gaussian or Laplacian, the framework leverages a well-known result from rate-distortion theory to provide closed-form derivative estimates during optimization. CVXQ adopts an interesting compounding (non-uniform) quantization, where weights are first projected to the sigmoid domain before applying uniform round-to-nearest quantization. A codebook is employed to enable dequantization via simple lookup, avoiding complex inverse computations. Tested across a wide range of model sizes in OPT and Llama2, CVXQ outperforms GPTQ, AWQ, and OWQ at 3- and 4-bit rates per weight in nearly all cases. Full implementation will be available soon here.
Pruning / Sparsity
- LazyLLM: DYNAMIC TOKEN PRUNING FOR EFFICIENT LONGCONTEXT LLM INFERENCE by Apple and Meta AI (https://arxiv.org/pdf/2407.14057). The paper introduces an LLM acceleration method that selectively computes the KV for tokens important for the next token prediction in both the prefilling and decoding stages. Contrary to static pruning approaches that prune the prompt at once, LazyLLM allows language models to dynamically select different subsets of tokens from the context in different generation steps, even though they might be pruned in previous steps. The method also introduces a concept of AuxCache to store the tokens that are omitted during the previous steps of text generation but required at the current step. Experiments on standard datasets across various tasks demonstrate that LazyLLM can significantly accelerate the generation without fine-tuning, e.g., prefilling stage of the LLama 2 7B model by 2.34x while maintaining accuracy.
- Compact Language Models via Pruning and Knowledge Distillation by Nvidia (https://www.arxiv.org/pdf/2407.14679). Authors propose compression best practices for LLMs that combine depth, width, attention, and MLP pruning with knowledge distillation-based retraining. They arrive at these best practices through a detailed empirical exploration of pruning strategies for each axis, methods to combine axes, distillation strategies, and search techniques for arriving at optimal compressed architectures. They use this guide to compress the Nemotron-4 family of LLMs by a factor of 2-4× and compare their performance to similarly-sized models on a variety of language modeling tasks. Deriving 8B and 4B models from an already pretrained 15B model using this approach requires up to 40x fewer training tokens per model compared to training from scratch; this results in compute cost savings of 1.8x for training the full model family (15B, 8B, and 4B).
- SQFT: Low-cost Model Adaptation in Low-precision Sparse Foundation Models by Intel Labs (https://github.com/IntelLabs/Hardware-Aware-Automated-Machine-Learning). This paper proposes an end-to-end solution for low-precision sparse parameter-efficient fine-tuning of large pre-trained models. It includes an innovative strategy that enables the merging of sparse weights with low-rank adapters without losing the sparsity induced in the base model, overcoming the limitations of previous approaches. SQFT also addresses the challenge of having quantized weights and adapters with different numerical precisions, enabling merging in the desired numerical format without sacrificing accuracy. Multiple adaptation scenarios, models, and comprehensive sparsity levels demonstrate the effectiveness of SQFT. Models and open-source code are available.
- ShadowLLM: Predictor-based Contextual Sparsity for Large Language Models by Cornell University and Google (https://arxiv.org/abs/2406.16635). Contemporary research on contextual sparsity primarily uses magnitude-based metrics to measure the importance of attention heads and neurons in LLMs. This paper aims to assess various importance metrics from the literature, including those based on(1) activation norm, (2) first-order gradient, (3) combination of norm and gradient, (4) second-order gradient, and (5) sensitivity-based metrics. The authors conclude that the PlainAct criterion – the L1-norm of the product of magnitude and gradient – emerges as the better metric by offering a robust sparsity-task tradeoff and learnability in importance rank. The authors also propose using just a single predictor, with the attention scores of the first transformer block as input, to forecast sparsity patterns for the entire LLM, as opposed to DejaVu, which requires predictors at regular intervals of transformer blocks. This innovation simplifies predictor training and implementation while also reducing inference overhead, achieving up to 20% faster generation than DejaVu across sizes of OPT family. Code is here.
- STUN: Structured-Then-Unstructured Pruning for Scalable MoE Pruning by SNU and Snowflake AI Research (https://arxiv.org/pdf/2409.06211). The work discovers a novel way to prune experts of MoE where the method reduces the complexity of expert selection from combinatorial O(kn/√n) down to O(1) using several greedy assumptions. The authors exploit the structure of router weight, applying clustering based on a so-called behavioral similarity metric to identify (dis)similar experts and utilize the centroid as pruned representation to compute a first-order Taylor approximation of the relative distortion. The entire expert pruning can be effectively run without any calibration data and unnecessarily on GPU, especially for the MoE with large numbers of experts. The work also found that expert pruning followed by unstructured pruning provides a better Pareto front. A key result on Snowflake Arctic, a 480B-parameter MoE with 128 experts, shows that STUN achieves 40% sparsity with minimal performance loss in just two hours using a single H100 GPU where unstructured pruning methods alone fall short.
Other
- Accuracy is Not All You Need by Microsoft Research, India (https://arxiv.org/pdf/2407.09141). The authors study the accuracy difference between compressed and source models. They claim that when the accuracy metrics are similar, they observe the phenomenon of flips, wherein answers change from correct to incorrect and vice versa in proportion. The authors conduct a detailed study of metrics across multiple compression techniques, models, and datasets, demonstrating that the behavior of compressed models as visible to end users is often significantly different from the baseline model, even when accuracy is similar. They further evaluate compressed models qualitatively and quantitatively using MT-Bench, showing that compressed models are significantly worse than baseline models in this free-form generative task. They argue that compression techniques should also be evaluated using distance metrics. Finally, the authors propose two metrics, KL-Divergence and % flips, and show that they are well correlated.
- Scaling LLM Test-Time Compute Optimally can be More Effective than Scaling Model Parameters by UC Berkeley and Google DeepMind (https://arxiv.org/pdf/2408.03314).The paper studies the scaling of inference-time computation in LLMs, focusing on answering the question: If an LLM is allowed to use a fixed but non-trivial amount of inference-time compute, how much can it improve its performance on a challenging prompt? Answering this question has implications not only on the achievable performance of LLMs, but also on the future of LLM pretraining and how one should trade inference-time and pre-training compute. Authors analyze two primary mechanisms to scale test-time computation: (1) searching against dense, process-based verifier reward models; and (2) updating the model’s distribution over a response adaptively, given the prompt at test time. They find that in both cases, the effectiveness of different approaches to scaling test-time compute critically varies depending on the difficulty of the prompt. This observation motivates applying a “compute-optimal” scaling strategy, which acts to most effectively allocate test-time compute adaptively per prompt. Using this compute-optimal strategy, authors can improve the efficiency of test-time compute scaling by more than 4x compared to a best-of-N baseline. Additionally, in a FLOPs-matched evaluation, they find that on problems where a smaller base model attains somewhat non-trivial success rates, test-time compute can be used to outperform a 14x larger model.
- Transformers are SSMs: Generalized Models and Efficient Algorithms Through Structured State Space Dual it by Tri Dao and Albert Gu (https://arxiv.org/abs/2405.21060). This paper discusses improvements to Mamba, the selective structure state space model (SSM) proposed as an alternative to Transformer-based models. The authors provide a framework called State Space Duality (SSD) that connects SSMs and variants of the attention mechanism. The Mamba-2 architecture is proposed, which obtains 2-8x speedup compared to the previous version of Mamba, and it is designed to be friendly to tensor and sequence parallelism. Experiments show that Mamba-2 outperforms Mamba and Transformer-based models in different model sizes. The authors also discuss hybrid models that can benefit from the combination of SSD with components from Transformer blocks.
Software
- A thorough analysis of performance and bottlenecks when using 4-bit KV cache on Nvidia with PyTorch: https://pytorch.org/blog/int4-decoding. Authors show step-by-step improvement when computing the Self-Attention operation of the Transformer block and compare results with CUDA and Flash Decoding baselines in 4-bit per-row and per-channel quantization settings of KV-cache.
- Llama.cpp introduced SIMD-friendly ternary weights packing/unpacking: https://compilade.net/blog/ternary-packing
- HuggingFace posted an exploratory work and recipes for ternary weights (1.58 bits) LLM weight compression: https://huggingface.co/blog/1_58_llm_extreme_quantization.
Optimizing Whisper and Distil-Whisper for Speech Recognition with OpenVINO and NNCF
Authors: Nikita Savelyev, Alexander Kozlov, Ekaterina Aidova, Maxim Proshin
Introduction
Whisper is a general-purpose speech recognition model from OpenAI. The model can transcribe speech across dozens of languages and even handle poor audio quality or excessive background noise. You can find more information about this model in the research paper, OpenAI blog, model card and GitHub repository.
Recently, a distilled variant of the model called Distil-Whisper has been proposed in the paper Robust Knowledge Distillation via Large-Scale Pseudo Labelling. Compared to Whisper, Distil-Whisper runs several times faster with 50% fewer parameters, while performing to within 1% word error rate (WER) on out-of-distribution evaluation data.
Whisper is a Transformer-based encoder-decoder model, also referred to as a sequence-to-sequence model. It maps a sequence of audio spectrogram features to a sequence of text tokens. First, the raw audio inputs are converted to a log-Mel spectrogram by action of the feature extractor. Then, the Transformer encoder encodes the spectrogram to form a sequence of encoder hidden states. Finally, the decoder autoregressively predicts text tokens, conditional on both the previous tokens and the encoder's hidden states.
You can see the model architecture in the diagram below:
In this article, we would like to demonstrate how to improve Whisper and Distil-Whisper inference speed with OpenVINO for Intel hardware. Additionally, we show how to make models even faster by applying 8-bit Post-training Quantization with Neural Network Compression Framework (NNCF). In the end we present evaluation results from accuracy and performance standpoints on a large-scale dataset.
All code snippets presented in this article are from the Automatic speech recognition using Distil-Whisper and OpenVINO Jupyter notebook, so you can follow along.
Converting Model to OpenVINO format
We are going to load models from Hugging Face Hub with the help of Optimum Intel library which makes it easier to load and run OpenVINO-optimized models. For more details, pleaes refer to the Hugging Face Optimum documentation.
For example, the following code loads the Distil-Whisper large-v2 model ready for inference with OpenVINO.
Models from the Distil-Whisper family are available at Distil-Whisper Models collection and Whisper models are available at OpenAI Hugging Face page.
To transcribe an input audio with the loaded model, we first compile the model to the device of choice and then call generate() method on input features prepared by corresponding processor.
The output is the following. As you can see the transcription equals the reference text.
Running Post-Training Quantization with NNCF
NNCF enables post-training quantization by adding quantization layers into the model graph and then using a subset of the training dataset to initialize parameters of these additional quantization layers. During quantization, some layers (e.g., MatMuls, Convolutions) are transformed to be executed in INT8 instead of FP16/FP32. If a quantized operation is parameterized then its corresponding weight variable is also converted to INT8.
In general, the optimization process contains the following steps:
- Create a calibration dataset for quantization.
- Run nncf.quantize() to obtain quantized encoder and decoder models.
- Serialize the INT8 models using openvino.save_model() function.
Whisper model consists of an encoder and decoder submodels. Furthermore, for the decoder model its forward() signature is different for the first call compared to all subsequent calls. During the first call, key-value cache is empty and is not needed for decoder inference. Starting from the second call, key-value cache is fed to the decoder. Because of this, these two cases are represented by two separate OpenVINO models: openvino_decoder_model.xml and openvino_decoder_with_past_model.xml. Since the first decoder model is inferred only once it does not make much sense to quantize it. So, we apply quantization to the encoder and the decoder with past models.
The first step towards quantization is collecting calibration data. For that, we need to collect some number of model inputs for both models. To do that, we patch OpenVINO model request objects with an InferRequestWrapper class instance that will intercept model inputs during inference and store them in a list. We infer the model on about 50 samples from validation split of librispeech_asr dataset.
With the collected calibration data for encoder and decoder models we can proceed to quantization itself. Let's examine the quantization call for the encoder model. For the decoder model, it is similar.
After both models are quantized and saved, the quantized Whisper model can be loaded and run the same way as shown previously. Comparing the transcriptions produced by original and quantized models results in the following.
As you can see for the quantized distil-whisper-large-v2 transcription is the same.
Evaluating on Common Voice Dataset
We evaluate Whisper and Distil-Whisper large-v2 model variants on a Common Voice 13.0 speech-to-text dataset. We use en/test split containing 16372 audio samples amounting to about 27 hours of recordings.
The evaluation is done across three model types: original PyTorch model, original OpenVINO model and quantized OpenVINO model. Additionally, we run tests on three Intel CPUs: Cascade Lake Intel(R) Core(TM) i9-10980XE, Ice Lake Intel(R) Xeon(R) Gold 6338 and Sapphire Rapids Intel(R) Xeon(R) Gold 6430L.
For all combinations above we measure transcription time and accuracy. When measuring time for a model we sum up generate() call durations for all audio samples. Transcription accuracy is represented as Accuracy = (100 - WER), WER stands for Word Error Rate. We compute accuracy for each audio sample and then take the average value across the dataset. The results are given in the table below.
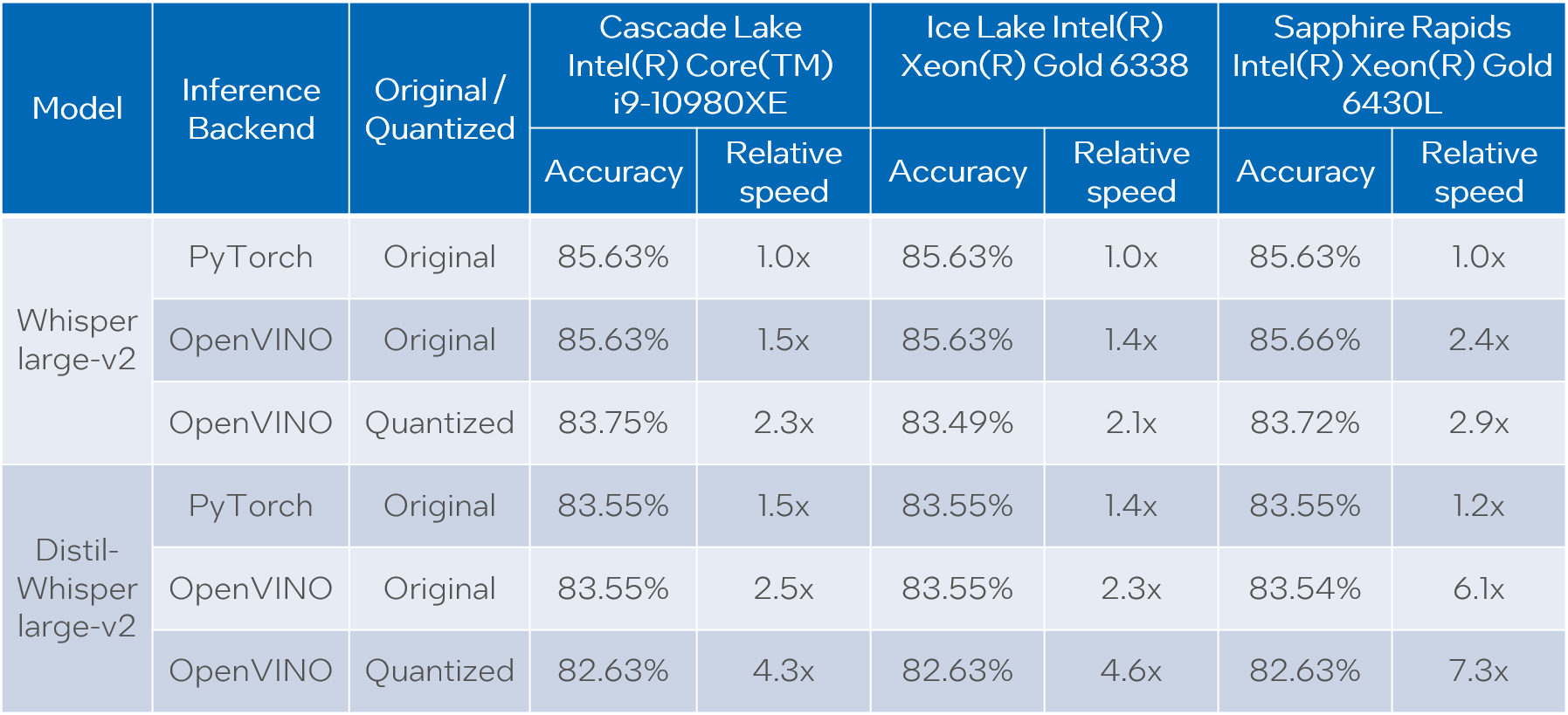
Please note that we report transcription time in relative terms such that the values for each CPU are normalized over its corresponding column. The duration of audio data in the dataset is 27.06 hours and the absolute transcription time values for Whisper large-v2 PyTorch on each CPU are:
- 20.35 hours for Core i9-10980XE
- 14.09 hours for Xeon Gold 6338
- 15.03 hours for Xeon Gold 6430L
Based on the results we can conclude that:
- OpenVINO models execute 1.4x - 5.1x faster than PyTorch models with pretty much the same accuracy across all cases.
- When compared to original PyTorch models, quantized OpenVINO models provide 2.1x - 6.1x performance boost with 1-2% accuracy drop.
NOTE: in terms of this article we focus on presenting performance values. Accuracy of quantized models can be improved with a more careful selection of calibration data.
Notices and Disclaimers:
Performance varies by use, configuration, and other factors. Learn more at www.intel.com/PerformanceIndex. Performance results are based on testing as of dates shown in configurations and may not reflect all publicly available updates. No product or component can be absolutely secure. Intel technologies may require enabled hardware, software or service activation.
The products described may contain design defects or errors known as errata which may cause the product to deviate from published specifications. Current characterized errata are available on request.
Test Configuration: Intel® Core™ i9-10980XE CPU Processor at 3.00GHz with DDR4 128 GB at 3000MHz, OS: Ubuntu 20.04.3 LTS; Intel® Xeon® Gold 6338 CPU Processor at 2.00GHz with DDR4 256 GB at 3200MHz, OS: Ubuntu 20.04.3 LTS; Intel® Xeon® Gold 6430L CPU Processor at 1.90GHz with DDR5 1024 GB at 4800MHz, OS: Ubuntu 20.04.6 LTS. Testing was performed using distil-whisper-asr notebook for model export and whisper evaluation notebook for model evaluation.
The test was conducted by Intel in December 2023.
Conclusion
We demonstrated how to load and run Whisper and Distil-Whisper models for audio transcription task with OpenVINO and Optimum Intel, and how to perform INT8 post-training quantization of these models with NNCF. Further we evaluated these models on a large scale speech-to-text dataset across multiple CPU devices. The evaluation results show a significant performance boost of OpenVINO vs PyTorch models without loss of transcription quality, and even a larger boost with a tolerable accuracy drop when we apply INT8 quantization.
Q4'23: Technology Update – Low Precision and Model Optimization
Authors
Alexander Kozlov, Nikita Savelyev, Nikolay Lyalyushkin, Vui Seng Chua, Pablo Munoz, Alexander Suslov, Andrey Anufriev, Liubov Talamanova, Yury Gorbachev, Nilesh Jain, Maxim Proshin
Summary
This quarter we observe that most of the work is still dedicated to the Large Language Model optimization. Researchers try to break through W4A8 quantization setup for LLMs achieving the accuracy results that allows considering such optimized models for deployment scenario. Some teams work on lower-precision settings such as 2-bit weight quantization or even binary weight compression. Interestingly, some teams propose to stick to a higher bit-width (FP6) and data-free optimization approach to avoid overfitting to calibration data. We also see an increasing interest in applying various types of weight sparsity in LLMs. And, of course, we should note the tremendous improvement in the inference time of Diffusion models caused by the decrease in the overall number of iterations in the diffusion process. This allows running variations of Stable Diffusion on mobile devices in the below 1 second.
Papers with notable results
Quantization
- AWEQ: Post-Training Quantization with Activation-Weight Equalization for Large Language Models by Jilin University (https://arxiv.org/pdf/2311.01305.pdf). Authors apply a known recipe for DL models quantization to LLM models. It contains weight equalization and bias correction methods stacked together. The difference is in how they estimate parameters and where to apply both methods. The method shows good results for W8A8 and W4A8 settings and outperforms GPTQ method on LLAMA and OPT models.
- AFPQ: Asymmetric Floating Point Quantization for LLMs by China Universities and Microsoft Research Asia (https://arxiv.org/pdf/2311.01792.pdf).Authors propose accurate asymmetric schema for the floating-point quantization. Instead of using typical asymmetric schema with scale and zero point, they use just2 scales: one is for positive values and another - for negative ones. It gives better accuracy NF4/NF3 quantization on different LLAMA models with no memory overhead. Code is available: https://github.com/zhangsichengsjtu/AFPQ.

- Enhancing Computation Efficiency in Large Language Models through Weight and Activation Quantization by Hanyang University, SAPEON Korea Inc., Seoul National University (https://arxiv.org/pdf/2311.05161.pdf). Authors present two techniques: activation-quantization-aware scaling (a trade-off between SQ and AWQ) and sequence-length-aware calibration (adaptation of OPTQ to various sequence lengths) to enhance PTQ by considering the combined effects on weights and activations and aligning calibration sequence lengths to target tasks. They also introduce dINT, a hybrid data format combining integer and denormal representations, to address the underflow issue in W4A8 quantization, where small values are rounded to zero. The combined approach allows for achieving superior results compared to baselines. However, dINT has a limitation of efficient implementation on a general-purpose HW, such as CPU and GPU.
- POST-TRAINING QUANTIZATIONWITH LOW-PRECISION MINIFLOATS AND INTEGERS ON FPGAS by AMD Research, National University of Singapore, and Tampere University (https://arxiv.org/pdf/2311.12359.pdf). Authors compare integer and mini float quantization techniques, encompassing a combination of state-of-the-art PTQ methods, such as weight equalization, bias correction, SmoothQuant, learned rounding, and GPTQ. They explore the accuracy-hardware tradeoffs, providing analysis for three models -ResNet-18, MobileNetV2, and ViT-B32 - based on a custom FPGA implementation. Experiments indicate that mini float quantization typically outperforms integer quantization for bit-widths of four or more, both for weights and activations. However, when compared against FPGA hardware cost model, integer quantization often retains its Pareto optimality due to its smaller hardware footprint at a given precision.

- I&S-ViT: An Inclusive& Stable Method for Pushing the Limit of Post-Training ViTs Quantization by Xiamen University, Tencent, and Peng Cheng Laboratory (https://arxiv.org/pdf/2311.10126.pdf). The paper introduces a method that regulates the PTQ of ViTs in an inclusive and stable fashion. It first identifies two issues in the PTQ of ViTs: (1)Quantization inefficiency in the prevalent log2 quantizer for post-Softmax activations; (2) Rugged and magnified loss landscape in coarse-grained quantization granularity for post-LayerNorm activations. Then, the method addresses these issues by introducing: (1) A novel shift-uniform-log2 quantizer(SULQ) that incorporates a shift mechanism followed by uniform quantization to achieve both an inclusive domain representation and accurate distribution approximation; (2) A three-stage smooth optimization strategy that amalgamates the strengths of channel-wise and layer-wise quantization to enable stable learning. The method achieves comparable results in the W4A4 and W3A3 quantization settings.
- Quantizable Transformers: Removing Outliers by Helping Attention Heads Do Nothing by Qualcomm AI Research (https://arxiv.org/pdf/2306.12929.pdf). This work aims to remove outliers by construction (pretraining) so that transformer can be quantized easily without the need of finer quantization granularity (e.g. per channel, per group). The authors root-caused that outliers in trained transformers are essentially the artifact of attention head attenuating uninformative tokens and outliers emerged in the formulation/backpropagation of softmax, residual connections and layer normalization to sustain the effect of these tokens. Two independent solutions are proposed - (1) Clipped softmax that allows exact zeros and ones in softmax to avoid growing outliers during training. (2) Gated attention which is a tiny neural network (linear+sigmoid) added to the vanilla attention to decouple the needs of large attention output for disregarding the uninformative tokens. Pretraining with the proposed formulation on BERT, OPT and ViT has been shown to converge similarly if not better than baseline recipe. Most notably, the ease of per-tensor int8 static quantization to both weight and activation in post-training fashion has been empirically verified. Code is coming soon at https://github.com/qualcomm-ai-research/outlier-free-transformers
- A Speed Odyssey for Deployable Quantization of LLMs by Meituan (https://arxiv.org/pdf/2311.09550.pdf).Authors propose a solution for deployable W4A8 quantization that comprises a tailored quantization configuration and a novel Fast GEMM kernel for 4-bitinteger matrix multiplication that reduces the cost, and it achieves 2.23× and1.45× speed boosting over the TensorRT-LLM FP16 and INT8 implementation respectively. The W4A8 recipe is proven mostly on par with the state-of-the-art W8A8 quantization method SmoothQuant on a variety of common language benchmarks for the state-of-the-art LLMs.
- TFMQ-DM: Temporal Feature Maintenance Quantization for Diffusion Models by SenseTime and universities of US, China and Australia (https://arxiv.org/pdf/2311.16503.pdf). Authors investigate the problems in quantization of diffusion models and claim that these models heavily depend on the time-step t to achieve satisfactory multi-round denoising when t is encoded to a temporal feature by a few modules totally irrespective of the sampling data. They propose a Temporal Feature Maintenance Quantization framework building upon a Temporal Information Block which is just related to the time-step t and unrelated to the sampling data. Powered by this block design, authors propose temporal information aware reconstruction and finite set calibration to align the full-precision temporal features in a limited time. The method achieves accurate results even in W4A8quantization setting.
- QUIK: TOWARDS END-TO-END4-BIT INFERENCE ON GENERATIVE LARGE LANGUAGE MODELS by ETH Zurich, Institute of Science and Technology Austria, Xidian University, KAUST, Neural Magic (https://arxiv.org/pdf/2310.09259v2.pdf). The paper addresses the problem where both weights and activations should be quantized. Authors show that the majority of inference computations for large generative models such as LLaMA, OPT, and Falcon can be performed with both weights and activations being cast to 4 bits, in a way that leads to practical speedups, while at the same time maintaining good accuracy. They achieve this via a hybrid quantization strategy called QUIK, which compresses most of the weights and activations to 4-bit, while keeping some outlier weights and activations in higher precision which leads to practical end-to-end throughput improvements of up to 3.4x relative to FP16 execution. Code is available at: https://github.com/IST-DASLab/QUIK.
- Post-training Quantization with Progressive Calibration and Activation Relaxing for Text-to-Image Diffusion Models by Tsinghua University (https://arxiv.org/pdf/2311.06322.pdf). Authors propose a post-training quantization method for text-to-image diffusion models, which consists of a progressive calibration strategy that considers the accumulated quantization error across timesteps, and an activation relaxing strategy that improves the performance with a small cost. They also propose a new QDiffBench benchmark, which utilizes data in the same domain for a more accurate evaluation of the generation accuracy.
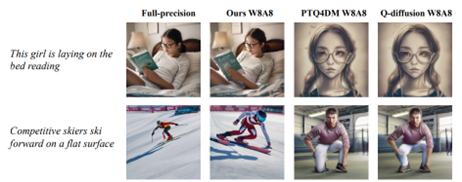
- Enabling Fast 2-bit LLM on GPUs: Memory Alignment, Sparse Outlier, and Asynchronous Dequantization by Shanghai Jiao Tong University and Tsinghua University (https://arxiv.org/pdf/2311.16442.pdf).The paper proposes range-aware quantization with memory alignment. It points out that the range of weights by groups varies. Thus, only 25% of the weights are quantized using 4-bit with memory alignment. Such a method reduces the accuracy loss for 2-bit Llama2-7b quantization from 8.7% to 2.9%. Authors show as well that only a small fraction of outliers exist in weights quantized using2-bit. These quantize these sparse outliers with < 3% increased average weight bit and improve the accuracy by >0.5%. They also accelerate GPU kernels by introducing asynchronous dequantization achieving 3.92× improvement on a kernel level.
- ZeroQuant(4+2): Redefining LLMs Quantization with a New FP6-Centric Strategy for Diverse Generative Tasks by DeepSpeed (https://arxiv.org/pdf/2312.08583.pdf).Authors show that popular data-aware LLM compression methods such as GPTQ can overfit to calibrated datasets especially on moderate-size LLMs (<=1B). They also illustrate that FP6, employing a basic round-to-nearest (RTN) algorithm and a per-channel quantization approach, consistently achieves accuracy on par with full-precision models. They propose an unpacking (mapping) scheme for FP8 so that it can be efficiently used with FP16 inference.
Pruning/Sparsity
- Sparse Fine-tuning for Inference Acceleration of Large Language Models by IST Austria, Skoltech & Yandex, and Neural Magic (https://arxiv.org/pdf/2310.06927.pdf). The paper analyses the challenges of LLMs pruning, namely loss spikes leading to divergence, poor recovery from fine-tuning, and overfitting. To overcome these issues, authors incorporate standard cross-entropy, output knowledge distillation, and a type of per-token ℓ2 knowledge distillation on top of SparseGPT method. They show that the resulting sparse models can be executed with inference speedups on CPU and GPU, especially when stacking with INT8quantization. The code is available: https://github.com/IST-DASLab/SparseFinetuning.
- ReLU Strikes Back: Exploiting Activation Sparsity in LLMs by Apple. (https://arxiv.org/pdf/2310.04564.pdf). This work advocates to reinstate ReLU as main activation function in LLMs due to its intriguing property – high post-ReLU activation sparsity can be translated to computational efficiency with sparse runtime. To overcome training from scratch and for LLMs employing GeLU/SiLU, the paper proposes “Relufication”, a two-stage uptraining by first replacing non-ReLU activations in pre-trained LLMs with ReLU, and then appending ReLU to normalization layers in second stage for more sparsity. With increase of activation sparsity, the authors also observe high overlapping activated neurons during decoding (termed aggregated sparsity) and suggest weight reuse to alleviate memory transfer. The authors show application of aggregated sparsity in speculative decoding and demonstrate 27% speedup of OPT-6.7B at a minor degradation of perplexity.
- Deja Vu: Contextual Sparsity for Efficient LLMs at Inference Time by Rice University, Zhe Jiang University, Stanford University, University of California, ETH Zurich, Adobe Research, MetaAI, Carnegie Mellon University (https://proceedings.mlr.press/v202/liu23am/liu23am.pdf). Authors propose a contextual dynamic sparsity method for LLMs. Contrary to a usual sparsity approach where a model is pruned once and then inferenced on every input sample in the same pruned state, here authors compute the set of pruned operations on the run resulting in a more flexible pruning scheme. Foreach transformer layer this is achieved by predicting set of MHA heads and MLP matrix columns to exclude based on previous layers activations. Prediction is performed by small separately trained perceptron networks. To remove the performance bottleneck produced by the need to inference of perceptron networks authors propose to make sparsity predictions for (i+1)-th transformer layer based on activations from (i-1)-th layer, resulting in parallel computation of i-th layer and sparsity sets for (i+1)-th layer. This is viable due to shown similarity between activations of neighboring layers in LLMs. For OPT-175Bmodel the approach achieves over 6x performance improvement compared to Hugging Face implementation and over 2x improvement compared to state-of-the-art FasterTransformer model.
- SparseByteNN: A Novel Mobile Inference Acceleration Framework Based on Fine-Grained Group Sparsity by ByteDance (https://arxiv.org/pdf/2310.19509.pdf). The paper introduces SparseByteNN, consisting of three components: a)compression algorithm component, which provides out-of-the-box pruning capabilities for pre-trained models b) model conversion tool, which converts the model IR of the training framework into Model IR of sparse engine c) sparse inference engine, which provides efficient inference implementation compatible with CPUs for fine-grained kernel group sparsity. Experimental results on Qualcomm 855 show that for 30% sparse MobileNet-v1, SparseByteNN achieves 1.27×speedup over the dense version. The code will be available at: https://github.com/lswzjuer/SparseByteNN.
Neural Architecture Search
- LoNAS: Elastic Low-Rank Adapters for Efficient Large Language Models by Anonymous (https://openreview.net/pdf?id=pzB-1OCS6gd). Researchers demonstrate a novel integration of low-rank (LoRA)adapters with Neural Architecture Search. LoNAS efficiently fine-tunes and compress large language models (LLMs). A weight-sharing super-network is generated using the frozen weights of the input model, and the attached elastic low-rank adapters. The reduction in trainable parameters results in less the memory requirements to train the super-network, enabling the manipulation of LLMs in resource-constrained devices, without sacrificing the performance of the resulting compressed models. LoNAS’ high-performing compressed models result in faster inference times, cost savings during the model’s lifetime, and an increase in the range of devices in which large language models can be deployed. Experiments’ results on six reasoning datasets demonstrate the benefits of LoNAS.
- Bridging the Gap between Foundation Models and Heterogenous Federated Learning by Iowa State U. and Intel Labs (https://arxiv.org/abs/2310.00247). This paper explores the application of Neural Architecture Search (NAS) in combination with Federated Learning (FL). The proposed framework, Resource-aware Federated Foundation Models (RaFFM) introduces model compression and salient parameter prioritization in the context of Federated Learning, allowing for the collaborative training of large foundation models using heterogeneous devices. Compared to traditional FL methods, RaFFM yields better resource utilization, without sacrificing in model performance.
- Rankitect: Ranking Architecture Search Battling World-class Engineers at Meta Scale by Meta Platforms (https://arxiv.org/pdf/2311.08430.pdf). Researchers at Meta demonstrate the real-world applications of Neural Architecture Search (NAS). They apply NAS to production models, e.g., Click Through Rate (CTR) model, on a system that serves billions of users. The baseline models explored in this work have already been optimized by world-class engineers. The proposed NAS framework, Rankitect, improves over existing models by exploring search spaces with no inductive bias from the baseline models, and discovers new models from scratch that outperform those hand-crafted by human experts. Rankitect also keeps human engineers in-the-loop by allowing the manual design of search spaces, which results in even more efficient models.
- QuadraNet: Improving High-Order Neural Interaction Efficiency with Hardware-Aware Quadratic Neural Networks by George Mason University, University of Maryland, University at Buffalo, Peking University (https://arxiv.org/pdf/2311.17956.pdf). This paper presents QuadraNet, a new neural network design methodology based on efficient quadratic neurons that captures high-order neural interactions similar to Transformer-based models. The design of this alternative to Transformer-based models is hardware-aware through the application of Neural Architecture Search(NAS). Experiments with QuadraNets show improvements of 1.5x in throughput without any reduction in accuracy compared to their Transformer-based counterparts.
Other
- Divergent Token Metrics: Measuring degradation to prune away LLM components – and optimize quantization by Aleph Alpha, Hessian.AI and German Universities. (https://arxiv.org/pdf/2311.01544.pdf). The work highlights that the commonly used perplexity (PPL) metric in compression research does not reflect the degradation of compressed model and cannot distinguish subtleties (see figure below). The authors propose a family of divergent token metrics (DTM), namely First Token Divergence, i.e., when the first diverging token happens w.r.t baseline generated text, as well as Share of Divergent Tokens denoting the total number of divergent tokens. In a series of experiments pertaining to layer-wise quantization or pruning, DTM-based ranking consistently outperforms PPL-based ranking methods.

- SIMPLIFYING TRANSFORMERBLOCKS by ETH Zurich (https://arxiv.org/pdf/2311.01906.pdf). The paper introduces a set of Transformer block pruning techniques that makes them more lightweight from the number of parameters and computations standpoint. This set includes: removing skip connection both in the Attention sub-block and Feed-Forward sub-block, removing value and projection parameters, removing normalization layers, and model depth scaling. Authors also show how to recover the accuracy after model perturbation using fine-tuning. The proposed method produces the decoder and decoder Transformer models that perform on part with their baselines: 15% faster training throughput, and using 15% fewer parameters.
- MobileDiffusion: Subsecond Text-to-Image Generation on Mobile Devices by Google (https://arxiv.org/pdf/2311.16567.pdf).The paper presents a comprehensive guide for crafting highly efficient text-to-image diffusion models. Authors applied the following tricks to highly optimize UNet model in the Diffusion pipeline: more transformers in the middle of Unet (at lower resolution), retaining cross-attention layers while discarding only the self-attention layers at high resolutions, sharing key-value projections, replacing gelu with swish, fine-tune softmax into relu, trim feed-forward layers, use separable convolution, prune redundant residual blocks, reduce sampling iterations, knowledge distillation. The resulting model is able to generate 512×512 images in sub-second on mobile devices: 0.2 second on iPhone 15 Pro.

- Online Speculative Decoding by UC Berkeley, UCSD, Sisu Data, SJTU (https://arxiv.org/pdf/2310.07177.pdf). Practical speedup of speculative decoding is often impeded by the capability gap between draft and target model which can be 10-20X gap in parameters, leading to high rejection of draft predictions and fallback to more forward passes of target model. This work proposes online fine-tuning of draft model by distillation with the readily available rejected predictions. The proposed solution incorporates a replay buffer tracking logits of draft and target model, and distillation backpropagation is executed at a regular interval. Experimental results demonstrate not only significant improvement in acceptance rate, translating up to a theoretical 3X of latency reduction, but also adaptability against distribution shift in input queries.
- Token Fusion: Bridging the Gap between Token Pruning and Token Merging by Michigan State University Samsung Research America (https://arxiv.org/pdf/2312.01026.pdf).The paper introduces a method (ToFu) that combines token pruning and token merging. ToFu dynamically adapts to each layer’s properties, ensuring optimal performance based on the model’s functional linearity with respect to the interpolation in its input. Authors exploit MLERP merging technique, an enhancement over traditional average merging, inspired by the SLERP method. This approach merges tokens while preserving their norm distribution. Evaluation shows that ToFu outperforms ToMe in terms of accuracy while showing the similar performance gain at inference.
Deep Learning Software
- Medusa: Simple Framework for Accelerating LLM Generation with Multiple Decoding Heads by Together.AI. Contemporary speculative decoding solutions (Leviathan et al., Chen et al.) require multiple models (target and draft models) which often involves intricate optimization& selection of draft models to attain practical acceleration. For simplicity, Together.AI unveils a user-friendly framework, Medusa, built a top of a research work in 2018, "Block wise Parallel Decoding", with multiple enhancements. Medusa simplifies the creation of draft models without separate models by extending base model with multiple decoding heads. By keeping the base model frozen, the Medusa heads are trained in a parameter-efficient way and all on a single GPU. Medusa also features a tree-based attention mechanism for parallel evaluation of the proposed candidates, and a truncated sampling for efficient creative generation. Results and framework can be found at https://github.com/FasterDecoding/Medusa.
- HyperAttention: Long-context Attention in Near-Linear Time by Yale University and Google https://github.com/insuhan/hyper-attn. Authors propose algorithm that consists of (1) finding heavy entries inattention matrix and (2) column subsampling. For (1), authors use the sorted locality sensitive hashing (sortLSH) based on the Hamming distance. Applying sortLSH makes heavy entries in the attention matrix (sorting rows/columns) located in near diagonal hence authors do block-diagonal approximation which can be done fast. The method supports casual masking. Code is available here: https://github.com/insuhan/hyper-attn.
- Flash-Decoding for long-context inference by Stanford University. Flash Attention v1 & v2 are designed and optimized primarily for training case and exhibit low utilization of compute units when applied for LLM generation, especially for long context. As identified cause is rooted in low- batch size (query tokens) in relative to context length, Flash Decoding extends flash attention by adding 2nd-level tiling over the keys/values to improve compute utilization while retaining memory efficiency of flash attention. On A100, the micro-benchmark for multi-head attention with flash decoding kernel achieves almost constant run-time as the sequence length scales to up to 64k, translating up to 8X speedup of CodeLLaMa-34b over vanilla flash attention at very long sequences. Implementation is available at official flash attention repo & xformers.
- LLM in a flash: Efficient Large Language Model Inference with Limited Memory by Apple (https://arxiv.org/pdf/2312.11514.pdf).The paper tackles the challenge of efficiently running LLMs that exceed the available DRAM capacity by storing the model parameters on flash memory but bringing them on demand to DRAM. The method involves constructing an inference cost model that harmonizes with the flash memory behavior, guiding to optimize in two critical areas: reducing the volume of data transferred from flash and reading data in larger, more contiguous chunks. Within this flash memory-informed framework, authors introduce two principal techniques. First, “windowing” strategically reduces data transfer by reusing previously activated neurons, and second, “row-column bundling”, tailored to the sequential data access strengths of flash memory, increases the size of data chunks read from flash memory. These methods collectively enable running models up to twice the size of the available DRAM, with a 4-5x and 20-25x increase in inference speed compared to naive loading approaches in CPU and GPU, respectively.
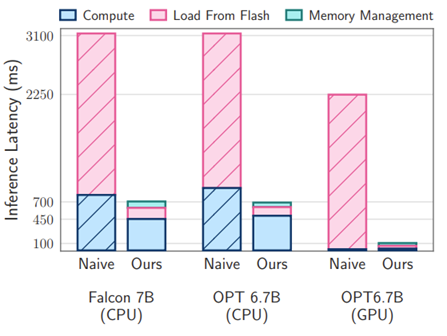
Efficient Inference and Quantization of CGD for Image Retrieval with OpenVINO™ and NNCF
Author:Xiake Sun, Wenyi Zou, Churkin Andrey
Introduction
With the advent of e-commerce and online websites, image retrieval applications have been increasing all along around our daily life. Top e-commerce platform such as Amazon and Alibaba have been heavily utilizing image retrieval to put forward what they think is the most suitable product based on what we have seen just now.
Image retrieval is the process of finding an image from a collection or database from the traits of a query image. The traits are usually visual similarities between the images. The top retrieved images can provide hypotheses about which parts of the scene are likely visible in the query image.
Since images in their original form don’t reflect these traits in their pixel-based data, we need to transform this pixel data into a latent space where the representation of the image will reflect the traits. Naver Corporation proposed Combination of Multiple Global Descriptors (CGD) for Image Retrieval task. The CGD framework exploits multiple global descriptors to get an ensemble effect when it can be trained in an end-to-end manner. Quantitative and qualitative analysis results show that exploiting multiple global descriptors led to higher performance over the single global descriptor.
Neural Network CompressionFramework (NNCF) provides a suite of post-training and training-time algorithms for neural network inference optimization in OpenVINO™ with minimal accuracy drop. NNCF is designed to work with models from PyTorch, TensorFlow, ONNX, and OpenVINO™. In this blog, we use NNCF Post-Training Quantization (PTQ) to quantize CGD model, which can further boost inference while keeping acceptable accuracy without fine-tuning.

Figure1. shows the CGD framework. The framework is described with ResNet-50 backbone where Stage 3 down sampling is removed. From the last feature map, each of n global descriptor branches outputs a k-dimensional embedding vector, which is concatenated into the combined descriptor for ranking loss. Exclusively the first global descriptor is used for auxiliary classification loss where M denotes the number of classes.
CGD framework utilizes the following global descriptors with different focuses:
- Sum pooling of convolutions (SPoC): activates larger regions on the image representation.
- Generalized mean pooling (GeM): generalizes max and average pooling with a pooling parameter.
- Maximum activation of convolutions (MAC): activates more focused regions.
In this blog, we choose CGD ResNet50(SG) model with ResNet50 backbone that combines SPoC and GeM type of global descriptors. Figure 2 shows some retrieval results of CGD Pytorch model based on Standard Online Products (SOP) dataset. The left most query image serves as input to retrieve the 8 most similar image from the database, where the green bounding box means that the predicted class match the query image class, while the red bounding box means a mismatch of image class. Therefore, the retrieved image can be further filtered out with class information.
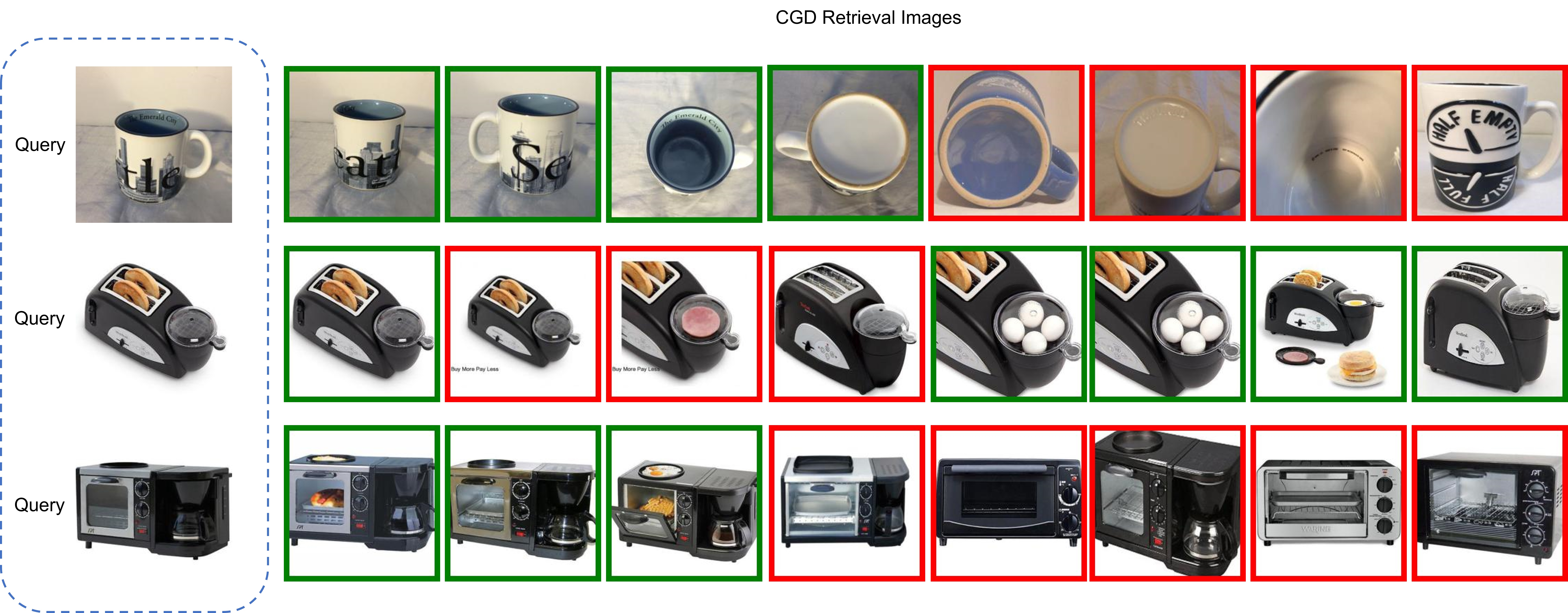
CGD Model Enabling and Quantization with OpenVINO™ and NNCF
To leverage efficient inference with OpenVINO™ runtime on the intel platform, we proposed the following workflow in Figure 3 for CGD model enabling and quantization with OpenVINO™ and NNCF PTQ, which is implemented in a single Python script run_quantize.py.

CGD model uses ResNet50 backbone extracted latent feature to create multiple global descriptors, then the global descriptors will be normalized and concatenated as output.

For INT8 quantization, we found some useful tricks to mitigate accuracy issue caused by accuracy sensitive layers, e.g., YOLOv8 OpenVINO Notebook proposes to keep several accuracy sensitive layers in post-processing subgraph as FP32 precision to better preserve accuracy after NNCF PTQ.
For INT8 quantization of CGD model, the left part of Figure 4 shows the subgraph of CGD for global descriptor combination and normalization. Original torch.nn.functional.normalize is accuracy sensitive, which are converted to OpenVINO™ operators (e.g. Power, Divide). Quantization of these operators from FP32 to INT8 weights can lead to accuracy degradation. Here we marked all accuracy-sensitive layers in the right part of Figure 4.
Furthermore, we can use ignored_scopes in NNCF configuration to skip these layers for INT8 quantization to remain FP32 precision as follows:
CGD OpenVINO™ Demo
Here we can run a CGD demo with CGD_OpenVINO_Demo as follows:
Setup Environment
Prepare dataset based on Standard Online Products (SOP)
Download pre-trained Pytorch CGD ResNet50(SG) model trained on SOP dataset to the results directory.
Verify Pytorch FP32 Model Image Retrieval Results
Run NNCF PTQ for default quantization with ignore scopes
Generated FP32 ONNX model and FP32/INT8 OpenVINO™ model will be saved in the “models” directory. Besides, we also store evaluation results of OpenVINO™ FP32/INT8 model as a Database in the “results” directory respectively. The database can be directly used for image retrieval via input query image.
Verify OpenVINO™ FP32 Model Image Retrieval Results
Verify OpenVINO™ INT8 Model Image Retrieval Results

Table 1 shows CGD OpenVINO™ FP32 and INT8 accuracy verification and performance evaluation results with OpenVINO™ 2023.0 on Intel® Xeon® Platinum 8358 Processor.
From an accuracy perspective, test_recall@1/2/4/8 measures if the top n image retrieval results match with the query image. OpenVINO™ INT8 PTQ quantizes all FP32 layers to INT8, which leads to ~1.2% accuracy degradation compared with OpenVINO™ FP32 Model. OpenVINO™ INT8 PTQ (w/ IgnoreScope) skips quantization of accuracy sensitive layers via ignore scopes, which controls the accuracy difference between OpenVINO™ INT8 model and OpenVINO™FP32 model within 0.16%.
Compared with OpenVINO™ FP32 model, both OpenVINO™ INT8 PTQ and OpenVINO™ INT8 PTQ (w/ Ignore Scopes) can reach ~4x performance boost. Results show that keeping serval layers as FP32 precision has minimal impact on OpenVINO™ INT8 model.

Figure 5 shows the CGD Image Retrieval Results of Pytorch FP32, OpenVINO™ FP32/INT8 models with the same query image. The Pytorch and OpenVINO™ FP32 retrieved images are the same. Although the 7th image of OpenVINO™ INT8 model results is not matched with FP32 model's results, it can be further filtered out with predicted class information.
Conclusion
In this blog, we introduced how to enable and quantize the CGD model with OpenVINO™ runtime and NNCF:
- Proposed INT8 quantization NNCF PTQ with ignore scopes to reach ~4x performance boost while keeping minimal accuracy degradation (<0.16%) compared to FP32 model.
- Provided a demo repository for CGD model enabling, quantization, accuracy verification, and deployment with OpenVINO™ and NNCF.
Reference
Joint Pruning, Quantization and Distillation for Efficient Inference of Transformers
Introduction
Pre-trained transformer models are widely deployed for various NLP tasks such as text classification, question answering, and generation task. The recent trend is that models continue to scale while yielding improved performance. However, growth of transformers also leads to great amount of compute resources and energy needed for deployment. The goal of model compression is to achieve model simplification from the original without significantly diminished accuracy. Pruning, quantization, and knowledge distillation are the three most popular model compression techniques for deep learning models. Pruning is a technique for reducing the size of a model to improve efficiency or performance. By reducing the number of bits needed to represent data, quantization can significantly reduce storage and computational requirements. Knowledge distillation involves training a small model to imitate the behavior of a larger model.
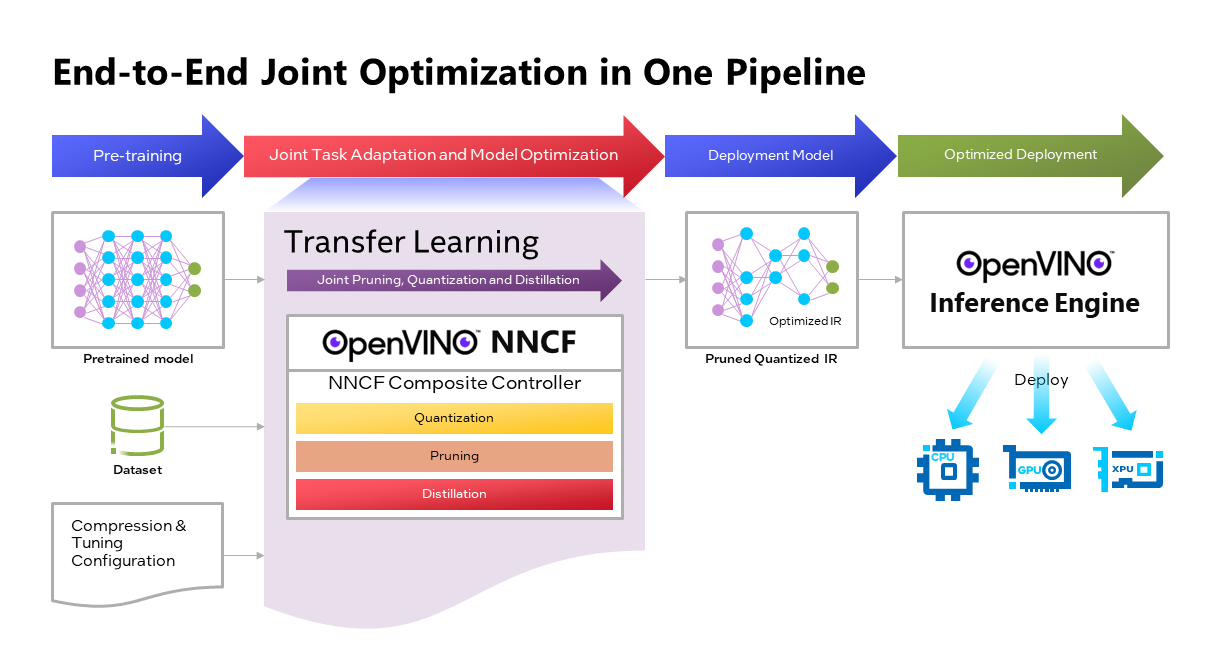
OpenVINOTM Neural Network Compression Framework (NNCF) develops Joint Pruning, Quantization and Distillation (JPQD) as a single joint-optimization pipeline to improve transformer inference performance by pruning, quantization, and distillation in parallel during transfer learning of a pretrained transformer. JPQD alleviates the developer complexity of sequential optimization of different compression techniques, resulting in an optimized model with significant efficiency improvement while preserving good task accuracy. The output of JPQD is a structurally pruned, quantized model in OpenVINOTM IR, which is ready to deploy with OpenVINOTM runtimes optimized on Intel platforms. Optimum intel provides simple API to integrate JPQD into training pipeline for Hugging Face Transformers.
JPQD of BERT-base Model with Optimum Intel
In this blog, we introduce how to apply JPQD to BERT-base model on GLUE benchmark for SST-2 text classification task.
Here is a compression config example with the format that follows NNCF specifications. We specify pruning and quantization in a list of compression algorithms with hyperparameters. The pruning method closely resembles the work of Movement Pruning (Sanh et al., 2020) and Block Pruning For Faster Transformers (Lagunas et al., 2021) for unstructured and structured movement sparsity. Quantization refers to Quantization-aware Training (QAT), see details for QAT in previous blog. At the beginning of training, the model under optimization will be initialized with pruning and quantization operators with this configuration.

Figure 2 shows the sparsity level of BERT-base model over the optimization lifecycle, including two major stages:
- Unstructured sparsification: In the first stage, model weights are gradually sparsified in the grain size specified by "sparse_structure_by_scopes". The BertAttention layers (Multi-Head Attention: MHA) will be sparsified in 32x32 block size, while BertIntermediate, and BertOutput layers (Feed-Forward Network: FFN) will be sparsified in its row or column respectively. The first stage serves as a warmup stage defined by parameter “warmup_start_epoch” and “warmup_end_epoch”. The “importance_regularization_factor” defines regularization factor onweight importance scores. The factor stays zero before warmup stage, and gradually increases during warmup, finally stays at the fixed value after warmup, users might need some heuristics to find a satisfactory trade-off between sparsity and task accuracy.
- Structured masking and fine-tuning: The first warm-up stage will produce the unstructured sparsified model. Currently, unstructured sparsity optimized inference is only supported on 4th Gen Intel® Xeon® Scalable Processors with OpenVINO 2022.3 or a later version, for details, please refer to Accelerate Inference of Sparse Transformer Models with OpenVINO™ and 4th Gen Intel® Xeon®Scalable Processors. But it is possible to discard some sparse structure entirely from the model to save compute and memory footprint. NNCF provides a mechanism to achieve structured masking by “enable_structured_masking”: true, where it automatically resolves the structured masking between dependent layers and rewinds the sparsified parameters that do not participate in acceleration for task modeling. As Figure 2 shows, the sparsity level has dropped after “warmup_end_epoch” due to structured masking and the model will continue to be fine-tuned.
Known limitation: currently structured pruning with movement sparsity only supports BERT, Wav2vec2, and Swin family of models. See here for more information.
For distillation, the teacher model can be loaded with transformer API, e.g., a BERT-large pre-trained model from Hugging Face Hub. OVTrainingArguments extends transformers’ TrainingArguments with distillation hyperparameters, i.e., distillation weight and temperature for ease of use. The snippet below shows how we load a teacher model and create training arguments with OVTrainingArguments. Subsequently, the teacher model, with the instantiated OVConfig and OVTrainingArguments is fed to OVTrainer. The rest of the pipeline is identical to the native transformers' training, while internally the training is applied with pruning, quantization, and distillation.
Besides, NNCF provides JPQD examples of othertasks, e.g., question answering. Please refer to the examples provided here.
End-to-End JPQD of BERT-base Demo
Set up Python environment with necessary dependencies.
Run text classification example with JPQD of BERT on GLUE
All JPQD configurations and results are saved in ./jpqd-bert-base-ft-$TASK_NAME directory. Optimized OpenVINOTM IR is generated for efficient inference on intel platforms.
BERT-base Performance Evaluation and Accuracy Verification on Xeon

Table 1 shows BERT-base model for text classification task performance evaluation and accuracy verification results on 4th Gen Intel® Xeon® Scalable Processors. BERT-base FP32 model serves as the baseline. BERT-base INT8 (QAT) refers to the model optimized with the 8-bit quantization method only. BERT-base INT8 (JPQD) refers to the model optimized by pruning, quantization, and distillation method.
Here we use benchmark app with performance hint “throughput” to evaluate model performance with input sequence length=128.
As results shows, BERT-base INT8 (QAT) can already reach a 2.39x compression rate and 3.17x performance gain without significant accuracy drop (1.3%) on SST-2 compared with baseline. BERT-base INT8 (JPQD) can further increase compression rate to 5.24x to reach 4.19x performance improvement while keeping minimal accuracy drop (<1%) on SST-2 compared with baseline.

With proper fine-tuning, JPQD can even improve model accuracy while increasing performance in the meantime. Table 2 shows BERT-base model for question answering task performance evaluation and accuracy verification results on 4th Gen Intel® Xeon® Scalable Processors. BERT-base INT8 (JPQD) can increase compression rate to 5.15x to reach 4.25x performance improvement while improving Exact Match (1.35%) and F1 score (1.15%) metric on SQuAD compared with FP32 baseline.
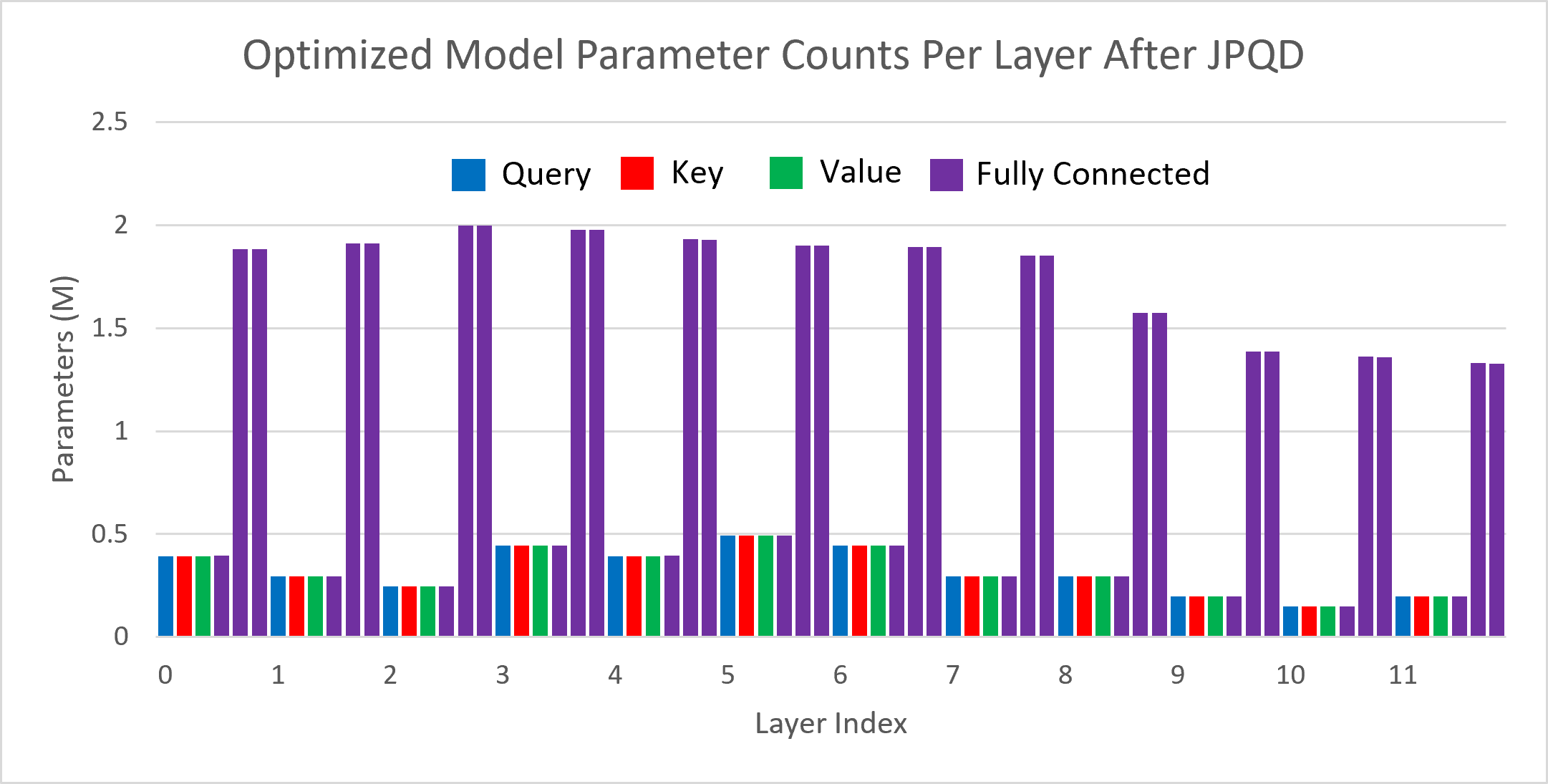
Figure 3 shows the visualization of parameter counts per layer in the BERT-base model optimized by JPQD for the text classification task. You can find that fully connected layers are actually “dense”, while most (Multi-Head Attention) MHA layers will be much sparser compared to the original model.
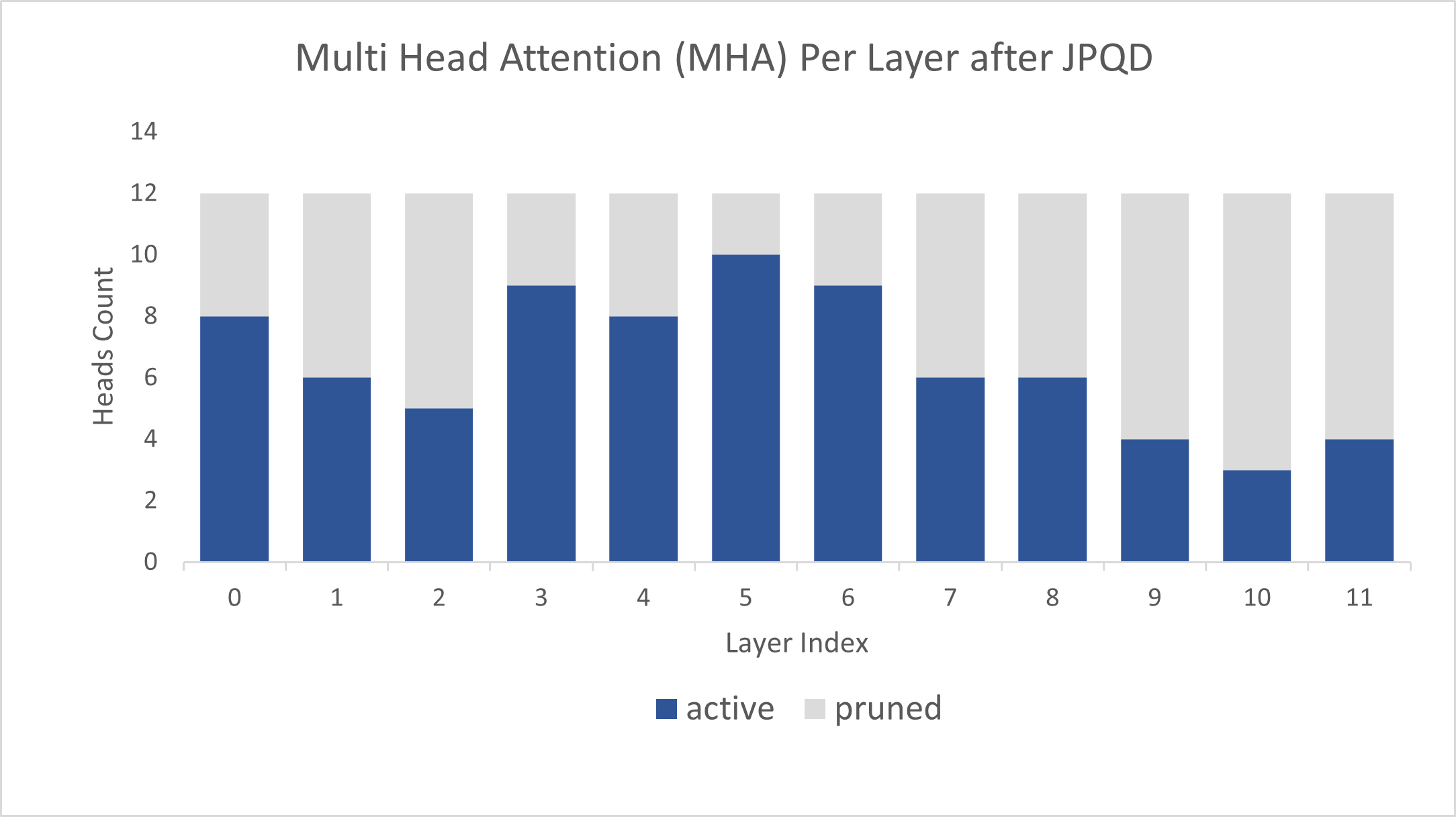
Figure 4 shows MHA head counts per layer in the BERT-base model optimized by JPQD for the text classification task, where active (blue) refer to remaining MHA head counts, while pruned (grey) refers to removed MHA head counts. Instead of pruning uniformly across all MHA heads in transformer layers, we observed that JPQD tends to preserve the weight to the lower layers while heavily pruning the highest layers, similar to experimental results from Movement Pruning (Sanh et al., 2020).
Conclusion
In this blog, we introduce a Joint Pruning, Quantization, and Distillation (JPQD) method to accelerate transformers inference on intel platforms. Here are three key takeaways:
- Optimum Intel provides simple API to integrate JPQD into training pipeline to enable pruning, quantization, and distillation in parallel during transfer learning of a pre-trained transformer. Optimized OpenVINOTM IR will be generated for efficient inference on intel architecture.
- BERT-base INT8 (JPQD) model for text classification task can reach 5.24x compression rate, leading to 4.19x performance improvement on 4th Gen Intel® Xeon® Scalable Processors while keeping minimal accuracy drop (<1%) on SST-2 compared with BERT-base FP32 models.
- BERT-base INT8 (JPQD) model for question answering task can reach 5.15x compression rate to achieve 4.25x performance improvement on 4th Gen Intel® Xeon® Scalable Processors while improving Exact Match (1.35%) and F1 score (1.15%) metric on SQuAD compared with BERT-base FP32 model.
Reference
- Hugging Face Optimum Intel
- Hugging Face Neural Networks Block Movement Pruning
- Intel®Xeon® Processors Are Still the Only CPU With MLPerf Results, Raising the Bar By5x
Additional Resources
Provide Feedback & Report Issues
Notices & Disclaimers
Intel technologies may require enabled hardware, software, or service activation.
No product or component can be absolutely secure.
Your costs and results may vary.
Intel does not control or audit third-party data. You should consult other sources to evaluate accuracy.
Intel disclaims all express and implied warranties, including without limitation, the implied warranties of merchantability, fitness for a particular purpose, and non-infringement, as well as any warranty arising from course of performance, course of dealing, or usage in trade.
No license (express or implied, by estoppel or otherwise) to any intellectual property rights is granted by this document.
© Intel Corporation. Intel, the Intel logo, and other Intel marks are trademarks of Intel Corporation or its subsidiaries. Other names and brands may be claimed as the property of others.
OpenVINO™ Enable PaddlePaddle Quantized Model
OpenVINO™ is a toolkit that enables developers to deploy pre-trained deep learning models through a C++ or Python inference engine API. The latest OpenVINO™ has enabled the PaddlePaddle quantized model, which helps accelerate their deployment.
From floating-point model to quantized model in PaddlePaddle
Baidu releases a toolkit for PaddlePaddle model compression, named PaddleSlim. The quantization is a technique in PaddleSlim, which reduces redundancy by reducing full precision data to a fixed number so as to reduce model calculation complexity and improve model inference performance. To achieve quantization, PaddleSlim takes the following steps.
- Insert the quantize_linear and dequantize_linear nodes into the floating-point model.
- Calculate the scale and zero_point in each layer during the calibration process.
- Convert and export the floating-point model to quantized model according to the quantization parameters.
As the Figure1 shows, Compared to the floating-point model, the size of the quantized model is reduced by about 75%.
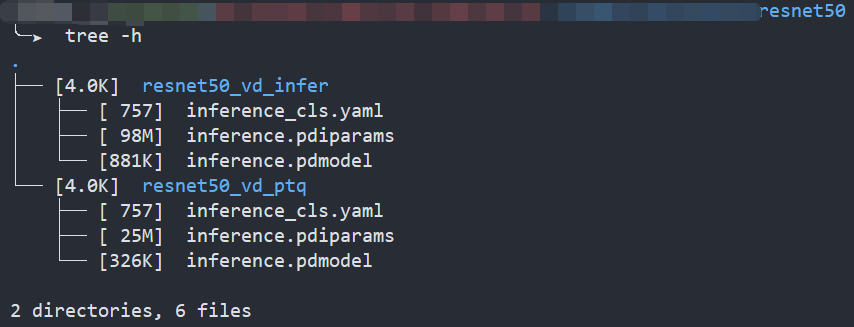
Enable PaddlePaddle quantized model in OpenVINO™
As the Figure2.1 shows, paired quantize_linear and dequantize_linear nodes appear intermittently in the model.

In order to enable PaddlePaddle quantized model, both quantize_linear and dequantize_linear nodes should be mapped first. And then, quantize_linear and dequantize_linear pattern scan be fused into FakeQuantize nodes and OpenVINO™ transformation mechanism will simplify and optimize the model graph in the quantization mode.
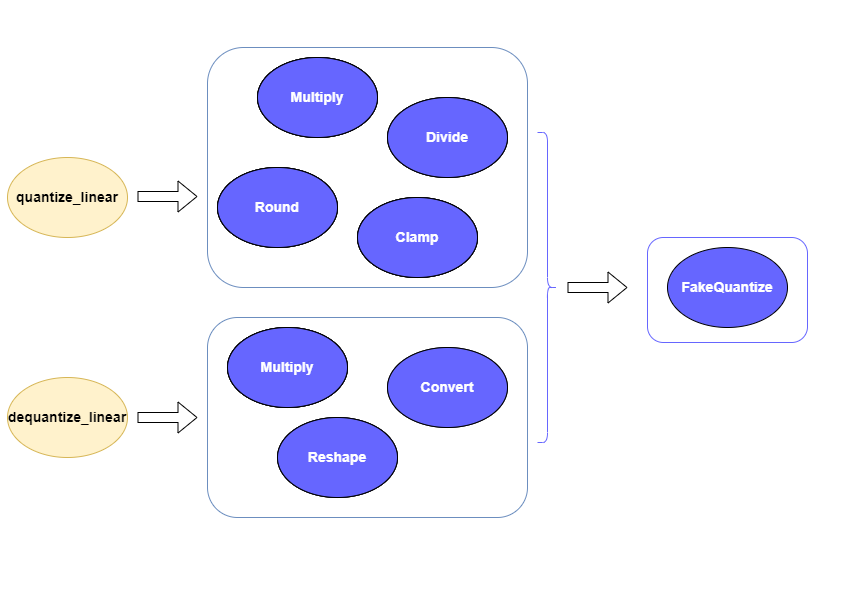
To check the kernel execution function, just profile and dump the execution progress, you can use benchmark_app as an example. The benchmark_app provides the option"-pc", which is used to report the performance counters information.
- To report the performance counters information of PaddlePaddle resnet50 float model, we can run the command line:

- To report the performance counters information of PaddlePaddle resnet50 quantized model, we can run the command line:

By comparing the Figure2.3 and Figure2.4, we can easily find that the hotpot layers of PaddlePaddle quantized model are dispatched to integer ISA implementation, which can accelerate the execution.
Accuracy
We compare the accuracy between resnet50 floating-point model and post training quantization(PaddleSlim PTQ) model. The accuracy of PaddlePaddle quantized model only decreases slightly, which is expected.
Performance
Throughput Speedup
The throughput of PaddlePaddle quantized resnet50 model can improve >3x.
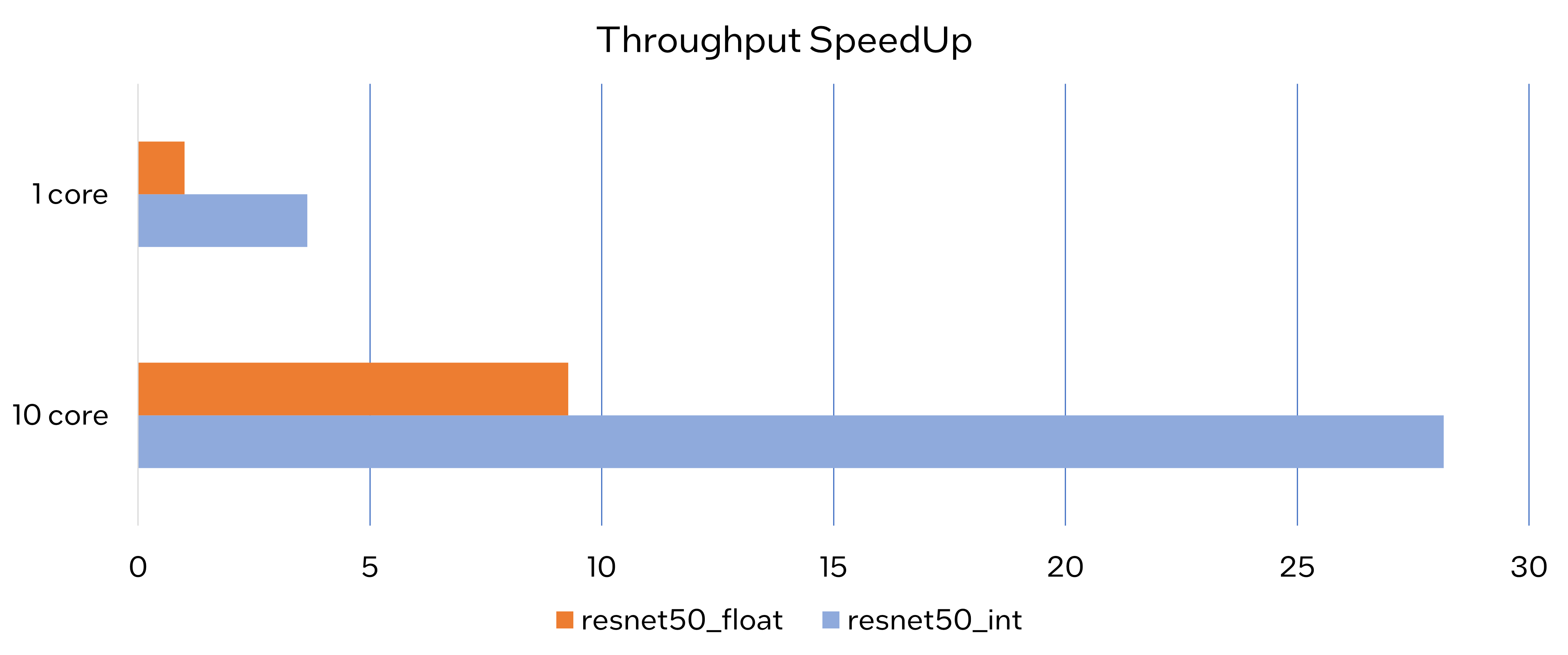
Latency Speedup
The latency of PaddlePaddle quantized resnet50 model can reduce about 70%.
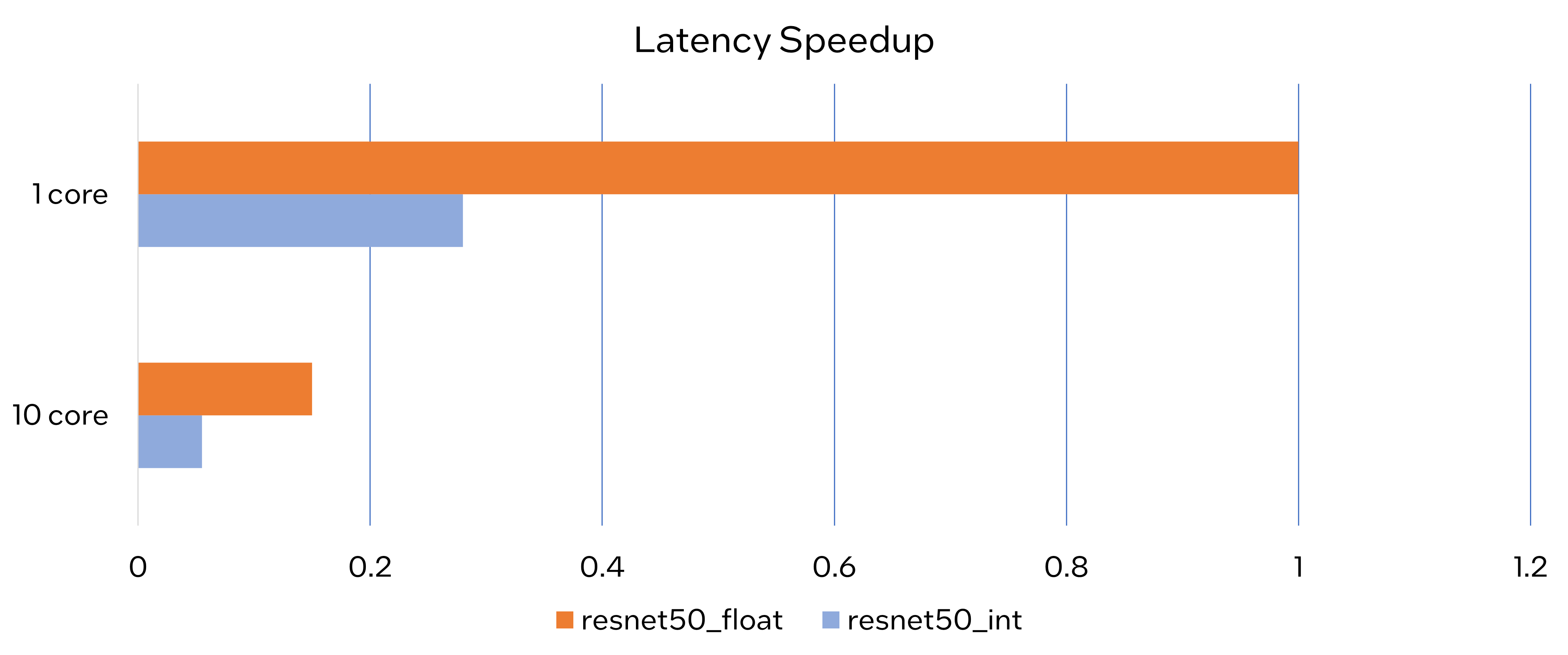
Conclusion
In this article, we elaborated the PaddlePaddle quantized model in OpenVINO™ and profiled the accuracy and performance. By enabling the PaddlePaddle quantized model in OpenVINO™, customers can accelerate both throughput and latency of deployment easily.
Notices & Disclaimers
- The accuracy data is collected based on 50000 images of val dataset in ILSVRC2012.
- The throughput performance data is collected by benchmark_app with data_shape "[1,3,224,224]" and hint throughput.
- The latency performance data is collected by benchmark_app with data_shape "[1,3,224,224]" and hint latency.
- The machine is Intel® Xeon® Gold 6346 CPU @3.10GHz.
- PaddlePaddle quantized model can be achieve at https://github.com/PaddlePaddle/FastDeploy/blob/develop/docs/en/quantize.md.
Q4'22: Technology update – low precision and model optimization
Authors
Alexander Kozlov, Pablo Munoz, Vui Seng Chua, Nikolay Lyalyushkin, Nikita Savelyev, Yury Gorbachev, Nilesh Jain
Summary
We still observe a lot of attention to the quantization and the problem of recovering accuracy after quantization. We highly recommend reading SmoothQuantpaper from the Highlights published by Song Han Lab about improving the accuracy of quantized Transformer models.
Highlights
- SmoothQuant: Accurate and Efficient Post-Training Quantization for Large Language Models by MIT and Nvidia (https://arxiv.org/pdf/2211.10438.pdf). A training-free, accuracy preserving, and general-purpose post-training quantization (PTQ) solution to enable 8-bit weight, 8-bit activation (W8A8)quantization for LLMs that can be implemented efficiently. SmoothQuant smooths the activation outliers by migrating the quantization difficulty from activations to weights with a mathematically equivalent transformation. Concretely, they compute activation statistics using a few sequences and then use these to scale down the activations and scale up the weights such that the worst-case outliers are minimized. Demonstrate up to 1.56× speedup and 2× memory reduction for LLMs with negligible loss in accuracy
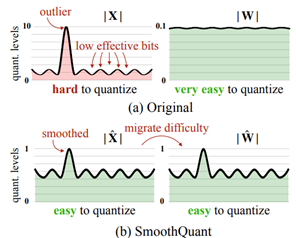
- CPT-V: A Contrastive Approach to Post-Training Quantization of Vision Transformers by University of Texas at Austin and ARM Inc. (https://arxiv.org/pdf/2211.09643.pdf). The method finds the optimal set of quantization scales that globally minimizes a contrastive loss without changing weights. CPT-V proposes a block-wise evolutionary search to minimize a global contrastive loss objective, allowing for accuracy improvement of existing vision transformer (ViT) quantization schemes. CPT-V improves the top-1 accuracy of a fully quantized ViT-Base by 10:30%, 0:78%, and 0:15% for 3-bit,4-bit, and 8-bit weight quantization levels.
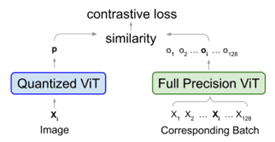
Papers with notable results
Quantization
- SUB-8-BIT QUANTIZATION FORON-DEVICE SPEECH RECOGNITION: A REGULARIZATION-FREE APPROACH by Amazon Alexa AI (https://arxiv.org/pdf/2210.09188.pdf). The paper introduces a method for ASR models compression that enables on-centroid weight aggregation without augmented regularizes. Instead, it leverages Softmax annealing to impose soft-to-hard quantization on centroids from the µ-Law constrained space. The method supports different quantization modes for a wide range of granularity: different bit depths can be specified for different kernels/layers/modules. The method allows compressing a Conformer into sub-5-bit with more than 6x model size reduction and Bifocal RNN-T into5-bit that reduces the memory footprint by 30.73% and P90 user-perceived latency by 31.30% compared to INT8.
- Outlier Suppression: Pushing the Limit of Low-bit Transformer Language Models by SenseTime Research and Chinese Universities (https://arxiv.org/pdf/2209.13325.pdf). Propose an outlier suppression framework including two components: Gamma Migration and Token-Wise Clipping. The Gamma Migration produces a more quantization-friendly model by migrating the outlier amplifierγ of LayerNorm into subsequent modules in an equivalent transformation and bringing more robust activation for quantization without extra computation burden. The Token-Wise Clipping further efficiently finds a suitable clipping range with minimal final quantization loss in a coarse-to-fine procedure. The coarse-grained stage, which leverages the fact that those less important outliers only belong to a few tokens, can obtain a preliminary clipping range quickly in a token-wise manner. The fine-grained stage then optimizes it. However, it onlysucceeds on small language models such as BERT, RoBERTa, BART and fails to maintain the accuracy for LLMs. The PyTorch implementation is available: https://github.com/wimh966/outlier_suppression.
- GPTQ: ACCURATE POST-TRAININGQUANTIZATION FOR GENERATIVE PRE-TRAINED TRANSFORMERS by ETH Zurich and IST Austria (https://arxiv.org/pdf/2210.17323.pdf). The paper introduces a method for low-bit quantization of transformer models. Essentially, the method combines Layer-wise fine-tuning of quantization parameters with Optimal Brain Quantization method for bit-width selection. The Code is available here: https://github.com/IST-DASLab/gptq.
- NoisyQuant: Noisy Bias-Enhanced Post-Training Activation Quantization for Vision Transformers by Nanji, Peking and Berkeley universities (https://arxiv.org/pdf/2211.16056.pdf).Authors provide a theoretical justification for a way to reduce the quantization error of heavy-tailed distributions with a fixed additive noisy bias. They propose a method for a quantizer-agnostic enhancement for post-training quantization (PTQ) performance on activation quantization. The method is applied on top of existing PTQ quantizers and shows superior results for Vision Transformer models trained on ImageNet dataset: up to 1.7% improvement for a linear quantization scheme up to 0.7% for a nonlinear one.
- Exploiting the Partly Scratch-off Lottery Ticket for Quantization-Aware Training by Tencent and Chinese Universities (https://arxiv.org/pdf/2211.08544.pdf). In this paper, the authors claim the phenomenon that a large portion of quantized weights reaches the optimal quantization level after a few training epochs. Based on this observation, they zero out gradient calculations of these weights in the remaining training period to avoid meaningless updating. To find the ticket, authors develop a heuristic method that freezes a weight once the distance between the full-precision one and its quantization level is smaller than a controllable threshold. The method helps to eliminate 30%-60%weight updating and 15%-30% FLOPs of the backward pass, while keeping the baseline performance. For example, it improves 2-bit ResNet-18 by 1.41%,eliminating 56% weight updating and 28% FLOPs of the backward pass. Code is at https://github.com/zysxmu/LTS.
- QFT: Post-training quantization via fast joint finetuning of all degrees of freedom by Hailo AI (https://arxiv.org/pdf/2212.02634.pdf).The paper proposes a modification of the layer-wise/channel-wise post-training quantization method where all the parameters are trained jointly including the layer weights, quantization scales, cross-layer factorization parameters to reduce the overall quantization error. The training setup is common and uses the original model as a teacher for layer-wise KD. The method achieves results in a4-bit, 8-bit quantization setup.
- Make RepVGG Greater Again: A Quantization-aware Approach by Meituan (https://arxiv.org/pdf/2212.01593.pdf). An interesting read about the challenges in the quantization of RepVGG model. Authors analyze what exactly leads to the significant accuracy degradation when quantizing this model to 8-bits. They found a high variance in activations of some layers which is induced by the model architecture. They propose several tricks (essentially normalization and regularization changes) that can be applied along with QAT. With such changes, they can achieve < 2% of accuracy degradation. BTW, OpenVINO team finding is that using FP8 data types it is possible to stay within ~1% of accuracy drop compared to FP32 baseline without bells and whistles. Only scaling and Bias Correction are required.
- A Closer Look at Hardware-Friendly Weight Quantization by Google Research (https://arxiv.org/pdf/2210.03671.pdf). Authors study the two quantization scale estimation methods to identify the sources of performance differences between the two classes, namely, sensitivity to outliers and convergence instability of the quantizer scaling factor. It is done in strong HW constraints: uniform, per-tensor, symmetric quantization. They propose various techniques to improve the performance of both quantization methods - they fix the optimization instability issues present in the MSQE-based methods during the quantization of MobileNet models and allow us to improve the validation performance of the gradient-based methods. The proposed method achieves superior results in those constraints.
- CSMPQ: CLASS SEPARABILITYBASED MIXED-PRECISION QUANTIZATION by universities of China (https://arxiv.org/pdf/2212.10220.pdf). The paper introduces the class separability of layer-wise feature maps to search for optimal quantization bit-width. Essentially, authors leverage the TF-IDF metric from NLP to measure the class separability of layer-wise feature maps that are averaged across spatial dimensions. The method can be applied on top of the existing quantization algorithms, such as BRECQ and delivers good results, e.g. 71.30% top-1 acc with only 1.5Mb on MobileNetV2.
Pruning
- Structured Pruning Adapters by Aarhus University and Cactus Communications (https://arxiv.org/pdf/2211.10155.pdf). The paper introduces task-switching network adapters that accelerate and specialize networks for downstream tasks. Authors propose channel- and block-based adaptors and evaluate them with a suite of pruning methods on both computer vision and natural language processing benchmarks. The method achieves comparable results when downstream ResNEt-50 ImageNet to CIFAR, Flowers, Cats and Dogs and BERT to SQuAD v1.1. The code is available at: https://github.com/lukashedegaard/structured-pruning-adapters.
- HeatViT: Hardware-Efficient Adaptive Token Pruning for Vision Transformers by Northeastern University, Simon Fraser University, and CoCoPIE LLC (https://arxiv.org/pdf/2211.08110.pdf). The paper introduces an algorithm and FPGA co-design for a token selector to enable image adaptive token pruning in Visual Transformers. Authors also propose a latency-aware multi-stage training strategy to learn the insertion of token selectors in ViTs. They also replace non-linearities inside ViT models with polynomial approximations and stack token pruning with 8-bit quantization. The method can achieve 28.4%∼65.3% computation reduction, for various widely used ViTs on the ImageNet, and 3.46×∼4.89× speedup with a trivial resource utilization overhead on FPGA.
- Soft Masking for Cost-Constrained Channel Pruning by Stanford University and Nvidia (https://arxiv.org/pdf/2211.02206.pdf). Authors propose a filter pruning method with a soft mask re-parameterization of the network weights so that channel sparsity can be adaptively rewired. They also apply a scaling technique for the batch normalization weights to mitigate gradient instability at high channel pruning ratios. To perform channel pruning subject to a cost constraint, authors reformulate it as the multiple-choice knapsack problem. The method achieves SOTA results on ImageNet and VOC tasks. The code is available at: https://github.com/NVlabs/SMCP.
- Pruning’s Effect on Generalization Through the Lens of Training and Regularization by MIT, University of Toronto, Mosaic ML, and Google Brain (https://arxiv.org/pdf/2210.13738.pdf). Authors study the impact of model pruning on the generalization capabilities. Even though the study is conducted on toy examples, it’s quite extensive and proves known facts that pruning can be considered as an additional regularization and can lead to better training results.
- oViT: An Accurate Second-Order Pruning Framework for Vision Transformers by Yandex, Neural Magic, and IST Austria (https://arxiv.org/pdf/2210.09223.pdf). Authors introduce an approximate second-order pruner for Vision Transformer models that estimates sparsity ratios for different parts of the model. They also provide a set of general sparse fine-tuning recipes, enabling accuracy recovery at reasonable computational budgets. In addition, the authors propose a pruning framework that produces sparse accurate models for a sequence of sparsity targets in a single run, accommodating various deployments under a fixed compute budget. The method is evaluated on various ViT models including classical ViT, DeiT, XCiT, EfficientFormer and Swin, and shows SOTA results(e.g. 75% of sparsity at <1% of accuracy drop).
- A Fast Post-Training Pruning Framework for Transformers by UC Berkeley and Samsung (https://arxiv.org/pdf/2204.09656.pdf). The proposed method prunes Transformer models without any fine-tuning. When applied to BERT and DistilBERT authors achieve 2.0x reduction in FLOPs and 1.56x speedup in inference latency while maintaining < 1% accuracy loss. Notably, the whole pruning process finishes in less than 3 minutes on a single GPU. The method consists of three main stages: (1) a lightweight mask search algorithm finds which Transformer heads and filters to prune based on the Fisher information (2) mask rearrangement that improves binary masks produced by the previous stage and (3) mask tuning tweaks some of the 1's in the mask by making them real-valued.
- Fast DistilBERT on CPUs by Intel (https://arxiv.org/pdf/2211.07715.pdf). The work proposes a new pipeline to apply Prune-OFA with block-wise structured pruning, jointly with quantize-aware training and distillation. The work also provides an advanced Int8 sparse GEMM inference engine which is friendly to Intel VNNI instructions as a companion runtime to accelerate the resultant model from the proposed pipeline. DistilBERT/SQuADv1.1 optimized by the pipeline and deployed with the new engine outperforms Neural Magic’s proprietary sparse inference engine in throughput performance (under production latency constraint) by 50% and 4.1X over low-precision performance of ONNXRuntime. Source code can be found at https://github.com/intel/intel-extension-for-transformers.
Neural Architecture Search
- EZNAS: Evolving Zero-Cost Proxies for Neural Architecture Scoring by Intel Labs (https://openreview.net/forum?id=lSqaDG4dvdt). Authors propose a genetic programming approach to automate the discovery of zero-cost neural architecture scoring metrics. The discovered metrics outperform existing hand-crafted metrics and generalize well across neural architecture design spaces. Two search spaces are explored using EZNAS:NASBench-201 and Network Design Spaces (NDS), demonstrating the strong generalization capabilities of the discovered zero-cost metrics.
- Resource-Aware Heterogenous Federated Learning using Neural Architecture Search by Iowa State University and Intel Labs (https://arxiv.org/pdf/2211.05716.pdf). This paper proposes a framework for Resource-aware Federated Learning (RaFL). The framework uses Neural Architecture Search (NAS)to enable on-demand specialized model deployment for resource-diverse edge devices. Furthermore, the framework uses a novel model architecture fusion scheme to allow for the aggregation of the distributed learning results. RaFL demonstrates superior resource efficiency and reduction in communication overhead compared to state-of-the-art solutions.
- NAS-LID: Efficient Neural Architecture Search with Local Intrinsic Dimension by Nvidia and universities of China, India, and GB (https://arxiv.org/pdf/2211.12759.pdf). Authors first apply the so-called local intrinsic dimension (LID) method that evaluates the geometrical properties of sampled model architectures by calculating the low-cost LID features layer-by-layer, and the similarity characterized by LID. The method can be embedded into the existing NAS frameworks, e.g. OFA of Proxyless NAS. The method significantly accelerates architecture search time and shows comparable performance on public benchmarks. The code is available: https://github.com/marsggbo/NAS-LID.
- Automatic Subspace Evoking for Efficient Neural Architecture Search by Hisense and universities of China (https://arxiv.org/pdf/2210.17180.pdf). A method that proposes to decouple architecture search into global and local search steps that are aimed at enhancing the performance of NAS. Specifically, we first perform a global search to find promising subspaces and then perform a local search to obtain the resultant architectures. The method exploits GNN and RNN models in the search algorithm that are trained jointly. The method shows superior results compared to some well-known NAS frameworks.
- AUTOMOE: NEURAL ARCHITECTURESEARCH FOR EFFICIENT SPARSELY ACTIVATED TRANSFORMERS by Microsoft Research and University of British Columbia (https://arxiv.org/pdf/2210.07535.pdf). Authors introduce heterogeneous search space for Transformers consisting of variable number, FFN size and placement of experts in both encoders and decoders; variable number of layers, attention heads and intermediate FFN dimension of standard Transformer modules. They extend Supernet training to this new search space which combines all possible sparse architectures into a single graph and jointly trains them via weight-sharing. They also use an evolutionary algorithm to search for optimal sparse architecture from Supernet with the best possible performance on a downstream task. The method shows better results compared to dense NAS methods for Transformers.
Other
- GhostNetV2: Enhance Cheap Operation with Long-Range Attention by Huawei, Peking University, and University of Sydney (https://arxiv.org/pdf/2211.12905.pdf). In this paper, authors propose a hardware-friendly attention mechanism (dubbed DFC attention) and GhostNetV2 architecture for mobile applications. The proposed DFC attention is aimed at capturing the dependence between long-range pixels. They also revisit the expressiveness bottleneck in previous GhostNet and propose to enhance expanded features so that a GhostNetV2 block can aggregate local and long-range information simultaneously. The approach shows good results, 75.3% top-1 accuracy on ImageNet with 167M FLOPs. The code is available at https://github.com/huawei-noah/Efficient-AI-Backbones/tree/master/ghostnetv2_pytorch.
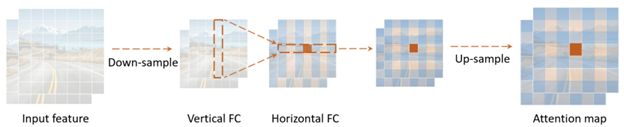
Deep Learning Software
- Nivida open-sourced Transformer Engine – a plugin that helps to train Transformers in FP8 data types: https://github.com/NVIDIA/TransformerEngine.
- Accelerate your models with HuggingFace Optimum Intel and OpenVINO: A blog post about new API capabilities to run Transformer models on Intel CPU and GPU with OpenVINO and optimize with NNCF – https://huggingface.co/blog/openvino.
Deep Learning Hardware
- Faster Training and Inference: Habana Gaudi®-2 vs Nvidia A100 80GB: A post about Habana Gaudi 2 accelerating model training and inference in AWS – https://huggingface.co/blog/habana-gaudi-2-benchmark.
- Alibaba Cloud unveils the new Arm server chip - Yitian 710, with the 5nm process, withInt8 MatMul, BFloat16.
Q4'24: Technology Update – Low Precision and Model Optimization
Authors
Alexander Kozlov, Nikolay Lyalyushkin, Nikita Savelyev, Souvikk Kundu, Andrey Anufriev, Pablo Munoz, Alexander Suslov, Liubov Talamanova, Daniil Lyakhov, Yury Gorbachev, Nilesh Jain, Maxim Proshin
Summary
What a quarter! Tons of works for Transformer model optimization in Q4’24 including fundamental ones such as “scaling lows for quantized LLMs“. Such a huge effort can indicate a growing adoption of LLMs and AI in general and the need for a further cost reduction. We had to extend the Highlights to six papers this time considering the amount of work being done.
Highlights
- Scaling Laws for Precision by Harvard, Stanford, MIT, Carnegie Mellon Universities, and Databricks (https://arxiv.org/pdf/2411.04330). In this work, authors devise “precision-aware” scaling laws for both training and inference. They propose that training in lower precision reduces the model’s effective parameter count, allowing predicting the additional loss incurred from training in low precision and post-train quantization. For inference, they find that the degradation introduced by post-training quantization increases as models are trained on more data, eventually making additional pretraining data actively harmful. For training, their scaling laws allow predicting the loss of a model with different parts in different precisions and suggest that training larger models in lower precision may be compute optimal. Authors unify the scaling laws for post and pretraining quantization to arrive at a single functional form that predicts degradation from training and inference in varied precisions. They fit on over 465 pretraining runs and validate our predictions on model sizes up to 1.7B parameters trained on up to 26B tokens.
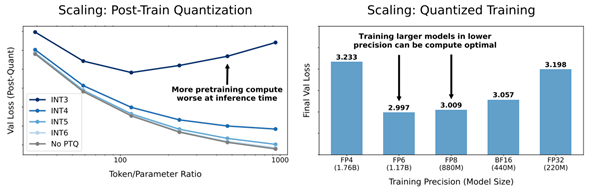
- Low-Bit Quantization Favors Undertrained LLMs: Scaling Laws for Quantized LLMs with100T Training Tokens by University of Virginia, Tencent AI Lab Seattle (https://arxiv.org/pdf/2411.17691).Authors propose a perspective that one can use to measure an LLM’s training levels and determine the number of training tokens required for fully training LLMs of various sizes. Moreover, authors use the scaling laws to predict the quantization performance of different-sized LLMs trained with 100 trillion tokens. Our projection shows that the low-bit quantization performance of future models, which are expected to be trained with over 100 trillion tokens, may NOT be desirable. This poses a potential challenge for low-bit quantization in the future and highlights the need for awareness of a model’s training level when evaluating low-bit quantization research. Checkpoints are available at: https://huggingface.co/Xu-Ouyang.

- Hymba: A Hybrid-head Architecture for Small Language Models by Nvidia, Georgia Institute of Technology, and HKUST (https://www.arxiv.org/abs/2411.13676).The paper introduces a family of small language models featuring a hybrid-head parallel architecture that integrates transformer attention mechanisms with state space models (SSMs) for enhanced efficiency. Additionally, authors introduce learnable meta tokens that are prepended to prompts, storing critical information. This model is further optimized by incorporating cross-layer key-value (KV) sharing and partial sliding window attention, resulting in a compact cache size. Hymba-1.5B-Base model surpasses all sub-2B public models in performance and even outperforms Llama-3.2-3B with1.32% higher average accuracy, an 11.67× cache size reduction, and 3.49×throughput. Models are available on the Hugging Face Hub.

- THE SUPER WEIGHT IN LARGE LANGUAGE MODELS by Apple and University of Notre Dame (https://arxiv.org/pdf/2411.07191). This work presents a finding that pruning single parameters can destroy an LLM’s ability to generate text – increasing perplexity by 3 orders of magnitude and reducing zero-shot accuracy to guessing. It proposes a data-free method for identifying such parameters, termed super weights, using a single forward pass through the model. Authors find that these super weights induce correspondingly rare and large activation outliers, termed super activations. When preserved with high precision, super activations can improve simple round-to-nearest quantization to become competitive with state-of-the-art methods. For weight quantization, they similarly find that by preserving the super weight and clipping other weight outliers, round-to-nearest quantization can scale to much larger block sizes than previously considered. The code is available at n https://github.com/mengxiayu/LLMSuperWeight.

- Pushing the Limits of Large Language Model Quantization via the Linearity Theorem by Yandex, HSE University, ISTA, GenAI CoE, KAUST, Neural Magic (https://arxiv.org/pdf/2411.17525). The paper presents a “linearity theorem” establishing a direct relationship between the layer-wise ℓ2 reconstruction error and the model perplexity increase due to quantization. This enables two novel applications: (1) a simple data-free LLM quantization method using Hadamard rotations and MSE-optimal grids, dubbed HIGGS, which outperforms all prior data-free approaches such as the extremely popular NF4 quantized format, and (2) an optimal solution to the problem of finding non-uniform per-layer quantization levels which match a given compression constraint in the medium-bit width regime, obtained by reduction to dynamic programming. Authors demonstrate improved accuracy-compression trade-offs on Llama-3.1 and 3.2- family models, as well as on Qwen-family models.

- SANA:EFFICIENT HIGH-RESOLUTION IMAGE SYNTHESIS WITH LINEAR DIFFUSION TRANSFORMERS by NVIDIA, MIT, Tsinghua University (https://arxiv.org/pdf/2410.10629). Authors introduce Sana, a text-to-image frame work that can generate images up to 4096×4096 resolution. Core designs include: (1) Deep compression autoencoder: unlike traditional AEs, which compress images only 8×,authors trained an AE that can compress images 32×, effectively reducing the number of latent tokens. (2) Linear DiT: they replace all vanilla attention in DiT with linear attention (3) Decoder-only text encoder: they replaced T5 with modern decoder-only small LLM as the text encoder and designed complex human instruction with in-context learning to enhance the image-text alignment. (4) Efficient training and sampling: they propose Flow-DPM-Solver to reduce sampling steps. As a result, Sana-0.6B is very competitive with modern giant diffusion model (e.g. Flux-12B), being 20times smaller and 100+ times faster in measured throughput. Project web page with code: https://nvlabs.github.io/Sana/.

Papers with notable results
Quantization
- VPTQ: EXTREME LOW-BIT VECTOR POST-TRAINING QUANTIZATION FOR LARGE LANGUAGE MODELS by Microsoft and University of Science and Technology of China (https://arxiv.org/abs/2409.17066). The authors introduce Vector Post-Training Quantization and use Second-Order Optimization to formulate the LLM VQ problem and guide the algorithm design by solving the optimization. They further refine the weights using Channel-Independent Second-Order Optimization for a granular VQ. In addition, by decomposing the optimization problem, authors propose a brief codebook initialization algorithm and extend VPTQ to support residual and outlier quantization, which enhances model accuracy and further compresses the model. The method achieves good results on llama-2 and llama-3 model families, resulting in a 1.6-1.8× increase in inference throughput compared to SOTA. The code is available at https://github.com/microsoft/VPTQ.
- ADDITION IS ALL YOU NEED FOR ENERGY-EFFICIENT LANGUAGE MODELS by BitEnergy AI (https://arxiv.org/pdf/2410.00907). Authors propose the linear-complexity multiplication algorithm that approximates floating point number multiplication with integer addition operations. The new algorithm costs significantly less computation resource than 8-bit floating point multiplication but achieves higher precision. Compared to 8-bit floating point multiplications, the proposed method achieves higher precision but consumes significantly less bit-level computation which can potentially reduce 95% energy cost by elementwise floating point tensor multiplications and 80% energy cost of dot products. A numerical analysis and experiments indicate that the method with 4-bit mantissa achieves comparable precision as float8 e4m3 multiplications, and with 3-bit mantissa outperforms float8 e5m2. Evaluation results on popular benchmarks show that directly applying L-Mul to the attention mechanism is almost lossless.
- BitNet a4.8: 4-bit Activations for 1-bit LLMs by Microsoft and University of Chinese Academy of Sciences (https://arxiv.org/pdf/2411.04965). In this work, authots introduce BitNet a4.8, enabling 4-bit activations for 1-bit LLMs. BitNet a4.8 employs a hybrid quantization and sparsification strategy to mitigate the quantization errors introduced by the outlier channels. Specifically, they utilize 4-bit activations for inputs to the attention and feed-forward network layers, while sparsifying intermediate states followed with 8-bit quantization. Extensive experiments demonstrate that BitNet a4.8 achieves performance comparable to BitNet b1.58 with equivalent training costs, while being faster in inference with enabling 4-bit (INT4/FP4) kernels. Additionally, BitNet a4.8 activates only 55% of parameters and supports 3-bit KV cache.
- MagR: Weight Magnitude Reduction for Enhancing Post-Training Quantization by Uniiversity at Albany and IBM (https://arxiv.org/pdf/2406.00800). MagR is an optimization-based preprocessing technique for improving post-training quantization. It solves an l_∞-regularized problem to reduce outlier weights and center them around zero, enabling smoother and more efficient quantization. Unlike linear transformations that require extra steps at inference, MagR is a non-linear transformation that adds no overhead. Experiments show state-of-the-art results, including a Wikitext2 perplexity of 6.7 on the LLaMA2-70B model using per-channel INT2 weight quantization.
- Cherry on Top: Parameter Heterogeneity and Quantization in Large Language Models by Shanghai University of Finance and Economics (https://arxiv.org/pdf/2404.02837). This paper identifies “cherry” parameters in large language models—those few parameters with a disproportionately large effect on performance—while most parameters matter far less. Building on this insight, the authors introduce CherryQ, a quantization technique that maintains these critical parameters in high precision and aggressively quantizes the rest. CherryQ delivers improved perplexity and downstream task results, enabling efficient LLM deployment. Remarkably, a 3-bit quantized Vicuna-1.5 model matches the performance of 16-bit models, illustrating the potential of leveraging parameter heterogeneity for more efficient inference.
- QTIP: Quantization with Trellises and Incoherence Processing by Cornell University (https://arxiv.org/pdf/2406.11235). QTIP is a new PTQ approach leveraging trellis-coded quantization (TCQ) for ultra-high-dimensional vector quantization of LLM weights. Unlike conventional VQ methods whose codebook size grows exponentially with dimension, TCQ uses a stateful decoder to maintain efficiency as dimensions scale. QTIP provides a hardware-friendly “bitshift” trellis structure and can be tuned for lookup-only or computed lookup-free decoding. This allows faster, more memory-efficient inference and achieves state-of-the-art quantization quality, outperforming previous VQ-based methods.
- ESPACE: Dimensionality Reduction of Activations for Model Compression by NVIDIA (https://arxiv.org/pdf/2410.05437). ESPACE introduces a new LLM compression method based on dimensionality reduction of activations rather than weight decomposition. By projecting activations onto pre-calibrated principal components, ESPACE retains model expressivity without retraining. It achieves weight compression indirectly through matrix multiplication associativity. Theoretically, it ensures optimal computational accuracy when constructing projection matrices. Experiments show up to 50% compression on GPT3, Llama2, and Nemotron4 with minimal accuracy loss, and in some cases, improved perplexity. ESPACE also speeds up inference. Compared to existing tensor decomposition methods, ESPACE advances state-of-the-art LLM compression.
- Delta-CoMe: Training-Free Delta-Compression with Mixed-Precision for Large Language Models by several Chinese universities (https://arxiv.org/pdf/2406.08903). This work addresses compressing delta weights for fine-tuned LLMs, where maintaining task-specific performance is challenging using low-rank or low-bit methods. Observing that delta weights’ singular values are long-tailed, the authors propose a mixed-precision delta quantization approach. By assigning higher-bit precision to more influential singular vectors, their method preserves accuracy. Experiments on diverse fine-tuned LLMs—including math, code, and chat models—show that this approach matches full-precision performance and significantly outperforms standard low-rank and low-bit baselines. It is also compatible with various backbone models, such as Llama-2, Llama-3, and Mistral.
- StepbaQ: Stepping backward as Correction for Quantized Diffusion Models by MediaTek and Purdue University (https://openreview.net/pdf?id=cEtExbAKYV). StepbaQ reframes quantization error in diffusion models as a “stepback” in their denoising process. By analyzing how this accumulated error distorts the sampling trajectory, StepbaQ introduces a correction mechanism that uses quantization error statistics from a small calibration dataset. Without altering quantization settings, it significantly improves model quality. For instance, StepbaQ boosts the FID score of quantized SD v1.5 by 7.30 under W8A8, and SDXL-Turbo by 17.31 under W4A8. This plug-and-play solution enhances performance on resource-constrained devices while maintaining broad applicability.
- LLMCBench: Benchmarking Large Language Model Compression for Efficient Deployment by Beihang University, ETH Zurich and Canerige Mellon University (https://arxiv.org/pdf/2410.21352). LLMCBench is a comprehensive benchmark designed to evaluate large language model compression techniques under realistic conditions. Moving beyond limited and specialized assessments, it tests various models, datasets, and metrics. LLMCBench establishes clearly defined evaluation tracks based on real production requirements and conducts extensive experiments with multiple mainstream compression methods. Through in-depth analysis, it offers insights into the strengths and weaknesses of these approaches. Ultimately, LLMCBench aims to guide the selection and design of effective compression algorithms, serving as a valuable resource for future research and development in LLM efficiency.
- DuQuant: Distributing Outliers via Dual Transformation Makes Stronger Quantized LLMs (https://duquant.github.io/). Generalization of the SmoothQuant algorithm which allows to mitigate the massive outliers and quantize not just LLM weights but activations as well. Shows promising results for LLama2/3 -8B W6A6 and W4A4 quantization. The code is available at: https://github.com/Hsu1023/DuQuant.
- Efficient Multi-task LLM Quantization and Serving for Multiple LoRA Adapters (https://openreview.net/pdf?id=HfpV6u0kbX). Multi quantized Lora adapters quantization via techniques like Multi-Lora GPTQ and LoRa Inlaid. Technics to dynamically add a new task/dataset to existing quantized LLM are discussed in the paper, promising pipeline for quantized LLM serving / update is presented.
- PROGRESSIVE MIXED-PRECISION DECODING FOR EFFICIENT LLM INFERENCE. Samsung AI Center, Cambridge UK, Imperial College London UK (https://arxiv.org/abs/2410.13461). The authors propose a novel phase-aware method that selectively allocates precision during different phases of LLM inference, achieving both strong context extraction during prefill and efficient memory bandwidth utilization during decoding. To further address the memory-boundedness of the decoding phase, the authors introduce Progressive Mixed-Precision Decoding (PMPD), a technique that enables the gradual lowering of precision deeper in the generated sequence, together with a spectrum of precision-switching schedulers that dynamically drive the precision lowering decisions in either task-adaptive or prompt-adaptive manner. Extensive evaluation across diverse language tasks shows that when targeting Nvidia GPUs, PMPD achieves 1.4−12.2× speedup in LLM linear layers over fp16 models, while when targeting an LLM-optimized NPU, our approach delivers a throughput gain of 3.8−8.0× over fp16 models and up to 1.54× over uniform quantization approaches while preserving the output quality.
- AMXFP4: TAMING ACTIVATION OUTLIERS WITH ASYMMETRIC MICROSCALING FLOATING-POINT FOR 4-BIT LLM INFERENCE by Hanyang University and Rebellions Inc. (https://arxiv.org/pdf/2411.09909). Authors propose Asymmetric Microscaling 4-bit Floating-Point (AMXFP4) for efficient LLM inference. This data format leverages asymmetric shared scales to mitigate outliers while naturally capturing the asymmetry introduced by group-wise quantization. Unlike conventional 4-bit quantization methods that rely on data rotation and costly calibration, AMXFP4 uses asymmetric shared scales for direct 4-bit casting, achieving better quantization accuracy across various LLM tasks, including multi-turn conversations, long-context reasoning, and visual question answering The code is available at https://github.com/aiha-lab/MX-QLLM.git.
- SageAttention2 Technical Report: Accurate 4 Bit Attention for Plug-and-play Inference Acceleration by Tsinghua University (https://arxiv.org/pdf/2411.10958). Authors propose an improvement over the previous version of SageAttention method which utilizes 4-bit matrix multiplication (Matmul) alongside additional precision-enhancing techniques. First, they propose to quantize matrixes (Q, K) to INT4 in a warp-level granularity and quantize matrixes to FP8. Second, they propose a method to smooth Q and V, enhancing the accuracy of attention. Third, they propose an adaptive quantization method to ensure the end-to-end metrics over various models. Authors claim a good performance improvement at small drop of accuracy for large language processing, image generation, and video generation. The codes are available at https://github.com/thu-ml/SageAttention.
- CATASTROPHIC FAILURE OF LLM UNLEARNING VIA QUANTIZATION (https://openreview.net/pdf?id=lHSeDYamnz). The paper reveals that applying quantization to models that have undergone unlearning can restore the "forgotten" information. Authors conduct experiments using various quantization techniques across multiple precision levels to evaluate this phenomenon. They find that for unlearning methods with utility constraints, the unlearned model retains an average of 21% of the intended forgotten knowledge in full precision, which significantly increases to 83% after 4-bit quantization. They also provide a theoretical explanation for the observed phenomenon and propose a quantization-robust unlearning strategy aimed at mitigating this intricate issue. Results highlight a fundamental tension between preserving the utility of the unlearned model and preventing knowledge recovery through quantization, emphasizing the challenge of balancing these two objectives. The code is available at: https://anonymous.4open.science/r/FailureUnlearning-20DE.
- Llama Guard 3-1B-INT4: Compact and Efficient Safeguard for Human-AI Conversations by Meta (https://arxiv.org/pdf/2411.17713). Author used a complex approach to optimize Llama Guard 3-1B for mobile platforms. Namely, they reduce the number of decoder blocks and MLP width of Llama Guard 3-1B-INT4 using a block-level and neuron-level sensitivity analysis, respectively. They use quantization-aware training (QAT) to reduce the weight bitwidth to 4 and the activation bitwidth to 8, such that the model size is cut down by 4× and the model can be efficiently run via ExecuTorch’s XNNPACK backend. They make use of the fact that Llama Guard models only require a limited output vocabulary and reduce the unembedding layer output shape from 128k to 20. Finally, the authors fine-tune the model with distillation from a Llama Guard 2-8B teacher to recover any lost model quality resulting from the compression steps.
- MPQ-DM: Mixed Precision Quantization for Extremely Low Bit Diffusion Models by Institute of Computing Technology, University of Chinese Academy of Sciences, ETH Zurich, Beijing Jiaotong University (https://arxiv.org/pdf/2412.11549). The paper presents a Mixed-Precision Quantization method for Diffusion Models. It mainly relies on two techniques: (1) To mitigate the quantization error caused by outlier severe weight channels, authors propose an Outlier-Driven Mixed Quantization (OMQ) technique that uses Kurtosis to quantify outlier salient channels and apply optimized intra-layer mixed-precision bit-width allocation to recover accuracy performance within target efficiency. (2) To robustly learn representations crossing time steps, they construct a Time-Smoothed Relation Distillation (TRD) scheme between the quantized diffusion model and its full-precision counterpart, transferring discrete and continuous latent to a unified relation space to reduce the representation inconsistency. The method achieves good generation results on public benchmarks in low-bit quantization settings, e.g. W3A6, W3A4. Code is planned to be released here.
- Panacea: Novel DNN Accelerator using Accuracy-Preserving Asymmetric Quantization and Energy-Saving Bit-Slice Sparsity by POSTECH, University of Michigan (https://arxiv.org/pdf/2412.10059). The paper discloses how to build AI accelerator that leverages Bit-Slice Sparsity for the most prominent integer quantization scheme W-sym, A-asym. In contrast to the previous bit-slice computing, the accelerator compresses frequent nonzero slices, generated by asymmetric quantization, and skips their operations. To increase the slice level sparsity of activations, authors also introduce two algorithm hardware co-optimization methods: a zero-point manipulation and a distribution-based bit-slicing.
- Efficiency Meets Fidelity: A Novel Quantization Framework for Stable Diffusion by Zhejiang University and vivo Mobile Communication Co (https://arxiv.org/pdf/2412.06661). The paper introduces a mix-precision quantization strategy, multi-timestep activation quantization, and time information precalculation techniques to ensure high fidelity image generation of Stable Diffusion models in comparison to floating-point counterparts. The method achieves a good consistency of the image generation under the W8A8 and W4A8 settings.
- PREFIXQUANT: STATIC QUANTIZATION BEATS DYNAMIC THROUGH PREFIXED OUTLIERS IN LLMS by The University of Hong Kong, Shanghai AI Laboratory, Tongji University (https://arxiv.org/pdf/2410.05265). The paper proposes a technique that isolates outlier tokens offline without re-training. Specifically, it identifies high-frequency outlier tokens and prefixes them in the KV cache, preventing the generation of outlier tokens during inference and simplifying quantization. The method achieves very promising results in LLM static quantizaiton. For instance, in W4A4KV4 Llama-3-8B, with per-tensor static quantization it achieves a 7.43 WikiText2 perplexity and 71.08% average accuracy on 5 common-sense reasoning tasks. Additionally, the inference speed of W4A4 quantized models using PrefixQuant is 1.60× to 2.81× faster than FP16. The code is available at https://github.com/ChenMnZ/PrefixQuant.
- MixPE: Quantization and Hardware Co-design for Efficient LLM Inference by The Chinese University of Hong, Tsinghua University, Huawei Noah’s Ark Lab (https://arxiv.org/pdf/2411.16158). The paper proposes performing dequantization after per-group mixed-precision GEMM, significantly reducing dequantization overhead. Second, instead of relying on conventional multipliers, the method utilizes efficient shift&add operations for multiplication, optimizing both computation and energy efficiency. Experimental results demonstrate that the proposed design achieves better performance and energy trade-offs.
- “GIVE ME BF16 OR GIVE ME DEATH”? ACCURACY-PERFORMANCE TRADE-OFFS IN LLM QUANTIZATION by Neural Magic, Institute of Science and Technology Austria (https://arxiv.org/pdf/2411.02355). A thorough investigation, encompassing over 500,000 individual evaluations, yields several key findings: (1) FP8 weight and activation quantization (W8A8-FP) is lossless across all model scales, (2) INT8 weight and activation quantization (W8A8-INT) incurs surprisingly low 1-3% accuracy degradation, and (3) INT4 weight-only quantization (W4A16-INT) is competitive with 8-bit integer weight and activation quantization. They find that W4A16 offers the best cost-efficiency for synchronous deployments and for asynchronous deployment on mid-tier GPUs. At the same time, W8A8 formats excel in asynchronous “continuous batching” deployment of mid- and large-size models on high-end GPUs.
- GWQ: Gradient-Aware Weight Quantization for Large Language Models by PKU, CASIA, THU, USTB, UNITN, ETHz, PolyU, UCAS (https://arxiv.org/pdf/2411.00850). The authors propose gradient-aware weight quantization that leverages gradients to localize outliers, requiring only a minimal amount of calibration data for outlier detection. It retains the weights corresponding to the top 1% outliers preferentially at FP16 precision, while the remaining non-outlier weights are stored in a low-bit format. GWQ found experimentally that utilizing the sensitive weights in the gradient localization model is more scientific than utilizing the sensitive weights in the Hessian matrix localization model. The method shows accurate results for both LLM and VLM quantization.
- SDP4Bit: Toward 4-bit Communication Quantization in Sharded Data Parallelism for LLM Training by Indiana University, ByteDance, and University of Houston (https://arxiv.org/pdf/2410.15526). The paper proposes a method that reduces the communication of weights and gradients during the training to nearly 4 bits via two techniques: quantization on weight differences, and two-level gradient smooth quantization. Furthermore, the method presents an algorithm system co-design with runtime optimization to minimize the computation overhead of compression. Authors empirically evaluate the accuracy on the pre-training of GPT models with up to 6.7 billion parameters, and the results demonstrate a negligible impact on training loss. Furthermore, speed experiments show up to 4.08× speedup in end-to-end throughput on a scale of 128 GPUs.
- Quamba: A Post-Training Quantization Recipe for Selective State Space Models by University of Texas at Austin, National Yang Ming Chiao Tung University, and Cornell University (https://arxiv.org/pdf/2410.13229). Authors propose a static 8-bit per-tensor SSM quantization method which suppresses the maximum values of the input activations to the selective SSM for finer quantization precision and quantizes the output activations in an outlier-free space with Hadamard transform. 8-bit weight-activation quantized Mamba 2.8B SSM benefits from hardware acceleration and achieves a 1.72 × lower generation latency on an Nvidia Orin Nano 8G, with only a 0.9% drop in average accuracy on zero-shot tasks. Code is released at https://github.com/enyac-group/Quamba.
- RESTRUCTURING VECTOR QUANTIZATION WITH THE ROTATION TRICK by Stanford University and Google DeepMind (https://arxiv.org/pdf/2410.06424). The paper proposes a way to propagate gradients through the vector quantization layer of VQ-VAEs. The method smoothly transforms each encoder output into its corresponding codebook vector via a rotation and rescaling linear transformation that is treated as a constant during backpropagation. As a result, the relative magnitude and angle between encoder output and codebook vector becomes encoded into the gradient as it propagates through the vector quantization layer and back to the encoder. Еhis restructuring improves reconstruction metrics, codebook utilization, and quantization error. Code is available at https://github.com/cfifty/rotation_trick.
Pruning / Sparsity
- MaskLLM: Learnable Semi-Structured Sparsity for Large Language Models by NVIDIA National University of Singapore (https://arxiv.org/pdf/2409.17481). The paper introduces several fundamental findings on applying N:M sparsity to LLM models. It explicitly models N:M patterns as a learnable distribution through Gumbel Softmax sampling. This approach facilitates end-to-end training on large-scale datasets and offers two notable advantages: 1) High-quality Masks - our method effectively scales to large datasets and learns accurate masks; 2) Transferability - the probabilistic modeling of mask distribution enables the transfer learning of sparsity across domains or tasks. The method achieves SOTA results on Wikitext and as well as shows lossless compression for many downstream language tasks. The code is available at https://github.com/NVlabs/MaskLLM.
- MRT5: DYNAMIC TOKEN MERGING FOR EFFICIENT BYTE-LEVEL LANGUAGE MODELS by Stanford University (https://arxiv.org/pdf/2410.20771). The paper introduces a more efficient variant of ByT5 that integrates a token deletion mechanism in its encoder to dynamically shorten the input sequence length. After processing through a fixed number of encoder layers, a learnt delete gate determines which tokens are to be removed and which are to be retained for subsequent layers. MrT5 effectively “merges” critical information from deleted tokens into a more compact sequence, leveraging contextual information from the remaining tokens. In continued pre-training experiments, we find that MrT5 can achieve significant gains in inference runtime with minimal effect on performance. Code is available here: https://github.com/jkallini/mrt5.
- SQFT: Low-cost Model Adaptation in Low-precision Sparse Foundation Models by Intel Labs (https://aclanthology.org/2024.findings-emnlp.749.pdf). The authors propose and end-to-end solution for low-precision sparse parameter-efficient fine-tuning of large pre-trained models, allowing for effective model adaptation in resource-constrained environments. Additionally, an innovative strategy enables the merging of sparse weights with low-rank adapters without losing sparsity and accuracy, overcoming the limitations of previous approaches. SQFT also addresses the challenge of having quantized weights and adapters with different numerical precisions, enabling merging in the desired numerical format without sacrificing accuracy. Multiple adaptation scenarios, models, and comprehensive sparsity levels demonstrate the effectiveness of SQFT. Models and code are available at https://github.com/IntelLabs/Hardware-Aware-Automated-Machine-Learning.
- Post-Training Statistical Calibration for Higher Activation Sparsity by Intel Labs (https://arxiv.org/pdf/2412.07174). The paper presents a post-training activation pruning framework that (1) generalizes sparsification by input activations of Fully-Connected layers for generic and flexible application across Transformers, and (2) features a simple Mode-Centering technique to pre-calibrate activation distributions for maximizing post-training sparsity. The results demonstrate robust Pareto efficiency compared to prior methods, translating to a 1.5x additional LLM decoding speedup against] at iso model quality. The effectiveness of the method is empirically verified across a wide range of models, including recent Transformer Decoders, MoE, Mamba2, Encoding Transformer, and pre-quantized models. The code is available at: https://github.com/IntelLabs/SCAP.
- HashAttention: Semantic Sparsity for Faster Inference by UC Berkeley and ETH Zurich (https://arxiv.org/pdf/2412.14468). The paper proposes an approach that is casting pivotal token identification as a recommendation problem. Given a query, it encodes keys and queries in Hamming space capturing the required semantic similarity using learned mapping functions. The method identifies pivotal tokens for a given query in this Hamming space using bitwise operations, and only these pivotal tokens are used for attention computation. It can reduce the number of tokens used by a factor of 1/32× for the Llama-3.1-8B model with LongBench, keeping average quality loss within 0.6 points, while using only 32 bits per token auxiliary memory. Code is planned to be released.
- BEYOND 2:4: EXPLORING V:N:M SPARSITY FOR EFFICIENT TRANSFORMER INFERENCE ON GPUS by Tsinghua University, Huawei Noah’s Ark Lab, Beijing Jiaotong University (https://arxiv.org/pdf/2410.16135). Authors propose three approaches to enhance the applicability and accuracy of V:N:M-sparse Transformers, including heuristic V and M selection, V:N:M-specific channel permutation and three-staged LoRA training techniques. Experimental results show that, with with this, the DeiT-small achieves lossless accuracy at 64:2:5 sparsity, while the DeiT-base maintains accuracy even at 64:2:8 sparsity. In addition, the fine-tuned LLama2-7B at 64:2:5 sparsity performs comparably or better than training-free 2:4 sparse alternatives on downstream tasks.
Other
- InfiniPot: Infinite Context Processing on Memory-Constrained LLMs from by Qualcomm AI Research , Qualcomm Korea YH (https://arxiv.org/pdf/2410.01518). The paper introduces a KV cache control framework designed to enable pre-trained LLMs to manage extensive sequences within fixed memory constraints efficiently, without requiring additional training. The method leverages Continual Context Distillation (CCD), an iterative process that compresses and retains essential information through novel importance metrics, maintaining critical data. This distillation process is based on the combination of CE-loss over the predicted tokens and Attention scores. Evaluations indicate that the method significantly outperforms models trained for long contexts in various NLP tasks.
- DEEP COMPRESSION AUTOENCODER FOR EFFICIENT HIGH-RESOLUTION DIFFUSION MODELS by MIT, Tsinghua University, and NVIDIA (https://arxiv.org/pdf/2410.10733). Authors highlight that existing autoencoders have demonstrated impressive results at a moderate spatial compression ratio (e.g., 8×) but fail to maintain satisfactory reconstruction accuracy for high spatial compression ratios (e.g., 64×). They address this by introducing two techniques: (1) Residual Autoencoding, where we design our models to learn residuals based on the space-to-channel transformed; (2) Decoupled High-Resolution Adaptation, a decoupled three-phase training strategy for mitigating the generalization penalty of high spatial-compression autoencoders. Authors improve the autoencoder’s spatial compression ratio up to 128 while maintaining the reconstruction quality achieving significant speedup without accuracy drop (19.1× inference speedup and 17.9× training speedup on H100 GPU). Code is available at https://github.com/mit-han-lab/efficientvit.
- EoRA: Training-free Compensation for Compressed LLM with Eigenspace Low-Rank Approximation by Nvidia (https://arxiv.org/pdf/2410.21271). The paper proposes a method that directly minimizes compression-induced errors without requiring gradient-based training small amount of calibration data. The method projects compression errors into the eigenspace of input activations, leveraging eigenvalues to effectively prioritize the reconstruction of high-importance error components. It shows good results for compressed LLaMA2/3 models on various tasks, such as language generation, commonsense reasoning, and math reasoning tasks (e.g., 31.31%/12.88% and 9.69% improvements on ARC-Easy/ARC-Challenge and MathQA when compensating LLaMA3-8B that is quantized to 4-bit and pruned to 2:4 sparsity).
- Eigen Attention: Attention in Low-Rank Space for KV Cache Compression by Purdue University (https://arxiv.org/pdf/2408.05646). Authors propose Eigen Attention, which performs the attention operation in a low-rank space, thereby reducing the KV cache memory overhead. The proposed approach is orthogonal to existing KV cache compression techniques and can be used synergistically with them. Experiments demonstrate that Eigen Attention results in up to 40% reduction in KV cache sizes and up to 60% reduction in attention operation latency with minimal drop in performance. Code is available at https://github.com/UtkarshSaxena1/EigenAttn.
- RAGCache: Efficient Knowledge Caching for Retrieval-Augmented Generation by Peking University and ByteDance (https://arxiv.org/pdf/2404.12457). Authors propose RAGCache, the system that caches the intermediate states of external knowledge and shares them across multiple queries to reduce the redundant computation. They design a prefix-aware GDSF replacement policy that leverages the characteristics of RAG to minimize the miss rate and a dynamic speculative pipelining approach to minimize the end-to-end latency. The experimental results show that RAGCache reduces the time to first token (TTFT) by up to 4× and improves the throughput by up to 2.1× compared to vLLM integrated with Faiss.
- STAR: Synthesis of Tailored Architectures by Liquid AI (https://arxiv.org/pdf/2411.17800). In this work, authors propose a NAS-based approach for the synthesis of LLM architectures. This approach combines a search space based on the theory of linear input-varying systems, supporting a hierarchical numerical encoding into architecture genomes. The genomes are automatically refined and recombined with gradient-free, evolutionary algorithms to optimize for multiple model quality and efficiency metrics. Using the method, authos optimize large populations of new architectures, leveraging diverse computational units and interconnection patterns, improving over highly-optimized Transformers and striped hybrid models on the frontier of quality, parameter size, and inference cache for autoregressive language modeling.
- SWITTI: Designing Scale-Wise Transformers for Text-to-Image Synthesis by Yandex Research, HSE University, MIPT, Skoltech, ITMO University (https://arxiv.org/pdf/2412.01819). The paper presents text-to-image transformer that employs architectural modifications to improve training stability and convergence and excludes explicit autoregression for more efficient sampling and better scalability. Compared to state-of-the-art text-to-image diffusion models, the model is up to 7× faster while demonstrating competitive performance. Additionally, the model reduces memory consumption during inference, previously needed for storing key-value (KV) cache, enabling better scaling to higher resolution image generation. The model has weaker reliance on the text at high-resolution scales. This observation allows to disable classifier-free guidance at the last two steps, resulting in further ∼20% acceleration and better generation of fine-grained details, as confirmed by human evaluation.
- SWIFTKV: FAST PREFILL-OPTIMIZED INFERENCE WITH KNOWLEDGE-PRESERVING MODEL TRANSFORMATION by Snowflake AI Research (https://arxiv.org/pdf/2410.03960). The paper presents a model transformation and distillation procedure specifically designed to reduce the time and cost of processing prompt tokens while preserving the quality of generated tokens. The method combines three key mechanisms: i) SingleInputKV, which prefills later layers’ KV cache using a much earlier layer’s output, allowing prompt tokens to skip much of the model computation, ii) AcrossKV, which merges the KV caches of neighboring layers to reduce the memory footprint and support larger batch size for higher throughput, and iii) a knowledge-preserving distillation to recover the accuracy. For Llama-3.1-8B and 70B, the method reduces the compute requirement of prefill by 50% and the memory requirement of the KV cache by 62.5% while incurring minimum quality degradation across a wide range of tasks. Optimized models are available here.
- KV PREDICTION FOR IMPROVED TIME TO FIRST TOKEN by Apple (https://arxiv.org/pdf/2410.08391). In this method, a small auxiliary model is used to process the prompt and produce an approximation of the KV cache used by a base model. This approximated KV cache is then used with the base model for autoregressive generation without the need to query the auxiliary model again. Authors demonstrate that the method produces a pareto-optimal efficiency-accuracy trade-off when compared to baselines. On TriviaQA, they demonstrate relative accuracy improvements in the range of 15%−50% across a range of TTFT FLOPs budgets. They also demonstrate accuracy improvements of up to 30% on HumanEval python code completion at fixed TTFT FLOPs budgets. We release our code here.
- MAMBAEXTEND: A TRAINING-FREE APPROACH TO IMPROVE LONG-CONTEXT EXTENSION OF MAMBA (https://openreview.net/pdf?id=LgzRo1RpLS). The paper discloses the method that aims to extend the context length of SSM models, in particular Mamba family. The method leverages a training-free approach to calibrate only the scaling factors of discretization modules for different layers. Authors demonstrate both gradient-based and gradient-free zeroth-order optimization to learn the optimal scaling factors for each Mamba layer, requiring orders of magnitude fewer updates as opposed to the parameter fine-tuning-based alternatives. The method shows good accuracy on the Pile and Longbench benchmarks.
- Exploiting LLM Quantization by ETH Zurich (https://arxiv.org/pdf/2405.18137). A method which produces a malicious LLM from an original LLM. Malicious model performs similarly while in FP32 precision but malicious after the quantization. LLM -> malicious LLM -> Repairing malicious LLM via projected gradient descent subject to quantization blocks of the malicious LLM
- DEEP COMPRESSION AUTOENCODER FOR EFFICIENT HIGH-RESOLUTION DIFFUSION MODELS by MIT, Tsinghua University, and NVIDIA (https://arxiv.org/pdf/2410.10733). The proposed method is aimed to optimize image generation autoencoders by introducing two key techniques: (1) Residual Autoencoding, where authors design models to learn residuals based on the space-to-channel transformed features to alleviate the optimization difficulty of high spatial-compression autoencoders; (2) Decoupled High-Resolution Adaptation, a decoupled three-phase training strategy for mitigating the generalization penalty of high spatial-compression autoencoders. The method improves the autoencoder’s spatial compression ratio up to 128 while maintaining the reconstruction quality. Authors achieve significant speedup without accuracy drop. For example, on ImageNet 512 × 512, the model provides 19.1× inference speedup and 17.9× training speedup on H100 GPU for UViT-H while achieving a better FID. Code is available at: https://github.com/mit-han-lab/efficientvit.
- DUOATTENTION: EFFICIENT LONG-CONTEXT LLM INFERENCE WITH RETRIEVAL AND STREAMING HEADS by MIT, Tsinghua University, SJTU, University of Edinburgh, NVIDIA (https://arxiv.org/pdf/2410.10819). In this paper, authors identify that only a fraction of attention heads, a.k.a, Retrieval Heads, are critical for processing long contexts and require full attention across all tokens. In contrast, all other heads, which primarily focus on recent tokens and attention sinks–referred to as Streaming Heads–do not require full attention. They introduce a framework that only applies a full KV cache to retrieval heads while using a light-weight, constant-length KV cache for streaming heads, which reduces both LLM’s decoding and pre-filling memory and latency. DuoAttention uses a lightweight, optimization-based algorithm with synthetic data to identify retrieval heads accurately. The method reduces long-context inference memory by up to 2.55× for MHA and 1.67× for GQA models while speeding up decoding by up to 2.18× and 1.50× and accelerating pre-filling by up to 1.73× and 1.63× for MHA and GQA models, respectively. Code is available at: https://github.com/mit-han-lab/duo-attention.
Software
- KV-COMPRESS: PAGED KV-CACHE COMPRESSION WITH VARIABLE COMPRESSION RATES PER ATTENTION HEAD by Cloudflare (https://arxiv.org/pdf/2410.00161). KV-Compress introduces a method to reduce the KV cache memory footprint by selectively compressing attention heads based on their importance. While early approaches measure KV importance by aggregating attention across all past queries, recent works show performance improvements by focusing on the final prompt tokens within a limited observation window. KV-Compress evicts contiguous KV blocks within a PagedAttention framework, reducing the memory footprint proportionally to the theoretical compression rate. Extending Ada-SnapKV, it supports per-layer and per-head variable compression rates, achieving state-of-the-art results on the LongBench suite. The "query-group-compression" technique further compresses the KV cache of GQA models without expanding it into the dimension of total query heads, achieving up to a 4x additional reduction. Integrated within vLLM, KV-Compress demonstrates the first end-to-end benchmarks of an eviction-based KV cache compression method within a paged-attention-enabled framework for efficient LLM inference. Code is available at https://github.com/IsaacRe/vllm-kvcompress.
- Introducing Machete, a Mixed-Input GEMM Kernel Optimized for NVIDIA Hopper GPUs.
- AMD released TensorCast, a casting/quantization PyTorch-based library to emulate various precisions: https://github.com/ROCm/tensorcast.
- MInference: Million-Tokens Prompt Inference for Long-context LLMs. A research project that is driven by Microsoft for a long-context text generation tasks. It contains implementation of several state-of-the-art methods.
Q2'24: Technology Update – Low Precision and Model Optimization
Authors
Alexander Kozlov, Nikita Savelyev, Vui Seng Chua, Souvikk Kundu, Nikolay Lyalyushkin, Andrey Anufriev, Pablo Munoz, Alexander Suslov, Liubov Talamanova, Yury Gorbachev, Nilesh Jain, Maxim Proshin
Summary
This quarter we see an increasing interest in KV-cache optimization of Large Language and Vision Models. This actually expected as KV-cache is getting a bottleneck after the weight compression problem is solved to some degree. We also believe that KV-cache optimization will continue being a hot topic as it is also involved in the Video Generations scenario where we see a lot of work going on nowadays.
Highlights
- QServe: W4A8KV4 Quantization and System Co-design for Efficient LLM Serving by MIT, NVIDIA, UMass Amherst, MIT-IBM Watson AI Lab (https://arxiv.org/pdf/2405.04532). A regular work from Song Han Lab which is a comprehensive study of deep LLM optimization and a reference design of a tool for LLM serving. The LLM optimization part includes: W4A8 and 4-bit KV-cache quantization approach; Progressive quantization of weights, to comply with 8-bit compute after dequantizing4-bit weights to 8-bits; SmoothAttention method, to reduce the error of 4-bit quantization of Key cache that is compatible with RoPE operation and can be fused into a preceding Linear layer; Progressive quantization of weights, to comply with 8-bit compute after dequantizing4-bit weights to 8-bits. The inference part contains tips and tricks to design efficient inference kernels and execution pipelines on the Nvidia GPUs. The method shows superior results comparing to competitive solutions and demonstrates the ability to substantially reduce LLM serving costs. Some code and pre-compiled binaries are available here: https://github.com/mit-han-lab/qserve.
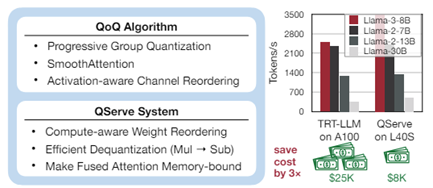
- ZipCache: Accurate and Efficient KV Cache Quantization with Salient Token Identification by Houmo AI and Chinese universities (https://arxiv.org/pdf/2405.14256).Authors present a KV cache quantization method for LLMs. First, they construct a strong baseline for quantizing KV cache. Through the proposed channel-separable token-wise quantization scheme, the memory overhead of quantization parameters is substantially reduced compared to fine-grained group-wise quantization. To enhance the compression ratio, they propose a normalized attention score. The quantization bit-width for each token is adaptively assigned based on their saliency. The authors also develop an approximation method that decouples the saliency metric from full attention scores compatible with FlashAttention. Experiments demonstrate that the method achieves good compression ratios at fast generation speed, for example, when evaluating Mistral-7B model on GSM8k dataset, the method is capable of compressing the KV cache by 4.98×,with only a 0.38% drop in accuracy.
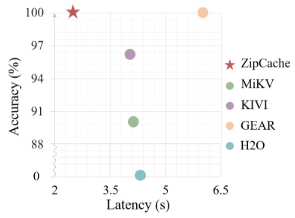
- BitsFusion: 1.99 bits Weight Quantization of Diffusion Model by Snap Inc. and Rutgers University (https://arxiv.org/pdf/2406.04333). The paper provides a thorough analysis of UNet weight-only quantization of Stable Diffusion 1.5 model. The authors propose an approach for mixed-precision quantization of diffusers. They quantize different layers into different bits according to their quantization error. The authors also introduce several techniques to initialize the quantized model to improve performance, including time embedding pre-computing and caching, adding balance integer, and alternating optimization for scaling factor initialization. Finally, they propose a two-stage Quantization-aware training where distillation is used at the first stage. The quantized model achieves very good results on various benchmarks. Code will be released here: https://github.com/snap-research/BitsFusion.

- Applying t-Distributions to Explore Accurate and Efficient Format[KA1] s for LLMs by Cornell University and Google (https://arxiv.org/abs/2405.03103). The paper investigates non-uniform quantization data formats by profiling the distributions of weight and activation across 30 models, including both LLM and non-LLM models. The authors discovered that Student’s t-Distribution is a better fit than the Gaussian distribution due to its flexible parameterization, which can resemble Gaussian, Cauchy, or other distributions observed indifferent neural networks. The authors derived Student Float (SF4) using a similar design process to Normal Float (NF4). SF4 outperforms NF4, FP4, and Int4 in accuracy retention across most cases and model architectures, making it a strong drop-in replacement for lookup-based datatypes like NF4. The paper proposes using SF4as a reference to extend supernormal support for existing datatypes like E2M1(one variant of FP4) and APoT4,by reassigning negative zero to a useful value, which is otherwise wasted. Additionally, the paper examines the Pareto frontier of datatypes in terms of model accuracy and MAC chip area, concluding that APoT4 and its supernormal extension are Pareto optimal for a set of models smaller than 7B parameters.
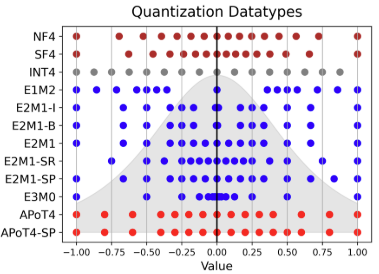
- ShiftAddLLM: MatMul-free LLM via Inference Time Reparameterization by Intel, Google Deep Mind, Google, Georgia Tech (https://arxiv.org/pdf/2406.05981).Authors developed an inference time reparameterization for traditional LLMs layers with MatMul ops to convert them to layers with Shift-Add and LUT query-based operations only. Specifically, authors quantize each weight matrix into binary matrices paired with group-wise scaling factors. The associated multiplications are reparameterized into (1) shifts between activations and scaling factors and (2) queries and adds according to the binary matrices. To reduce accuracy loss, they present a multi-objective optimization method to minimize both weight and output activation reparameterization errors. Additionally, based on varying sensitivity across layers to reparameterization, they develop an automated bit allocation strategy to further reduce memory usage and latency. The code is available at: https://github.com/GATECH-EIC/ShiftAddLLM.
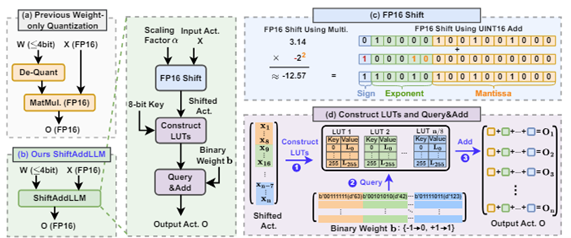
Quantization
- QServe: W4A8KV4 Quantization and System Co-design for Efficient LLM Serving by MIT, NVIDIA, UMass Amherst, MIT-IBM Watson AI Lab (https://arxiv.org/pdf/2405.04532). A regular work from Song Han Lab which is a comprehensive study of deep LLM optimization and a reference design of a tool for LLM serving. The LLM optimization part includes: W4A8 and 4-bit KV-cache quantization approach; Progressive quantization of weights, to comply with 8-bit compute after dequantizing4-bit weights to 8-bits; SmoothAttention method, to reduce the error of 4-bit quantization of Key cache that is compatible with RoPE operation and can be fused into a preceding Linear layer; Progressive quantization of weights, to comply with 8-bit compute after dequantizing4-bit weights to 8-bits. The inference part contains tips and tricks to design efficient inference kernels and execution pipelines on the Nvidia GPUs. The method shows superior results comparing to competitive solutions and demonstrates the ability to substantially reduce LLM serving costs. Some code and pre-compiled binaries are available here: https://github.com/mit-han-lab/qserve.
- LQER: Low-Rank Quantization Error Reconstruction for LLMs by Imperial College London London and University of Cambridge (https://arxiv.org/pdf/2402.02446). The paper combines quantization and low-rank approximation techniques to achieve accurate and efficient LLM optimization. The method employs MXINT4 datatype (int4 + shared exponent for 4 elements) for weight quantization while quantizing activation into 8 or 6 bits with per-token scaling factors. The method also introduces 8-bit LoRA adapters to restore accuracy after weight quantization. It does not use any kind of fine-tuning. Instead, it introduces the error decomposition into two low-rank matrices. The method achieves very accurate results in W4A8 and W4A6 settings, especially on Llama-2 model family.
- LLM-QBench: A Benchmark Towards the Best Practice for Post-training Quantization of Large Language Models by Beihang University, SenseTime Research, and Nanyang Technological University (https://arxiv.org/pdf/2405.06001).The paper focuses on identifying the most effective practices for quantizing LLMs, with the goal of balancing performance with computational efficiency. Fora fair analysis, the authors develop a quantization toolkit LLMC and design four crucial principles considering the inference efficiency, quantized accuracy, calibration cost, and modularization. By benchmarking on various models and datasets with over 500 experiments, three takeaways corresponding to calibration data, quantization algorithm, and quantization schemes are derived. Finally, a best practice of LLM PTQ pipeline is constructed. All the benchmark results and the toolkit can be found at https://github.com/ModelTC/llmc.
- SKVQ: Sliding-window Key and Value Cache Quantization for Large Language Models Houmo AI by Houmo AI and Chinese universities (https://arxiv.org/pdf/2405.06219). The paper addresses the problem of extremely low bit-width KV cache quantization. To achieve this, it proposes a method that rearranges the channels of the KV cache in order to improve the similarity of channels in quantization groups and applies clipped dynamic quantization at the group level. Additionally, the method ensures that the most recent window tokens in the KV cache are preserved with high precision. This helps maintain the accuracy of a small but important portion of the KV cache. Evaluation on LLMs demonstrates that the method surpasses previous quantization approaches, allowing for quantization of the KV cache to 2-bit keys and 1.5-bit values with minimal loss of accuracy. The code is available at https://github.com/cat538/SKVQ.
- Integer Scale: A Free Lunch for Faster Fine-grained Quantization of LLMs by Meituan (https://arxiv.org/pdf/2405.14597). The paper proposes a scheme to use integer scales when computing dot products of W4A8quantized LLMs. It allows keeping group scales for weights in the integer precision as well and using INT32 buffer as the accumulator of partial dot products. An additional floating point scale is required and applied to the super-group of dot products between weights and activations. It brings the proposed method close to the known double quantization approach. The paper provides extensive evaluation data for Llama2 and Llama3 models showing close results to the baseline floating-point scales.
- Mitigating Quantization Errors Due to Activation Spikes in GLU-Based LLMs by Hanyang University (https://arxiv.org/pdf/2405.14428).The paper aims at reducing the accuracy degradation of fully-quantized LLM models (both weights and activations are quantized). Authors propose two empirical methods, Quantization-free Module (QFeM) and Quantization-free Prefix (QFeP), to isolate the activation spikes during quantization that cause most of the accuracy drop. Essentially, they propose a way to identify what layers are more error-prone and keep these layers in the floating-point precision. The code is available at https://github.com/onnoo/activation-spikes.
- AdpQ: A Zero-shot Calibration Free Adaptive Post Training Quantization Method for LLMs by Huawei Noah Lab and McGill University (https://arxiv.org/pdf/2405.13358).This paper presents a novel zero-shot adaptive PTQ method for LLMs that does not require any calibration data. Inspired by Adaptive LASSO regression model, the authors proposed approach that tackles the challenge of outlier activations by separating salient weights using an adaptive soft-thresholding method. Guided by Adaptive LASSO, this method ensures that the quantized weights distribution closely follows the originally trained weights and eliminates the need for calibration data entirely. The method achieves good results at much faster quantization time.
- PTQ4SAM: Post-Training Quantization for Segment Anything by Beihang University (https://arxiv.org/pdf/2405.03144).A practical study on quantization of the Segment Anything model. The authors observe a challenging bimodal distribution for quantization and analyze its characteristics. To overcome it, they propose a Bimodal Integration (BIG)strategy, which automatically detects it and transforms the bimodal distribution to normal distribution equivalently. They also present the Adaptive Granularity Quantization which represents diverse post-Softmax distributions accurately with appropriate granularity. Experiments show that the method can achieve good results even in low-bit quantization settings (6 or4 bits). Code is available at https://github.com/chengtao-lv/PTQ4SAM.
- QNCD: Quantization Noise Correction for Diffusion Models by Kuaishou Technology (https://arxiv.org/pdf/2403.20137). Authors identify two primary quantization challenges for Duffusion models: intra and inter quantization noise. Intra quantization noise, exacerbated by embeddings in the resblock module, extends activation quantization ranges, increasing disturbances in each single denoising step. Besides, inter quantization noise stems from cumulative quantization deviations across the entire denoising process, altering data distributions step-by-step. Authors propose embedding-derived feature smoothing for eliminating intra quantization noise and a runtime noise estimation module for dynamically filtering inter quantization noise. Experiments demonstrate that the method achieves good results in W4A8 and W8A8 quantization settings on ImageNet (LDM-4). Code is available at: https://github.com/huanpengchu/QNCD.
- SliM-LLM: Salience-DrivenMixed-Precision Quantization for Large Language Models by The ETH Zürich, University of Hong Kong, and Beihang University (https://arxiv.org/pdf/2405.14917).The paper focuses on the problem of ultra-low bit weight quantization of LLMs. Specifically, it proposes the method relies on two novel techniques: (1)Salience-Determined Bit Allocation utilizes the clustering characteristics of salience distribution to allocate the bit-widths of each quantization group. This increases the accuracy of quantized LLMs and maintains the inference efficiency high; (2) Salience-Weighted Quantizer Calibration optimizes the parameters of the quantizer by considering the element-wise salience within the group. The method is evaluated in two setups for quantization parameters tuning: greedy search and gradient based search. Evaluation shows good results on Llama 1/2/3 models. Code is available at https://github.com/Aaronhuang-778/SliM-LLM.
- LCQ: Low-Rank Codebook based Quantization for Large Language Models by Nanjing University (https://arxiv.org/pdf/2405.20973). The paper proposes a method for LLM optimization using customized low-ranking codebooks the rank of which can be larger than one, for quantization. A gradient-based optimization algorithm is proposed to optimize the parameters of the codebook. The method also adopts a double quantization strategy for compressing the parameters of the codebook, which can reduce the storage cost of the codebook. Experiments show that achieves better accuracy than existing methods with a negligibly extra storage cost.
- P2 -ViT: Power-of-Two Post-Training Quantization and Acceleration for Fully Quantized Vision Transformer by Nanjing University and Sun Yat-sen University (https://arxiv.org/pdf/2405.19915). The paper introduces a Power-of-Two (PoT) post-training quantization and acceleration framework for ViT models. The authors analyze ViTs’ properties and develop a dedicated quantization scheme. This scheme incorporates techniques such as adaptive PoT rounding and PoT Aware smoothing, allowing for the efficient quantization of ViTs with PoT scaling factors. By doing this, computationally expensive floating-point multiplications and divisions with in the re-quantization process can be traded with hardware-efficient bitwise shift operations. Furthermore, we introduce a coarse-to-fine automatic mixed-precision quantization methodology for better accuracy-efficiency trade-offs. Finally, authors build a dedicated accelerator engine to better everage our algorithmic properties for enhancing hardware efficiency. Code is available at: https://github.com/shihuihong214/P2-ViT.
- QJL: 1-Bit Quantized JLTransform for KV Cache Quantization with Zero Overhead by New York University and Adobe Research (https://arxiv.org/pdf/2406.03482).The paper studies problems of KV-cache quantization of LLMs, specifically the Key part as it is more error-prone when lowering its precision. Authors propose an approach that consists of a Johnson-Lindenstrauss (JL) transform followed by sign-bit quantization for Key cache. They introduce an asymmetric estimator for the inner product of two vectors and demonstrate that applying the method to one vector and a standard JL transform without quantization to the other provides an unbiased estimator with minimal distortion. They also developed a CUDA-based implementation for optimized computation. When applied across various LLMs and NLP tasks to quantize the KV cache to only 3 bits, the method demonstrates a more than fivefold reduction in KV cache memory usage without an insignificant accuracy drop. Codes will be available at https://github.com/amirzandieh/QJL.
- ViDiT-Q: Efficient and Accurate Quantization of Diffusion Transformers for Image and Video Generation by Tsinghua University, Infinigence AI, 3Microsoft, and Shanghai Jiao Tong University (https://arxiv.org/pdf/2406.02540).The paper tackles the problems of accurate quantization of diffusion vision transformer models. Essentially, authors apply dynamic 8-bit per-token quantization to activations. They also propose to smooth activation with a Smoothquant-like approach but with different α factors tuned to each iteration of the diffusion process. Finally, authors propose to select a per-layer weight bit-width (e.g.W4A8, W6A6, or W8A8) depending on the sensitivity and position of the layer in the Transformer block. All these tricks lead to very good accuracy results in the image and video generation tasks.
- Instance-Aware Group Quantization for Vision Transformers by Yonsei University and Articron (https://arxiv.org/pdf/2404.00928). In this paper an approach for instance-aware group quantization for ViTs(IGQ-ViT) is introduced. According to the approach, channels of activation maps are dynamically split into multiple groups where each group has its own set of quantization parameters. Authors also extend their scheme to quantize softmax attentions across tokens. IGQ-ViT demonstrates superior accuracy results across image classification, object detection and instance segmentation task. Authors claim that performance overhead induced by dynamic quantization is no more than 4% compared to layer-wise quantization.
- Reg-PTQ: Regression-specialized Post-training Quantization for Fully Quantized Object Detector by Beihang University (https://openaccess.thecvf.com/content/CVPR2024/papers/Ding_Reg-PTQ_Regression-specialized_Post-training_Quantization_for_Fully_Quantized_Object_Detector_CVPR_2024_paper.pdf). In this paper authors explore full quantization of object detection models contrary to most existing approaches which quantize only detection backbones and keep detection head in original precision. Based on the findings, the reason behind poor quantization of detector heads is that they are optimized to solve regression tasks. Specifically, authors argue that (1) regressors are more sensitive to perturbation compared to classifiers, (2) minimizing quantization error does not necessarily result in optimal scaling factors for regressor and(3) regressors weights follow non-uniform distribution contrary to classifiers. To tackle these problems a novel Reg-PTQ method is introduced. Based on the results it achieves 7.6x and 5.4x reduction in computation and storage consumption under INT4 precision with little performance degradation.
- Towards Accurate Post-training Quantization for Diffusion Models (https://openaccess.thecvf.com/content/CVPR2024/papers/Wang_Towards_Accurate_Post-training_Quantization_for_Diffusion_Models_CVPR_2024_paper.pdf). In this paper authors propose a method for accurate post-training quantization of diffusion models. The main idea is to split diffusion timesteps for each layer into groups where each group corresponds to its own set of quantization parameters. Such split is obtained by minimizing some optimization objective on a calibration dataset. Besides this, a special timestep selection method is employed for sampling timesteps for calibration. Overall, the method demonstrates superior generation quality results over such baselines as LSQ, PTQ4DM and Q-Diffusion.
Pruning/Sparsity
- Effective Interplay between Sparsity and Quantization: From Theory to Practice by Google and EcoCloud (https://arxiv.org/pdf/2405.20935). Authors provide the theoretical analysis of how sparsity and quantization interact. Mathematical proofs establish that applying sparsity before quantization (S → Q) is the optimal sequence for compression. Authors demonstrate that sparsity and quantization are not orthogonal operations. Combining them introduces additional errors beyond the sum of their individual errors. They validate theoretical findings through experiments covering a diverse range of models, including prominent LLMs (OPT, LLaMA) and ViTs. The code will be published at: https://github.com/parsa-epfl/quantization-sparsity-interplay.
- Prompt-prompted Mixture of Experts for Efficient LLM Generation by CMU (https://arxiv.org/pdf/2404.01365). Authors introduce GRIFFIN, a training-free MoE that selects unique FF experts at the sequence level for efficient generation across a plethora of LLMs with different non-ReLU activation functions. This is possible due to a critical observation that many trained LLMs naturally produce highly structured FF activation patterns within a sequence, which we call flocking. Despite the method’s simplicity, it shows with 50% of the FF parameters, GRIFFIN maintains the original model’s performance with little to no degradation on a variety of classification and generation tasks, all while improving latency (e.g. 1.25× speed-up in Llama 213B on an NVIDIA L40). Code is available at https://github.com/hdong920/GRIFFIN.
- Sparse maximal update parameterization: A holistic approach to sparse training dynamics by Cerebras Systems (https://arxiv.org/pdf/2405.15743).This paper addresses the common issue in sparse training where hyper parameters from dense training are reused, leading to suboptimal convergence, and requiring extensive tuning for different sparsity ratios. The researchers introduce a novel sparse training methodology called Sparse Maximal Update Parameterization (SuPar), which extends the maximal update parameterization (uP)to sparse training. SuPar involves reparameterizing (see Table 1) weight initialization and learning rates relative to changes in sparsity, effectively preventing exploding or vanishing signals and maintaining stable activation, gradient, and weight update scales across varying sparsity levels and model widths. SuPar reparameterization is remarkable, it allows zero-shot hyperparameter transfer, i.e. practitioners can now tune small proxy models(dense/sparse) and transfer optimal HPs directly to models at scale for any model sparsity, thus enhancing the efficiency and reducing the cost of sparse model development. Experiments demonstrate that SμPar sets the Pareto frontier best loss across all sparsities and widths, including large dense model with width equal to GPT-3 XL.
- Sparse Expansion and Neuronal Disentanglement by MIT, IST Austria, Neural Magic (https://arxiv.org/pdf/2405.15756). Sparse Expansion is an approach of converting dense LLMs to mixture of sparse experts to attain inference efficiency. The method begins with applying dimensionality reduction (PCA) on the inputs of FFN linear layers, followed by a k-means clustering. The intuition is that tokens within a cluster share a sparse expert better without significant distortion. SparseGPT is then used to create a sparse expert for each cluster group. During inference, the PCA and k-means models act as routers, directing tokens to the appropriate sparse expert based on their cluster. While this increases the overall model size, acceleration is achieved through the conditional execution of experts and the sparse execution of these experts, with minimal cost for the routers. The paper includes layer-wise speedup benchmarks and shows that Sparse Expansion outperforms other one-shot sparsification approaches in perplexity for the same inference FLOP budget per token. A significant portion of the paper is dedicated to the concept of neuron entanglement, explaining, and quantifying the efficacy of sparse expansion.
- MULTIFLOW: Shifting Towards Task-Agnostic Vision-Language Pruning by University of Trento and Cisco Research (https://arxiv.org/pdf/2404.05621). Authors highlight that existing techniques for pruning of Visual-Language models(VLMs) are task-specific and propose a task-agnostic method for pruning VLMs. The proposed Multimodal Flow Pruning framework has the following properties: (1) the importance of a weight is computed based on saliency of the neurons it connects; and (2) parameters are pruned considering features of which modality they are used to compute allowing to avoid pruning too much from a single modality and too little from another. Experiments show that the proposed MULTIFLOW method outperforms recent more sophisticated competitors.
Other methods
- Flash Diffusion: Accelerating Any Conditional Diffusion Model for Few Steps Image Generation by Jasper Research (https://arxiv.org/pdf/2406.02347). The paper proposes a LoRA-compatible distillation method aiming at reducing the number of sampling steps required to generate high-quality samples from a trained diffusion model. Authors emphasize the versatility of the method through an extensive experimental study across various tasks (text-to-image, image inpainting, super-resolution, face-swapping), diffusion model architectures (SD1.5, SDXL and Pixart-α) and illustrate its compatibility with adapters. The method is relatively lightweight and can optimize SD1.5 model with 2 Nvidia H100 80GB with 13 hours of fine-tuning. Code is available at https://github.com/gojasper/flash-diffusion.
- GaLore: Memory-Efficient LLM Training by Gradient Low-Rank Projection by California Institute of Technology, Meta AI, University of Texas at Austin, and Carnegie Mellon University (https://arxiv.org/pdf/2403.03507). The paper introduces a Gradient Low-Rank Projection (GaLore), a training strategy that allows full-parameter learning but is more memory-efficient than common low-rank adaptation methods such as LoRA. The idea is to use PCA after a number of training steps to obtain a gradient projection matrix and use it to get a low-rank gradient matrix that is used for weights update. The approach reduces memory usage by up to 65.5% in optimizer states while maintaining both efficiency and performance for pre-training. 8-bit GaLore further reduces optimizer memory by up to 82.5% and total training memory by 63.3%, compared to a BF16 baseline. It demonstrates the feasibility of pre-training a 7B model on consumer GPUs with 24GB memory. The code is available at: https://github.com/jiaweizzhao/GaLore.
- MiniCache: KV Cache Compression in Depth Dimension for Large Language Models by ZIP Lab of Monash and Zhejiang University (https://arxiv.org/pdf/2405.14366).The authors propose a training-free KV cache compression technique by merging KV tokens across every two consecutive transformer layers, based on the observation that KV tokens are highly similar across depth, especially from the middle to the last transformer layers. Specifically, a pair of K/V projections from two consecutive layers can be encoded into respective scaling factors and a shared directional vector computed via Spherical Linear Interpolation(SLERP). To address the information loss from merging dissimilar tokens, the algorithm uses angular-based distance to filter KV positions for retention. The algorithm is straightforward, involving calibration of only two hyperparameters, and it has demonstrated to enhance a 4X compressed KV cache by4-bit quantization to over 5X compression while retaining reasonable accuracy of instruction-tuned Mistral, LLama2-7B across benchmarks.
- Scalable MatMul-free Language Modeling by University of California, Soochow University, LuxiTech (https://arxiv.org/pdf/2406.02528). Authors develop a MatMul-free language model by using additive operations in dense layers and element-wise Hadamard products for self-attention-like functions. Specifically, ternary weights eliminate MatMul in dense layers, similar to BNNs. To remove MatMul from self-attention, they optimize the Gated Recurrent to rely solely on element-wise products and show that this model competes with state-of-the-art Transformers while eliminating all MatMul operations. To quantify the hardware benefits of lightweight models, the authors provide an optimized GPU implementation in addition to a custom FPGA accelerator. By using fused kernels in the GPU implementation of the ternary dense layers, training is accelerated by 25.6% and memory consumption is reduced by up to 61.0% over an unoptimized baseline on GPU. Furthermore, by employing lower-bit optimized CUDA kernels, inference speed is increased by 4.57 times, and memory usage is reduced by a factor of 10 when the model is scaled up to 13B parameters. The code is available at https://github.com/ridgerchu/matmulfreellm.
- Unlocking Efficiency in Large Language Model Inference: A Comprehensive Survey of Speculative Decoding by Hong Kong Polytechnic, Peking University, Microsoft Research Asia and Alibaba (https://arxiv.org/abs/2401.07851). While LLMs are proliferating over the past two years, Speculative Decoding (SD) has emerged as a crucial paradigm to accelerate autoregressive generation. This survey is among the first to provide a comprehensive introduction and overview of the state of the art in SD, highlighting key developments in this space. A main contribution of this work is the introduction of Spec-Bench, a unified benchmark for evaluating SD methods across standardized subtasks such as multi-turn conversation, summarization, RAG, translation, question answering, and mathematical reasoning. The codes and benchmarks for various SD methods on RTX 3090 and A100 GPUs are accessible for further exploration and validation.
- Speculative Decoding via Early-exiting for Faster LLM Inference with Thompson Sampling Control Mechanism by Meituan and Meta AI (https://arxiv.org/pdf/2406.03853).The paper introduces an early-exiting framework for generating draft tokens, which allows a single LLM to fulfill the drafting and verification stages. The model is trained using self-distillation. The authors conceptualize the generation length of draft tokens as a multi-armed bandit problem and propose a control mechanism based on Thompson Sampling, which leverages sampling to devise an optimal strategy. They conducted experiments on three benchmarks and showed that the method can significantly improve the model’s inference speed.
- LayerSkip: Enabling Early Exit Inference and Self-Speculative Decoding by Meta, University of Toronto, Carnegie Mellon University, University of Wisconsin-Madison, Dana-Farber Cancer Institute (https://arxiv.org/pdf/2404.16710).Authors research the idea of early exit in LLMs for speculative decoding. First, during training, they apply layer dropout, with low dropout rates for earlier layers and higher dropout rates for later layers, and an early exit loss where all transformer layers share the same exit. Second, during inference, they show that this training recipe increases the accuracy of early exit at earlier layers, without adding any auxiliary layers or modules to the model. Third, they present a self-speculative decoding solution where we exit at early layers and verify and correct with remaining layers of the model. They run experiments on different Llama model sizes on different types of training: pretraining from scratch, continual pretraining, finetuning on specific data domain, and finetuning on specific task, and show speedups of up to 2.16× on summarization for CNN/DM documents, 1.82× on coding, and 2.0× on TOPv2 semantic parsing task.
Software
- INT4 Decoding GQA CUDA Optimizations for LLM Inference by Meta(https://pytorch.org/blog/int4-decoding).The authors provide a comprehensive study and ten practical steps, including KV-cache quantization, to improve the performance of Grouped-query Attention. All these optimizations result in performance improvements of up to 1.8x on the NVIDIA A100 GPU and 1.9x on the NVIDIA H100 GPU.
- torchao: PyTorch Architecture Optimization by Meta (https://github.com/pytorch/ao). PyTorch library for quantization and sparsity. Currently-available features contain full models quantization, INT8, INT4, MXFP4,6,8 weight-only quantization and efficient model fine-tuning with GaLore method.
- Introducing Apple’s On-Device and Server Foundation Models by Apple (https://machinelearning.apple.com/research/introducing-apple-foundation-models). Apple has established a set of pre-trained and optimized models for its HW. The claim is that 3B LLM model can be run at 30t/s on iPhone 15 Pro. In terms of optimizations that are being used, authors claim weight palletization to 2 and 4 bits, quantization of embeddings and activations and efficient Key-Value (KV) cache update. They use their own AXLearn library built on top of JAX and XLA for model pre-training and fine-tuning.
- BitBLAS by Microsoft (https://github.com/microsoft/BitBLAS).A library to support mixed-precision BLAS operations on GPUs. BitBLAS aims to support efficient mixed-precision DNN model deployment, especially the quantization in large language models (LLMs), for example, the 𝑊4𝐴16 in GPTQ, the 𝑊2𝐴16 in BitDistiller, the 𝑊2𝐴8 in BitNet-b1.58.
Q2'23: Technology update – low precision and model optimization
Authors
Alexander Kozlov, Nikita Savelyev, Nikolay Lyalyushkin, Vui Seng Chua, Pablo Munoz, Alexander Suslov, Andrey Anufriev, Liubov Talamanova, Yury Gorbachev, Nilesh Jain
Summary
This quarter we observed tremendous interest and breakthroughs in the Large Language Models optimization. Most research is basically focusing on low-bit weights quantization (INT4/INT3) which leads to a substantial reduction in model footprint and significant inference performance improvement in the case if corresponding HW kernels are available. There is also increased interest in low-bit floating-point data types such as FP8 and NF4. In addition, we reviewed relevant papers published on the recent CVPR conference and put them into a separate subsections for your convenience.
Highlights
- FP8 versus INT8 for efficient deep learning inference by Qualcomm AI Research (https://arxiv.org/pdf/2303.17951.pdf).A comprehensive study and comparison between FP8 and INT8 precisions for inference. Authors consider various modifications of FP8 data type and they fit inference of different DL models including Transformer and Convolutional networks. They also consider post-training and quantization-aware training settings and how models are mapped to the inference of the data types under consideration in terms of accurate results. The paper also contains an analysis of the HW efficiency of FP8 and INT8. The main conclusion of this paper is thatFP8 types do not provide an optimal solution for low-precision inference compared to INT8 types, especially, in edge scenarios. All the existing problems of INT8 inference can be worked around with mixed integer precisionINT4-INT8-INT16.
- Outlier Suppression+:Accurate quantization of large language models by equivalent and optimal shifting and scaling by SenseTime and universities of China and US (https://arxiv.org/pdf/2304.09145.pdf).The method represents a continuation of the idea described in Smooth Quant method. Besides the per-channel scaling of activations, authors also adopt the shift operation. They show how these additional operations can be incorporated into the optimizing model in a way that does not hurt performance after quantization. Experiments show that applying the method to various Language models including large OPT-family models allows quantizing them accurately even to the precisions lower than 8-bit, e.g. 6 or 4 bits.
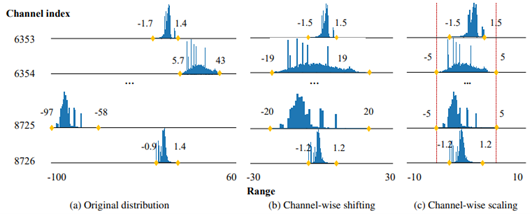
- AWQ: Activation-aware Weight Quantization for LLM Compression and Acceleration by Song Han Lab (https://arxiv.org/pdf/2306.00978.pdf).Authors propose a weights quantization method for Large Language models that is able to achieve 4, 3 and 2 bit compression with a moderate accuracy degradation. In the paper, they claim the importance of the small portion weights (salient weights) which is usually 0.1-1%. The method focuses on the accurate representation of these salient weights by searching for a quantization scaling factor. It also aligns all the weights using a trick similar to the Smooth Quant method but with a focus on weights unification within each channel. The authors also conduct a comparison with the latest GPTQ method and provide a way how to combine these two methods to achieve ultra-low-bit weight compression (2 bits). The method shows significant improvement in the inference speed (1.45x) compared to vanilla GPTQ. Code is available at: https://github.com/mit-han-lab/llm-awq
- QLORA: Efficient Finetuning of Quantized LLMs by University of Washington (https://arxiv.org/pdf/2305.14314.pdf).The paper proposes an effective way to reduce memory footprint during the Large Language Models fine-tuning by quantizing most of the weights to 4 bits. A new NormalFloat 4-bit (NF4) data type, that is information-theoretically optimal for normally distributed weights, is introduced for this purpose. Authors also use the so-called double quantization to compress weight quantization parameters and further reduce the model footprint. Most of the workflow is similar to LoRA method. The authors also provide CUDA kernels for fast training. The method is used to tune various LLMs and shows good results(sometimes even better than baselines). It is already integrated with Hugging Face: https://huggingface.co/blog/4bit-transformers-bitsandbytes.
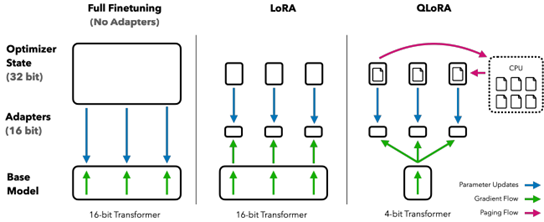
- PDP: Parameter-free Differentiable Pruning is All You Need by Apple Inc. (https://arxiv.org/pdf/2305.11203.pdf).The paper introduces a very simple and compelling idea on how to compute differentiable threshold to obtain the pruning mask based on the desired pruning ratio. The method allows to do it on-fly in a more efficient and stable way for the training so that the decision about which way to prune and which not is being taken during almost the whole training process. The method can be applied in a structured an unstructured way and achieves superior results on both Conv and Transformer-based models.
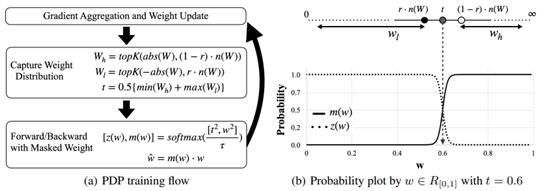
Papers with notable results
Quantization
- Memory-Efficient Fine-Tuning of Compressed Large Language Models via sub-4-bit Integer Quantization by NAVER Cloud, University of Richmond, SNU AI Center, KAIST AI (https://arxiv.org/pdf/2305.14152.pdf).The paper presents Parameter-Efficient and Quantization-aware Adaptation(PEQA), a quantization-aware PEFT technique that facilitates model compression and accelerates inference. PEQA operates through a dual-stage process: initially, the parameter matrix of each fully-connected layer undergoes quantization into a matrix of low-bit integers and a scalar vector subsequently, fine-tuning occurs on the scalar vector for each downstream task. This compresses the size of the model considerably, leading to a lower inference latency upon deployment and a reduction in the overall memory required. The method demonstrates scalability, task-specific adaptation performance for several well-known models, including LLaMA and GPT-Neo and -J.
- Integer or Floating Point? New Outlooks for Low-Bit Quantization on Large Language Models by Microsoft and universities of China (https://arxiv.org/pdf/2305.12356.pdf).Authors analyze the effectiveness and applicability of INT8/INT4 and FP8/FP4precision to quantization of Large Language Models. They conclude that there is no winner for both weight-only and weights-activations quantization settings. Thus they propose a relatively simple method that selects the optimal per-layer precision for LLM quantization. They provide an extensive comparison on different LLaMA models and compare results with the recent GPTQ (for weight-only) and vanilla FP8/INT8 (for weights-activations) quantization. The proposed method surpasses or outperforms baselines.
- PTQD: Accurate Post-Training Quantization for Diffusion Models by Zhejiang University and Monash University (https://arxiv.org/pdf/2305.10657.pdf). The paper is a post-training quantization framework for diffusion models and unifies a formulation for quantization noise and diffusion perturbed noise. The authors disentangle the quantization noise into correlated and uncorrelated parts regarding its full-precision counterpart. They propose how to correct the correlated part by estimating the correlation coefficient and propose Variance Schedule Calibration to rectify the residual uncorrelated part. The authors also introduce a Step-aware Mixed Precision scheme, which dynamically selects the appropriate bit-widths for synonymous steps, guaranteeing adequate SNR throughout the denoising process. Experiments demonstrate that the method reaches a good performance for mixed-precision post-training quantization of diffusion models on certain tasks.
- LLM-QAT: Data-Free Quantization Aware Training for Large Language Models by Meta AI and Reality Labs (https://arxiv.org/pdf/2305.17888.pdf).The paper proposes a data-free distillation method that leverages generations produced by the pre-trained model, which allows quantizing generative models independent of its training data, similar to post-training quantization methods. The method quantizes both weights and activations, and in addition the KV cache, which can be helpful for increasing throughput and support long sequence dependencies at current model sizes. Authors experiment with LLaMA models of sizes 7B, 13B, and 30B, at quantization levels down to 4- bits. They provide quite an extensive evaluation and compare results with modern quantization methods such as SmoothQuant.
- ZeroQuant-V2: Exploring Post-training Quantization in LLMs from Comprehensive Study to Low Rank Compensation by Microsoft (https://arxiv.org/pdf/2303.08302.pdf). The paper provides a thorough analysis of how the quantization of weights, activations, and weights-activations to different precisions (INT8 andINT4) impacts the accuracy of LLMs of different sizes and architectures (OPT and BLOOM). It states that weight quantization is less sensitive which aligns with common understanding. The authors also compare popular methods round-to-nearest (RTN), GPTQ, ZeroQuant with various quantization settings (per-row, per-group, per-block). Finally, they introduce a technique called Low Rank Compensation (LoRC), which employs low-rank matrix factorization on the quantization error matrix and achieves good accuracy results while being applied on top of other PTQ methods.
- NF4 Isn’t Information Theoretically Optimal (and that’s Good) by Toyota Technological Institute at Chicago (https://arxiv.org/pdf/2306.06965.pdf).Interesting research where the author studies a new NormalFloat4 data type proposed in QLoRA paper. He came up with the following conclusions: (1) The distribution of values to be quantized depends on the quantization block size, so an optimal code should vary with block size (2) NF4 does not assign an equal proportion of inputs to each code value (3) Codes which do have that property are not as good as NF4 for quantizing language models. He attempts to apply these insights to derive an improved code based on minimizing the expected L1 reconstruction error, rather than the quantile-based method. This leads to improved performance for larger quantization block sizes.
- SqueezeLLM: Dense-and-Sparse Quantization by BAIR UC Berkeley (https://arxiv.org/pdf/2306.07629.pdf). The paper shows that LLM (GPT) inference suffers from low arithmetic intensity and is a memory-bound problem. The authors then propose a non-uniform weight quantization method to trade computation for memory footprint. Two novel techniques are introduced. To deal with the outlying weight values, the Dense-and-Sparse decomposition factorizes a layer weight into a pair of matrices with the same shape as original – one for outliers which will be very sparse due to only~0.5% of large values, and the remaining elements are kept in another dense matrix. Since the sparse matrix has little non-zero elements, it can be stored as compressed sparse row (CSR) format without quantization and GEMM/GEMV can be realized via sparse library. As for the dense matrix with its range significantly narrowed than original, the authors formulate a Sensitivity-Based K-means Clustering that find 2bitwidth centroids by minimizing Fisher information metric, an approximate perturbation to the loss function that can be computed efficiently without the expensive 2nd order backprop. Across LLaMa 7B, 13B, 30B and its instruction-following derivatives Vicuna, SqueezeLLM in 4 or 3 bit consistently outperforms SOTA methods GPTQ,AWQ in perplexity evaluated on C4, WikiText-2 and zero-shot MMLU task. On A6000GPU, SqueezeLLM inference with a tailored LUT dequantization kernel show comparable latency to GPTQ.
- Towards Accurate for Vision Transformer by Meituan and Beihang University (https://arxiv.org/pdf/2303.14341.pdf).A practical study where authors highlight the problems of quantization for Vision Transformer models. They propose a bottom-elimination block wise calibration scheme to optimize the calibration metric to perceive the overall quantization disturbance in a block wise manner and prioritize the crucial quantization errors that influence more on the final output more. They also design a quantization scheme for Softmax to maintain the power-law character and keep the function of the attention mechanism. Experiments on various Vision Transformer architectures and tasks (Image Classification, Object Detection, Instance Segmentation) show that accurate 8-bit quantization is achievable for most of the models.
CVPR 2023 conference
- NIPQ: Noise proxy-based Integrated Pseudo-Quantization by Postech and Seoul National University (https://openaccess.thecvf.com/content/CVPR2023/papers/Shin_NIPQ_Noise_Proxy-Based_Integrated_Pseudo-Quantization_CVPR_2023_paper.pdf). Authors highlight a problem with straight-through estimator (STE) represented by a fact that it results in unstable convergence during quantization-aware training (QAT) leading in notable quality degradation. To resolve this issue, they suggest a novel approach called noise proxy-based integrated pseudo-quantization (NIPQ) that updates all quantization parameters (e.g., bit-width and truncation boundary)as well as the network parameters via gradient descent without STE instability. Experiments show that NIPQ outperforms existing quantization algorithms in various vision and language applications by a large margin. This approach is rather general and can be used to improve any QAT pipeline.
- One-Shot Model for Mixed-Precision Quantization by Huawei (https://openaccess.thecvf.com/content/CVPR2023/papers/Koryakovskiy_One-Shot_Model_for_Mixed-Precision_Quantization_CVPR_2023_paper.pdf). Authors focus on a problem of mixed precision quantization where an optimal bit width needs to be selected for every layer of a model. The suggested method, One-Shot MPS, learns optimal bit widths in a gradient-based manner and finds a diverse set of Pareto-front architectures in O(1) time. Authors claim that for large models the proposed method find optimal bit width partition 5 times faster than existing methods.
- Boost Vision Transformer with GPU-Friendly Sparsity and Quantization by Fudan University and Nvidia (https://openaccess.thecvf.com/content/CVPR2023/papers/Yu_Boost_Vision_Transformer_With_GPU-Friendly_Sparsity_and_Quantization_CVPR_2023_paper.pdf). In this paper authors suggest an approach to maximally utilize the GPU-friendly fine-grained 2:4 structured sparsity and quantization. Method consists of first pruning an FP16 vision transformer to a sparse representation and then quantizing it further to INT8/INT4 data types. his is done using multiple distillation losses in supervised or even unsupervised regimes. Experiments show about 3x performance boost for INT4quantization with less than 0.5% accuracy drop for classification, detection, and segmentation tasks.
- Q-DETR: An Efficient Low-Bit Quantized Detection Transformer by Beihang University, Zhongguancun Laboratory, Tencent and others (https://openaccess.thecvf.com/content/CVPR2023/papers/Xu_Q-DETR_An_Efficient_Low-Bit_Quantized_Detection_Transformer_CVPR_2023_paper.pdf). Authors show that during quantization of DETR detection transformer its accuracy is significantly degraded because of the information loss occurring in across-attention module. This issue it tackled by (1) distribution alignment of detection queries to maximize the self-information entropy and (2)foreground-aware query matching scheme to effectively transfer the teacher information to distillation-desired features. The resulting 4-bit Q-DETR can theoretically accelerate DETR with ResNet-50 backbone by 6.6x with only 2.6%accuracy drop.
- It also may be seen that some academic effort is targeted at data-free quantization. The following CVPR works suggest improvements in this direction: GENIE: Show Me the Data for Quantization (https://arxiv.org/pdf/2212.04780.pdf) by Samsung Research; Hard Sample Matters a Lot in Zero-Shot Quantization (https://arxiv.org/pdf/2303.13826.pdf) by South China University of Technology and others; Adaptive Data-FreeQuantization (https://arxiv.org/pdf/2303.06869.pdf) by Key Laboratory of Knowledge Engineering with Big Data and others.
Pruning
- DepGraph: Towards Any Structural Pruning by NUS, Zhejiang University and Huawei (https://arxiv.org/pdf/2301.12900.pdf). This paper tackles the highly challenging yet rarely explored aspects of structured pruning - generalized identification of dependent structures across layers for joint sparsification and removal. This problem is non-trivial, stemming from varying dependency in different model architectures, choice of pruning schemes as well as implementation. The authors start with all-to-all layer dependency, detailing the considerations and design decisions, gradually arriving at an intra and inter-layer dependency graph (DepGraph). The paper later shows how to use DepGraph to resolve grouping of dependent layer and structures. In experiments, norm-based regularization (L2) on derived groups is employed for sparsification training. The speedup and accuracy on CNN/CIFAR are competitive with many SOTA works. Most importantly, it shows the applicability of DepGraph not only for CNN but also for transformer, GNN and RNN, also the challenging DenseNet which has nested shortcut connection. This work is a good reference for any model optimization SW framework as structural dependency also exists for quantization and NAS. https://github.com/VainF/Torch-Pruning.
- Structural Pruning for Diffusion Models by NUS (https://arxiv.org/pdf/2305.10924.pdf).The paper introduces a method for Diffusion models pruning. The essence of the method is encapsulated in a Taylor expansion over pruned timesteps, a process that disregards non-contributory diffusion steps and ensembles informative gradients to identify important weights. Empirical assessment, undertaken across four diverse datasets shows that: the method enables approximately a 50%reduction in FLOPs at a mere 10% to 20% of the original training expenditure the pruned diffusion models inherently preserve generative behavior congruent with their pre-trained progenitors at low resolution. The code is available at https://github.com/VainF/Diff-Pruning.
- LLM-Pruner: On the Structural Pruning of Large Language Models by NUS (https://arxiv.org/pdf/2305.11627.pdf).The paper introduces a framework for the task-agnostic structural pruning of the large language model. The main advantage of the framework is the automatic structural pruning framework, where all the dependent structures are grouped without the need for any manual design. To evaluate the effectiveness of LLM-Pruner, authors conduct experiments on three large language models:LLaMA-7B, Vicuna-7B, and ChatGLM-6B. The compressed models are evaluated using nine datasets to assess both the generation quality and the zero-shot classification performance of the pruned models. The experimental results demonstrate that with the removal of 20% of the parameters, the pruned model maintains 93.6% of the performance of the original model after the light-weight fine-tuning.
- SpQR: A Sparse-Quantized Representation for Near-Lossless LLM Weight Compression by Neural Magic, Yandex, and universities of US, Europe and Russia (https://arxiv.org/pdf/2306.03078.pdf).The paper introduces new compressed format and quantization technique which enables near-lossless compression of LLMs across model scales, while reaching similar compression levels to previous methods. The method works by identifying and isolating outlier weights, which cause particularly large quantization errors, and storing them in higher precision, while compressing all other weights to 3-4 bits, and achieves relative accuracy losses of less than 1% in perplexity for highly-accurate LLaMA and Falcon LLMs. This makes it possible to run 33B parameter LLM on a single 24 GB consumer GPU without any performance degradation at 15% speedup. Code is available at: https://github.com/Vahe1994/SpQR.
- Dynamic Context Pruning for Efficient and Interpretable Autoregressive Transformers by ETH, CSEM, University of Basel (https://arxiv.org/pdf/2305.15805.pdf).The paper aims to overcome the length-quadratic complexity of the global causal attention and argues that static sparse attention (such as Big Bird, Sparse Attention) is sub-optimal in modeling, requiring pretraining from scratch, and has limited memory benefit during generation. The authors propose a fine-tuning method to learn layer-specific sparse attention which adaptively prune tokens in the context during deployment. Essentially, auxiliary modules are introduced a teach layer to learn the interactions between query and key to predict the retention of tokens. Although additional cost is incurred, high sparsity in long context results in overall memory benefit by reducing storage and retrieval from key-value cache, as well as compute benefit from lesser tokens for attention computation. As compared to adapting GPT2 with local and sparse attention, online context pruning shows lower (better) perplexity and retains perplexity when up to 60% of tokens pruned from context. Mean zero-shot accuracy across a number of tasks is shown maintained at a high level of sparsity.
- Revisiting Token Pruning for Object Detection and Instance Segmentation by University of Zurich (https://arxiv.org/pdf/2306.07050.pdf).In this paper, authors investigate token pruning to accelerate inference for object detection and instance segmentation, extending prior works from image classification. Through the experiments, they offer four insights for dense tasks: (i) tokens should not be completely pruned and discarded, but rather preserved in the feature maps for later use. (ii) reactivating previously pruned tokens can further enhance model performance. (iii) a dynamic prunin grate based on images is better than a fixed pruning rate. (iv) a lightweight,2-layer MLP can effectively prune tokens, achieving accuracy comparable with complex gating networks with a simpler design. Authors evaluate the impact of these design choices on COCO dataset and present a method integrating these insights that outperforms prior art token pruning models, significantly reducing performance drop from ∼1.5 mAP to ∼0.3 mAP for both boxes and masks.
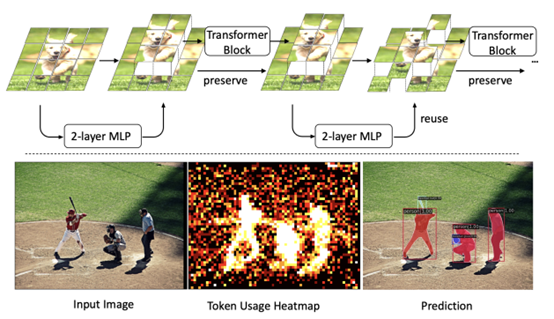
- PRUNING MEETS LOW-RANK PARAMETER-EFFICIENT FINE-TUNING by Zhejiang University and Monash University (https://arxiv.org/pdf/2305.18403.pdf).The paper introduces a parameter importance criterion for large pre-trained models that works with LoRA. With the gradients of the low-rank decomposition, it can approximate the importance of pre-trained parameters without a need to compute their gradients. Based on this, authors introduce LoRA Prune, an approach that unifies PEFT with pruning. Experiments on computer vision and natural language processing tasks demonstrate that LoRA Prune outperforms the compared pruning methods and achieves competitive performance with other (quantization-based) PEFT methods.
CVPR 2023 conference
- Integral Neural Networks by Thestage.ai and Huawei (https://openaccess.thecvf.com/content/CVPR2023/papers/Solodskikh_Integral_Neural_Networks_CVPR_2023_paper.pdf). A new family of deep neural networks called Integral Neural Networks (INN) is introduced in this paper. The weights of INNs are represented as continuous N-dimensional functions, and they are applied by continuous integration operation. During inference, continuous layers can be discretized into fixed representation with an arbitrary resolution. This can be used to prune the model to a desired degree without any fine-tuning and suffering only a small performance loss. Authors also suggest how a pre-trained CNN can be converted to INN. Results show that INNs achieve the same accuracy as CNNs while performing much better when pruned without fine-tuning. For example, a 30% pruned Integral ResNet18 has a 2% accuracy drop on ImageNet compared to 65% accuracy drop for a regular ResNet18.
- Joint Token Pruning and Squeezing Towards More Aggressive Compression of Vision Transformers by MEGVII Technology and Tsinghua University (https://openaccess.thecvf.com/content/CVPR2023/papers/Wei_Joint_Token_Pruning_and_Squeezing_Towards_More_Aggressive_Compression_of_CVPR_2023_paper.pdf). Authors attempt to improve vision transformer computational costs by pruning redundant tokens. Unlike traditional token pruning methods, the proposed Token Pruning & Squeezing module (TPS)approach also squeezes the pruned tokens into the kept ones according to their similarity. Experiments on various ViTs demonstrate the effectiveness of the method and higher robustness to the errors of the token pruning policy .Especially, for DeiT-tiny and -small TPS shrinks computational budget by 35% while improving the accuracy by 1-6% compared to baselines on ImageNet classification.
- Global Vision Transformer Pruning with Hessian-Aware Saliency by Nvidia, Berkeley and Duke University (https://arxiv.org/pdf/2110.04869.pdf). Authors propose a first systematic approach to global structural pruning of vision transformers by redistributing the parameters both across transformer blocks and between different structures within the block. Pruning is performed according to a novel Hessian-based criteria comparable across all layers and structures. A new architecture of ViT is proposed called Novel ViT (NViT) obtained by iterative pruning of DeiT-Base. NViT-Base achieves 2.5x FLOPs reduction and 1.9x performance speedup with almost no accuracy degradation. Based on this and other results authors claim outperforming prior state of the art by a large margin.
Neural Architecture Search
- PreNAS: Preferred One-Shot Learning Towards Efficient Neural Architecture Search by Alibaba Group (https://arxiv.org/pdf/2304.14636.pdf ). The authors demonstrate the use of zero-cost proxies to accelerate the training and improve the sample efficiency of weight-sharing NAS. Their method groups Transformer isomers and discards a subset of each group based on each architecture configuration zero-cost score. The reduced search space is then used during training. Pre-NAS outperforms alternative state-of-the-start NAS methods for both Vision Transformers and architectures based on convolution operations.
- Mixture-of-Supernets: Improving Weight-Sharing Supernet Training with Architecture-Routed Mixture-of-Experts by Meta and the University of British Columbia (https://arxiv.org/abs/2306.04845). Authors tackle several issues in traditional weight-sharing NAS, i.e., in NLP tasks, there is an observed performance gap between the selected architectures and training the same architectures from scratch, and additional training is required to improve the final accuracy of the Pareto front. The proposed method uses Mixture of Experts (MoE) to improve the underlying weight-sharing mechanisms. The authors demonstrate the approach using machine translation models, which achieve state-of-the-art performance.
- LayerNAS: Neural architecture search in polynomial complexity by Google Research (https://arxiv.org/pdf/2304.11517.pdf). Authors propose LayerNAS, an algorithm that enforces a sequential search process, transforming multi-objective NAS into a combinatorial optimization problem. LayerNAS outperforms several NAS baseline models in top 1 accuracy. However, it often obtains these improvements with models that have a larger number of parameters and MAdds. LayerNAS is not as efficient as One-shot NAS, but future work will attempt the application of LayerNAS insights into One-shot NAS approaches.
Other
- Inference with Reference: Lossless Acceleration of Large Language Models by Microsoft (https://arxiv.org/pdf/2304.04487.pdf). Authors study accelerating LLM’s inference by improving the efficiency of auto regressive decoding. Authors observe what in many real-world applications an LLM’s output tokens often come from its context and they propose LLMA, inference-with-reference decoding mechanism to accelerate LLM inference by exploiting the overlap between an LLM’s output and reference that is available for many practical scenarios. Experiments show that LLMA method can generate identical results as greedy decoding but achieve over 2x speed-up across different model sizes in practical application scenarios like retrieval-augmented and cache-assisted generation.
- Scaling Down to Scale Up: A guide to Parameter-Efficient Fine-Tuning by The University of Massachusetts (https://arxiv.org/pdf/2303.15647.pdf).This survey summarizes over 40 papers related to parameter-efficient fine-tuning methods between February 2019 and February 2023. It provides taxonomy (see figure 2) and highlights key methods in each category with pseudocodes, and compares qualitatively in the aspects of storage, backprop, and inference efficiency (Table 1). It is a good time-saving paper to keep up with the space.
- On Architectural Compression of Text-to-Image Diffusion Models by Nota Inc. Korea (https://arxiv.org/pdf/2305.15798.pdf).This work compresses pretrained Stable Diffusion (v1.4) by handpicked removal of multiple residual and attention blocks in the denoising UNet. The derived models are subsequently trained with diffusion loss in conjunction with knowledge distillation to match teacher’s noise prediction and intermediate feature maps. Authors produce 3 models (base, small, tiny) with each training only utilizing a single A100 GPU and 0.1% of text-image pairs from LAION AestheticsV2 6.5+. On Xeon Cascade Lake and RTX3090, latency of a single 512x512 text-to-image generation (25-step denoising) has been shown to improve by 30-45%. Authors also show the applicability of the distilled models for Dream Booth personalization, demonstrating up to 99% performance of Dream Booth with original Stable Diffusion model.
Deep Learning Software
- JaxPruner: A Concise Library for Sparsity Research by Google Research (https://arxiv.org/pdf/2304.14082.pdf). Google Research open-sources weight pruning framework for the research of network sparsification and sparse network training in Jax ecosystem. JaxPruner works seamlessly with popular Jax Optimizer (Optax) and provides a common abstraction for weight masking, mask update scheduler, pruning regularity, sparse training (straight through estimator) and sparse model storage format. In the companion paper, JaxPruner implements a set of baseline sparsity algorithms and demonstrates easy integration to Jax framework of various domains such as FedJax (Federated Learning), t5x (NLP), Dopamine & Acme(Deep RL). See https://github.com/google-research/jaxpruner for more details.
Q1'23: Technology update – low precision and model optimization
Authors
Alexander Kozlov, Nikolay Lyalyushkin, Pablo Munoz, Vui Seng Chua, Alexander Suslov, Yury Gorbachev, Nilesh Jain
Summary
We continue following the trends and reviewing papers and posts for your convenience. This quarter we observed quite a lot of new methods, and one of the main focuses is the optimization of Large Language Models which are started being adopted by the industry. Please pay attention to Token Merging, GPTQ, and FlexGen works which introduce interesting methods and show very promising results.
Papers with notable results
Quantization
- CSMPQ: CLASS SEPARABILITYBASED MIXED-PRECISION QUANTIZATION by universities of China (https://arxiv.org/pdf/2212.10220.pdf). The paper introduces the class separability of layer-wise feature maps to search for optimal quantization bit-width. Essentially, authors leverage the TF-IDF metric from NLP to measure the class separability of layer-wise feature maps that are averaged across spatial dimensions. The method can be applied on top of the existing quantization algorithms, such as BRECQ and delivers good results, e.g., 71.30% top-1 acc with only 1.5Mb on MobileNetV2.
- Understanding INT4 Quantization for Transformer Models: Latency Speedup, Composability, and Failure Cases by Microsoft (https://arxiv.org/abs/2301.12017). Show that INT4 quantization for LM does not greatly reduce the quality of encoder-only and encoder-decoder models (e.g. BERT, BART). Even with 50%sparsity accuracy drop is within 1% on MNLI. The authors provide an analysis of problems with decoder-only models (e.g., GPT). The method will be part of DeepSpeed.
- A Practical Mixed Precision Algorithm for Post-Training Quantization by Qualcomm AI Research (https://arxiv.org/pdf/2302.05397.pdf). In this paper, authors propose two-phase algorithm to solve the problem of mixed precision quantization in the post-training quantization setting. In the first phase, they create a per-layer sensitivity list by measuring the loss(SQNR) of the entire network with different quantization options for each layer. The second phase of the algorithm starts with the entire network quantized to the highest possible bitwidth, after which based on the sensitivity list created in phase 1, they iteratively flip the least sensitive quantizers to lower bit-width options until the performance budget is met or our accuracy requirement gets violated. The method shows comparable results for various models including CV and NLP.
- LUT-NN: Towards Unified Neural Network Inference by Table Lookup by Microsoft Research, Chinese Universities (https://arxiv.org/abs/2302.03213). Development of the idea of product quantization "multiplications without multiplications" – pre-calculate multiplications of "typical" numbers and in runtime, instead of multiplication and addition they do a lookup in the table. The accuracy is lower than the baseline networks, but way better than in previous methods. Latency-wise, the real speedup of LUT-NN is up to 7x for BERT and 2x for ResNet on CPU.
- Oscillation-free Quantization for Low-bit Vision Transformers by Hong Kong University of Science and Technology and Reality Labs, Meta (https://arxiv.org/pdf/2302.02210.pdf). In this work, authors are aiming at ultra-low-bit quantization of vision transformer models. They propose three techniques to address the problem of weight oscillation when quantizing to low-bits: statistical weight quantization to improve quantization robustness compared to the prevalent learnable-scale-based method; confidence-guided annealing that freeze sthe weights with high confidence and calms the oscillating weights; and query-key reparameterization to resolve the query-key intertwined oscillation and mitigate the resulting gradient misestimation. The method shows state-of-the-art results when quantizing DeiT-T/DeiT-S models to 2 and 4 bits.
- Mixed Precision Post Training Quantization of Neural Networks with Sensitivity Guided Search by University of Notre Dame and Google (https://arxiv.org/pdf/2302.01382.pdf). Authors are aiming at building an optimal bitwidth search algorithm. They conduct an analysis of metrics to of quantization error as well as two sensitivity-guided search algorithms. They found that a combination of Hessian trace + Gready search gives the best results in their setup. Experimental results show latency reductions of up to 27.59% (ResNet50) and 34.31% (BERT).
- Teacher Intervention: Improving Convergence of Quantization Aware Training for Ultra-Low Precision Transformers by Hanyang University and Seoul National Universities (https://arxiv.org/pdf/2302.11812.pdf).One more paper that claims benefits from knowledge distillation between intermediate layers of Transformer models during optimization. In this case, authors apply Quantization-aware Training at ultra-low bit width setup (e.g. ternary quantization). They perform an extensive analysis of KD on the training stability and convergence at multiple settings and do evaluation both on NLP and CV Transformer models.
- POWERQUANT: AUTOMORPHISMSEARCH FOR NONUNIFORM QUANTIZATION by Sorbonne University and Datakalab (https://arxiv.org/pdf/2301.09858.pdf). The paper proposes a non-uniform data-free quantization method that is, essentially, a modification of uniform quantization with exponent parameter alpha that is tuned during the quantization process. The method shows its effectiveness when applying 8 and 4 bits quantization to various types of models including Conv, RNN and Transformer models.
- GPTQ: Accurate Post-Training Quantization for Generative Pre-trained Transformers by IST Austria, ETH Zurich, and Neural Magic (https://arxiv.org/abs/2210.17323). Authors argue that contemporary PTQ methods such as AdaRound, BRECQ, ZeroQuant are too costly to quantize massive-scale LLM. GPTQ is an extension of Hessian-based post-training quantization method, Optimal Brain Quantization(OBQ) to scale up the process efficiently for billion parameters LLM which takes only minutes to quantize weight of 3 billion GPT and 4 hours for OPT-175Bon a single A100 GPU. The papers show that 3-bit weight quantized OPT-175B can be fit into a single 80GB A100 which would otherwise require 5xA100 for FP16,4xA100 for Int8 (SmoothQuant).The optimized model achieves >3X latency improvement with a custom dequantization kernel for FP16 inference. Although the work does not map to Int8 engine, it is a strong indication that mix low-bit weight (<8bit) and8-bit activation could further alleviate the memory footprint and bandwidth bottleneck in LLM by incurring a low-overhead weight dequantization. Code is available at: https://github.com/IST-DASLab/gptq.
Pruning
- SparseGPT: Massive Language Models Can Be Accurately Pruned In One-shot by IST Austria and Neural Magic (https://arxiv.org/abs/2301.00774). The layer-wise pruning decisions are based on series of careful approximations of the inverse Hessian of the data. LLM can be pruned to at least 50% sparsity in one-shot, without any retraining, at minimal loss of accuracy for LLM. SparseGPT generalizes to semi-structured (2:4 and 4:8) patterns and is compatible with weight quantization approaches.
- ZipLM: Hardware-Aware Structured Pruning of Language Models by IST Austria and Neural Magic (https://arxiv.org/pdf/2302.04089.pdf). The idea is to prune gradually based on measured latency for different number of attention heads and FFN shapes. The pruning decisions are based on estimation of the inverse Hessian of the data. Using it they obtain the optimal layer-wise mask and weight update to preserve original intermediate outputs. To recover accuracy after pruning they tune with 2 distillation losses: with teacher outputs and with intermediate token representations across the entire model. 2x faster BERT-large than the Block Movement Pruning algorithm for the same accuracy. ZipLM can match the performance of highly optimized MobileBERT model by simply compressing the baseline BERT architecture. Authors plan to open-source the framework as part of SparseML.
- R-TOSS: A Framework for Real-Time Object Detection using Semi-Structured Pruning by Colorado State University (https://arxiv.org/ftp/arxiv/papers/2303/2303.02191.pdf). A practical study on semi-structured pruning of ConvNets. Authors propose a method that generates a set of sparse patterns for the model and applies them to introduce the sparsity during the training. The same set is passed to the runtime to precompile the sparse kernels. They also propose a way how to spread the same idea to 1x1 Convs that are dominant in contemporary architectures. The method is applied to YOLOv5 and RetinaNet models and its efficiency is evaluated on Jetson TX2 platform.
- Dynamic Structure Pruning for Compressing CNNs by Korea University (https://arxiv.org/pdf/2303.09736.pdf). Interesting work on the structured pruning where all the filters of each operation are being split into the groups and each group is pruned independently along the input channel dimension. One can imagine that each operation is being split into several operations and each operates on its own portion of input channels (ala grouped convolution). Authors also propose a differentiable group learning method that can optimize filter groups using gradient-based methods during training. The method shows better efficiency compared to Filter pruning methods. Code is available at https://github.com/irishev/DSP.

- Automatic Attention Pruning: Improving and Automating Model Pruning using Attentions by Arizona State University and Meta (https://arxiv.org/pdf/2201.10520.pdf). Authors propose an iterative, structured pruning approach for finding the “winning ticket” models that are hardware efficient. They also implement an attention-based mechanism for accurately identifying unimportant filters for pruning, which is much more effective than existing methods as well as an adaptive pruning method that can automatically optimize the pruning process according to diverse real-world scenarios. Method shows comparable results for a variety of CV model architectures. Code is at: https://github.com/kaiqi123/Automatic-Attention-Pruning.git.
- Efficient Spatially Sparse Inference for Conditional GANs and Diffusion Models by CMU, MITand Stanford University. Motivated by the high unedited region during interactive image editing that translates to activation sparsity relative to previous generation, the authors propose Sparse Incremental Generative Engine (SIGE). SIGE employs tile-based sparse convolution to compute modified region in input activation and update to the cached output activation of the previous generation. SIGE is intelligently designed with joint Scatter-Gather kernel to avoid memory overheads and fuses element-wise operations. The paper shows superior synthesis fidelity (PSNR,LPIPS, FID) for the task of incremental inpainting as compared to weight pruning at similar MAC reduction ratio. The authors also extensively benchmark latency of SIGE applied to DDIM, PD, GauGan on Nvidia RTXs, Apple M1 Pro and Intel i9 workstation. Speedup can be up to 14X depending on percentage of edited region. Code: https://github.com/lmxyy/sige.
- Token Merging: Your ViT but faster by Georgia Tech and Meta AI (https://arxiv.org/pdf/2210.09461.pdf). As opposed to token pruning, the authors unveil runtime token merging (ToMe) modules inserted between attention and feed forward layer in vision transformer (ViT) which reduce number of tokens successively in every transformer block up to 98% tokens in final block, easily achieve substantial acceleration up to 2X without the need to train. During runtime, ToMe employs bipartite soft matching algorithm to merge similar tokens and is as lightweight as randomly dropping tokens. When accuracy degradation is high, authors devise a training mechanism for ToMe by mapping its backpropagation like average pooling. Its training efficiency improves considerably, 1.5X as compared to learning-based token pruning. The paper shows thorough ablation on design choices of matching algorithm, token merging schedule etc. and a plethora of accuracy-speedup results on off-the-shelf ViT trained with different supervised/self-supervision for image, video, and audio data. The work is featured in Meta Research blog and claimed to accelerate Stable Diffusion’s text-to-image generation by 1.7X without loss of visual quality. Code: https://github.com/facebookresearch/ToMe.
Neural Architecture Search
- Neural Architecture Search: Insights from 1000 Papers by Universities and Abacus AI (https://arxiv.org/pdf/2301.08727.pdf). A big survey of the many recent NAS methods. The document provides a good organization of various approaches and nice illustrations of different techniques.
- Enhancing Once-For-All: A Study on Parallel Blocks, Skip Connections and Early Exits by DEIB, Politecnico di Milano (https://arxiv.org/abs/2302.01888). The authors propose OFAv2, an extension of OFA aimed at improving its performance. The extension to the original OFA includes early exits, parallel blocks and dense skip connections. The training phase is extended with two new phases: Elastic Level and Elastic Height. The authors also include a new Knowledge Distillation technique to handle multi-output networks. The results are quite impressive. In OFAMobileNetV3, OFAv2 reaches up to 12.07% improvement in accuracy compared to the original OFA.
- DDPNAS: Efficient Neural Architecture Search via Dynamic Distribution Pruning by Xiamen University and Tencent(https://link.springer.com/article/10.1007/s11263-023-01753-6). The authors propose a framework, DDPNAS, that is used to dynamically prune the search space, and accelerate the search stage. However, this acceleration requires a more complex training stage, in which to find the optimal probability distribution of possible architectures, the approach samples a set of architectures that are trained and validated, and once the distribution has been updated, the operations with the lowest probability are pruned from these arch space.
- DetOFA: Efficient Training of Once-for-All Networks for Object Detection by Using Pre-trained Supernet and Path Filter by Sony Group Corporation (https://arxiv.org/pdf/2303.13121v1.pdf).The authors propose a new performance predictor called path filter. This predictor can accurately predict the relative performance of models in the same resource bucket. Using the information obtained from path filter, DetOFA prunes the search space and reduce the computational cost of identifying good subnetworks. This approach produces better-performing super-networks for object detection and a reduction in the cost of >30% compared with the vanilla once-for-all approach.
Other
- NarrowBERT: Accelerating Masked Language Model Pretraining and Inference by University of Washington (https://arxiv.org/pdf/2301.04761.pdf). Propose two simple methods to accelerate training/inference of transformers. Utilize the idea that training prediction occurs only for masked tokens, and on inference in many problems, representation is used only for the [CLS] token. In the first approach they calculate the attention (s) on all tokens only at the beginning of the network, and then perform linear layers (f) only for the desired tokens (masked or CLS). In the second - calculate the attention (s) on all tokens only at the beginning of the network, and then generate an attention only for the necessary tokens. Shows 3.5x boost on MNLI inference.
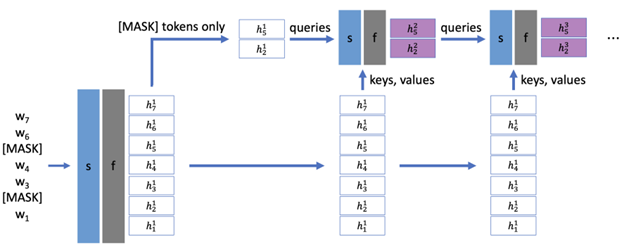
- TAILOR: Altering Skip Connections for Resource-Efficient Inference by UC San Diego, MIT and AMD (https://arxiv.org/abs/2301.07247). Continuation of the ideas of RepVGG - they remove or at least shorten the skip connection for more efficient inference: they do not store intermediate activations and save on memory. The model with the removed skip connections is distilled with a float version of itself to roughly preserve the original accuracy. The optimized hardware designs improve resource utilization by up to34% for BRAMs, 13% for FFs, and 16% for LUTs.
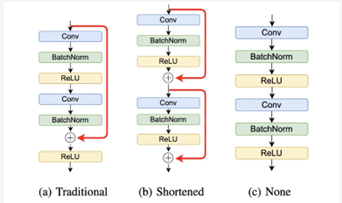
- Offsite-Tuning: Transfer Learning without Full Model by Massachusetts Institute of Technology (https://arxiv.org/pdf/2302.04870v1.pdf). In this paper, authors propose a transfer learning framework that can adapt large foundation models to downstream data without access to the full model. The setup assumes that the model owner sends a lightweight adapter and a lossy compressed emulator to the data owner, who then fine-tunes the adapter on the down stream data with the emulator’s assistance. The fine-tuned adapter is then returned to the model owner, who plugs it into the full model to create an adapted foundation model. The method can achieve comparable accuracy as full model fine-tuning while being privacy-preserving and efficient, achieving 6.5×speedup and 5.6× memory reduction. Code is available at: https://github.com/mit-han-lab/offsite-tuning.
- High-throughput Generative Inference of Large Language Models with a Single GPU by Stanford University, UC Berkeley, ETH Zurich, Yandex, HSE University, Meta, Carnegie Mellon University (https://arxiv.org/pdf/2303.06865.pdf). The paper introduces FlexGen, a high-throughput generation engine for running LLMs with limited GPU memory. It can be flexibly configured under various hardware resource constraints by aggregating memory and computation from the GPU, CPU, and disk. Through a linear programming optimizer, it searches for the best pattern to store and access the tensors, including weights, activations, and attention key/value (KV) cache. FlexGen further compresses both weights and KV cache to 4 bits with negligible accuracy loss. It achieves up to 100× higher throughput compared to state-of-the-art offloading systems. The FlexGen library runs OPT-175B up to 100× faster on a single 16GB GPU. Faster than deepspeed offloading. Code is available here: https://github.com/FMInference/FlexGen
Accelerate Inference of Sparse Transformer Models with OpenVINO™ and 4th Gen Intel® Xeon® Scalable Processors
Authors: Alexander Kozlov, Vui Seng Chua, Yujie Pan, Rajesh Poornachandran, Sreekanth Yalachigere, Dmitry Gorokhov, Nilesh Jain, Ravi Iyer, Yury Gorbachev
Introduction
When it comes to the inference of overparametrized Deep Neural Networks, perhaps, weight pruning is one of the most popular and promising techniques that is used to reduce model footprint, decrease the memory throughput required for inference, and finally improve performance. Since Language Models (LMs) are highly overparametrized and contain lots of MatMul operations with weights it looks natural to prune the redundant weights and benefit from sparsity at inference time. There are several types of pruning methods available:
- Fine-grained pruning (single weights).
- Coarse pruning: group-level pruning (groups of weights), vector pruning (rows in weights matrices), and filter pruning (filters in ConvNets).
Contemporary Language Models are basically represented by Transformer-based architectures. Using coarse pruning methods for such models is problematic because of the many connections between the layers. This trait means that, first, not every pruning type is applicable to such models and, second, pruning of some dimension in one layer requires adjustments in the rest of the layers connected to it.
Fine-grained sparsity does not have such a constraint and can be applied to each layer independently. However, it requires special support on the HW and inference SW level to get real performance improvements from weight sparsity. There are two main approaches that help to leverage from weight sparsity at inference:
- Skip multiplication and addition for zero weights in dot products of weights and activations. This usually results in a special instruction set that implements such logic.
- Weights compression/decompression to reduce the memory throughput. Compression is performed at the model load/compilation stage while decompression happens on the fly right before the computation when weights are in the cache. Such a method can be implemented on the HW or SW level.
In this blog post, we focus on the SW weight decompression method and showcase the end-to-end workflow from model optimization to deployment with OpenVINO.
Sparsity support in OpenVINO
Starting from OpenVINO 2022.3release, OpenVINO runtime contains a feature that enables weights compression/decompression that can lead to performance improvement on the 4thGen Intel® Xeon® Scalable Processors. However, there are some prerequisites that should be considered to enable this feature during the model deployment:
- Currently, this feature is available only to MatMul operations with weights (Fully-connected layers). So currently, there is no support for sparse Convolutional layers or other operations.
- MatMul layers should contain a high level of weights sparsity, for example, 80% or higher which is achievable, especially for large Transformer models trained on simple tasks such as Text Classification.
- The deployment scenario should be memory-bound. For example, this prerequisite is applicable to cloud deployment when there are multiple containers running inference of the same model in parallel and competing for the same RAM and CPU resources.
The first two prerequisites assume that the model is pruned using special optimization methods designed to introduce sparsity in weight matrices. It is worth noting that pruning methods require model fine-tuning on the target dataset in order to reduce accuracy degradation caused by zeroing out weights within the model. It assumes the availability of the HW capable of DL model training. Nowadays, many frameworks and libraries offer such methods. For example, PyTorch provides some capabilities for NN pruning. There are also resources that offer pre-trained sparse models that can be used as a starting point, for example, SparseZoo from Neural Magic.
OpenVINO also provides instruments for DL model pruning implemented in Neural Network Compression Framework (NNCF) that is aimed specifically for model optimization and offers different optimization options: from post-training optimization to deep compression when stacking several optimization methods. NNCF is also integrated into Hugging Face Optimum library which is designed to optimize NLP models from Hugging Face Hub.
Using only sparsity is not so beneficial compared to another popular optimization method such as bit quantization which can guarantee better performance-accuracy trade-offs after optimization in the general case. However, the good thing about sparsity is that it can be stacked with 8-bit quantization so that the performance improvements of one method reinforce the optimization effect of another one leading to a higher cumulative speedup when applying both. Considering this, OpenVINO runtime provides an acceleration feature for sparse and 8-bit quantized models. The runtime flow is shown in the scheme below:

Below, we demonstrate two end-to-end workflows:
- Pruning and 8-bit quantization of the floating-point BERT model using Hugging Face Optimum and NNCF as an optimization backend.
- Quantization of sparse BERT model pruned with 3rd party optimization solution.
Both workflows end up with inference using OpenVINO API where we show how to turn on a runtime option that allows leveraging from sparse weights.
Pruning and 8-bit quantization with Hugging Face Optimum and NNCF
This flow assumes that there is a Transformer model coming from the Hugging Face Transformers library that is fine-tuned for a downstream task. In this example, we will consider the text classification problem, in particular the SST2 dataset from the GLUE benchmark, and the BERT-base model fine-tuned for it. To do the optimization, we used an Optimum-Intel library which contains the optimization capabilities based on the NNCF framework and is designed for inference with OpenVINO. You can find the exact characteristics and steps to reproduce the result in this model card on the Hugging Face Hub. The model is 80% sparse and 8-bit quantized.
To run a pre-optimized model you can use the following code from this notebook:
Quantization of already pruned model
In case if you deal with already pruned model, you can use Post-Training Quantization from the Optimum-Intel library to make it 8-bit quantized as well. The code snippet below shows how to quantize the sparse BERT model optimized for MNLI dataset using Neural Magic SW solution. This model is publicly available so that we download it using Optimum API and quantize on fly using calibration data from MNLI dataset. The code snippet below shows how to do that.
Enabling sparsity optimization inOpenVINO Runtime and 4th Gen Intel® Xeon® Scalable Processors
Once you get ready with the sparse quantized model you can use the latest advances of the OpenVINO runtime to speed up such models. The model compression feature is enabled in the runtime at the model compilation step using a special option called: “CPU_SPARSE_WEIGHTS_DECOMPRESSION_RATE”. Its value controls the minimum sparsity rate that MatMul operation should have to be optimized at inference time. This property is passed to the compile_model API as it is shown below:
An important note is that a high sparsity rate is required to see the performance benefit from this feature. And we note again that this feature is available only on the 4th Gen Intel® Xeon® Scalable Processors and it is basically for throughput-oriented scenarios. To simulate such a scenario, you can use the benchmark_app application supplied with OpenVINO distribution and limit the number of resources available for inference. Below we show the performance difference between the two runs sparsity optimization in the runtime:
- Benchmarking without sparsity optimization:
- Benchmarking when sparsity optimization is enabled:
Performance Results
We performed a benchmarking of our sparse and 8-bit quantized BERT model on 4th Gen Intel® Xeon® Scalable Processors with various settings. We ran two series of experiments where we vary the number of parallel threads and streams available for the asynchronous inference in the first experiments and we investigate how the sequence length impact the relative speedup in the second series of experiments.
The table below shows relative speedup for various combinations of number of streams and threads and at the fixed sequence length after enabling sparsity acceleration in the OpenVINO runtime.

Based on this, we can conclude that one can expect significant performance improvement with any number of streams/threads larger than one. The optimal performance is achieved at eight streams/threads. However, we would like to note that this is model specific and depends on the model architecture and sparsity distribution.
The chart below also shows the relationship between the possible acceleration and the sequence length.

As you can see the benefit from sparsity is decreasing with the growth of the sequence length processed by the model. This effect can be explained by the fact that for larger sequence lengths the size of the weights is no longer a performance bottleneck and weight compression does not have so much impact on the inference time. It means that such a weight sparsity acceleration feature does not suit well for large text processing tasks but could be very helpful for Question Answering, Sequence Classification, and similar tasks.
References
Accelerate Inference of Hugging Face Transformer Models with Optimum Intel and OpenVINO™
Authors: Xiake Sun, Kunda Xu
1. Introduction

Hugging Face is a large open-source community that quickly became an enticing hub for pre-trained deep learning models across Natural Language Processing (NLP), Automatic Speech Recognition(ASR), and Computer Vision (CV) domains.
Optimum Intel provides a simple interface to optimize Transformer models and convert them to OpenVINO™ Intermediate Representation (IR) format to accelerate end-to-end pipelines on Intel® architectures using OpenVINO™ runtime.
Sentimental classification, as one of the popular NLP tasks, is the automated process of identifying opinions in the text and labeling them as positive or negative. In this blog, we use DistilBERT for the sentimental classification task as an example to show how Optimum Intel helps to optimize the model with Neural Network Compression Framework (NNCF) and accelerate inference with OpenVINO™ runtime.
2. Setup Environment
Install optimum-intel and its dependency in a new python virtual environment as follow:
3. Model Inference with OpenVINO™ Runtime
The Optimum inference models are API compatible with Hugging Face Transformers models; which means you could simply replace Hugging Face Transformer “AutoModelXXX” class with the “OVModelXXX” class to switch model inference with OpenVINO™ runtime. You could set “from_transformers=True” when loading the model with the from_pretrained() method, the loaded model will be automatically converted to an OpenVINO™ IR for inference with OpenVINO™ runtime.
Here is an example of how to perform inference with OpenVINO™ runtime for a sentimental classification task, the output of the pipeline consists of classification label (positive/negative) and corresponding confidence.
4. Model Quantization with NNCF framework
Most deep learning models are built using 32 bits floating-point precision (FP32). Quantization is the process to represent the model using less memory with minimal accuracy loss. To further optimize model performance on Intel® architecture via Intel® Deep Learning Boost, model quantization as 8 bits integer precision (INT8) is required.
Optimum Intel enables you to apply quantization on Hugging Face Transformer Models using the NNCF. NNCF provides two mainstream quantization methods - Post-Training Quantization (PTQ) and Quantization-Aware Training (QAT).
- Post-Training Quantization (PTQ) refers to quantizing a model with a representative calibration dataset without fine-tuning.
- Quantization-Aware Training (QAT) is applied to simulate the effects of quantization during training to mitigate its effect on the model’s accuracy
4.1. Model Quantization with NNCF PTQ
NNCF Post-training static quantization introduces an additional calibration step where data is fed through the network to compute the activations quantization parameters. Here is how to apply static quantization on a pre-trained DistilBERT using General Language Understanding Evaluation (GLUE) dataset as the calibration dataset:
The quantize() method applies post-training static quantization and export the resulting quantized model to the OpenVINO™ Intermediate Representation (IR), which can be deployed on any target Intel® architecture.
4.2. Model Quantization with NNCF QAT
Quantization-Aware Training (QAT) aims to mitigate model accuracy issue by simulating the effects of quantization during training. If post-training quantization results in accuracy degradation, QAT can be used instead.
NNCF provides an “OVTrainer” class to replace Hugging Face Transformer’s “Trainer” class to enable quantization during training with additional quantization configuration. Here is an example on how to fine-tune a DistilBERT with Stanford Sentiment Treebank (SST) dataset while applying quantization aware training (QAT):
4.3. Comparison of FP32 and INT8 model outputs
“OVModelForXXX” class provided the same API to load FP32 and quantized INT8 OpenVINO™ models by setting “from_transformers=False”. Here is an example of how to load quantized INT8 models optimized by NNCF and inference with OpenVINO™ runtime.
Here is an example for sentimental classification output of FP32 and INT8 models:

5. Mitigation of accuracy issue cause by saturation
8-bit instructions of old CPU generations (based on SSE,AVX-2, AVX-512 instruction sets) are prone to so-called saturation(overflow) of the intermediate buffer when calculating the dot product, which is an essential part of Convolutional or MatMul operations. This saturation can lead to a drop in accuracy when running inference of 8-bit quantized models on the mentioned architectures. The problem does not occur on GPUs or CPUs with Intel® Deep Learning Boost (VNNI) technology and further generations.
In the case a significant difference in accuracy (>1%) occurs after quantization with NNCF default quantization configuration, here is an example code to check if deployed platform supports Intel® Deep Learning Boost (VNNI) and further generations:
While quantizing activations use the full range of 8-bit data types, there is a workaround using only 7 bits to represent weights (of Convolutional or Fully-Connected layers) to mitigate saturation issue for many models on old CPU platform.
NNCF provides three options to deal with the saturation issue. The options can be enabled in the NNCF quantization configuration using the “overflow_fix” parameter:
- "disable": (default) option do not apply saturation fix at all
- "enable": option to apply for all layers in the model
- "first_layer_only": option to fix saturation issue for the first layer
Here is an example to enable overflow fix in quantization configuration to mitigate accuracy issue on old CPU platform:
After model quantization with updated quantization configuration with NNCF PTQ/NNCF, you can repeat step 4.3 to verify if quantized INT8 model inference results are consistent with FP32 model outputs.
Additional Resources
Provide Feedback & Report Issues
Notices & Disclaimers
Intel technologies may require enabled hardware, software, or service activation.
No product or component can be absolutely secure.
Your costs and results may vary.
Intel does not control or audit third-party data. You should consult other sources to evaluate accuracy.
Intel disclaims all express and implied warranties, including without limitation, the implied warranties of merchantability, fitness for a particular purpose, and non-infringement, as well as any warranty arising from course of performance, course of dealing, or usage in trade.
No license (express or implied, by estoppel or otherwise) to any intellectual property rights is granted by this document.
© Intel Corporation. Intel, the Intel logo, and other Intel marks are trademarks of Intel Corporation or its subsidiaries. Other names and brands may be claimed as the property of others.
Q1'22: Technology update – low precision and model optimization
Authors
Alexander Kozlov, Pablo Munoz, Vui Seng Chua, Nikolay Lyalyushkin, Yury Gorbachev, Nilesh Jain
Summary
This quarter we observe a kind of saturation in the popular optimization methods such as pruning and NAS. We reviewed a lot of papers about pruning (structured and unstructured) that do not provide any improvement over existing state-of-the-art or even performing on par. Such works mostly parasitize around the well-known methods. As for the NAS methods, there has been a significant amount of works that claim some theoretical analysis of the existing NAS techniques and their convergence without providing a way how to improve them. We did not include such results in the update.
Papers with notable results
Quantization
- F8NET: FIXED-POINT 8-BITONLY MULTIPLICATION FOR NETWORK QUANTIZATION by Snap Inc. and US universities (https://arxiv.org/pdf/2202.05239v1.pdf).A comprehensive study on applying fixed-point quantization to DNN inference acceleration. Authors provide the analysis on how various fractional length impacts the quantization error for various types of distributions of weights and activation. They also modify the famous PACT method to make it compatible with fixed-point arithmetic. They validate the approach for various models, including MobileNet V1/V2 and ResNet18/50.
- Quantune: Post-training Quantization of Convolutional Neural Networks using Extreme Gradient Boosting for Fast Deployment by Artificial Intelligence Research Laboratory, ETRI (https://arxiv.org/pdf/2202.05048v1.pdf).Authors propose Quantune, a method that combines both XGBoost and transfer learning to seek the optimal quantization configuration. They implemented Quantune based on the Glow compiler stack. The extended Glow provides layer-wise mixed precision and integer-only quantization so it can generate the binary code of the quantized models for various hardware targets, from CPU (x86and ARM) to the integer-only accelerator (VTA). The method outperforms the grid, random, and genetic algorithms by approximately 36.5× with a 0.07-0.65accuracy loss across the six CNN models. The method is available at: https://github.com/leejaymin/qaunt_xgboost.
- Logarithmic Unbiased Quantization: Simple 4-bit Training in Deep Learning by Habana Labs and Department of Electrical Engineering -Technion (https://arxiv.org/pdf/2112.10769v2.pdf).The paper examines the importance of having unbiased quantization in quantized neural network training. It proposes a logarithmic unbiased quantization method to quantize both the forward and backward phase to 4-bit. The method achieves SOTA results in 4-bit training for ResNet-50 on ImageNet and shows that just one epoch of fine-tuning in full precision combined with a variance reduction method significantly improves results.
- Automatic Mixed-Precision Quantization Search of BERT by Samsung Research (https://arxiv.org/pdf/2112.14938v1.pdf).In this paper, authors propose an automatic mixed-precision quantization approach for BERT compression that can simultaneously conduct quantization and pruning in a subgroup-wise level. The method leverages Differentiable Neural Architecture Search to assign scale and precision for parameters in each subgroup automatically, and at the same time pruning out redundant groups of parameters. The method is evaluated on four NLP tasks and shows comparable results.
- LG-LSQ: Learned Gradient Linear Symmetric Quantization by Tsing Hua University and Industrial Technology Research Institute (https://arxiv.org/ftp/arxiv/papers/2202/2202.09009.pdf). The paper proposes a method for accurate low-bit quantization with fine-tuning. It modifies the approach to learn quantization scaling factors by introducing three novelties: 1) the scaling simulated gradient (SSG) for determining the appropriate gradient for the scaling factor of the linear quantizer; 2) the arctangent soft round (ASR) to prevent the gradient from becoming zero, there by solving the discrete problem caused by the rounding process; 3) the minimize discretization error (MDE) method to determine an accurate gradient in backpropagation. All together they help to achieve state-of-the-art results for several models, e.g. fully 4-bit quantized MobileNet v2 on ImageNet within 1% of accuracy drop.
- Standard Deviation-Based Quantization for Deep Neural Networks by McGillUniversity (https://arxiv.org/pdf/2202.12422v1.pdf). Reincarnation of the idea of base-2 logarithmic quantization combined with the idea of standard deviation-based quantization where the floating-point range in the quantizer function is encoded by the estimated σ value and learnable multiplier coefficient. Authors also suggest using two-phase training to increase overall accuracy. The method shows quite good results for low-bit quantization, likeINT4, INT2.
Pruning
- Pruning-aware Sparse Regularization for Network Pruning by Chinese Universities (https://arxiv.org/pdf/2201.06776v1.pdf). Authors analyze sparsity-training-based methods and find that the regularization of unpruned channels is unnecessary and can lead to under-fitting. They propose a pruning method with pruning-aware sparse regularization. It imposes fine-grained sparse regularization on the specific filters selected by a pruning mask. The method reduces more than 51.07%FLOPs on ResNet-50, with a loss of 0.76% in the top-1accuracy on ImageNet. The code is released at https://github.com/CASIA-IVA-Lab/MaskSparsity.
- HRel: Filter Pruning based on High Relevance between Activation Maps and Class Labels by universities of India (https://arxiv.org/pdf/2202.10716.pdf).The paper describes and proposes one more criterion for the selection of prunable filters in CNNs. It is based on information theory and leverages from Mutual Information characteristic of distribution. It is used to compute the so-called “Relevance” of activation maps generated by filters for mini-batch and class labels for the samples in mini-batch. This “Relevance” is used to estimate the importance of the corresponding filters and prune the less important ones. The method achieves comparable results on Image Classification tasks, e.g. 0.68% drop in the top-1 accuracy after pruning 48.66%FLOPs of ResNet-50 on ImageNet.
- SPViT: Enabling Faster Vision Transformers via Soft Token Pruning by US and Switzerland universities (https://arxiv.org/pdf/2112.13890v1.pdf).The paper states that for Vision Transformer architectures token pruning holds a greater computation reduction compared to the compression of other dimensions. It proposes a method that introduces an attention-based multi head token selector and the token packaging technique to achieve per-image adaptive pruning. For lightweight models, the method allows the DeiT-S and DeiT-T to reduce inference latency by 40%-60% within 0.5% accuracy loss.

- EXPLORING STRUCTURALSPARSITY IN NEURAL IMAGE COMPRESSION by Harbin Institute of Technology and Peng Cheng Laboratory (https://arxiv.org/pdf/2202.04595v4.pdf).A practical study on applying the Filter Pruning method to accelerate the inference of Image Compression models. Authors use a simple pruning method based on a learnable per-channel masks. They apply the method to different Image Compression architectures and achieve up to 7× computation reduction and 3×acceleration.
Neural Architecture Search
- AutoDistil : Few-shot Task-agnostic Neural Architecture Search for Distilling Large Language Models by Miscrosoft Research and Pennsylvania State University (https://arxiv.org/pdf/2201.12507v1.pdf).Authors develop a few-shot task-agnostic Neural Architecture Search framework for the NLP domain. They use self-attention distillation to train the SuperLM and demonstrate this to be better than masked language modeling objective for task-agnostic SuperLM training. Experiments in the GLUE benchmark show that the method achieves 62.4% reduction in computational cost and 59.7%reduction in model size over state-of-the-art task-agnostic distillation methods.
- Fast Neural Architecture Search for Lightweight Dense Prediction Networks by European universities (https://arxiv.org/pdf/2203.01994v3.pdf). The paper proposes a multi-objective LDP method for searching for accurate and light weight dense prediction architectures (Segmentation, Depth Estimation, Super Resolution). It uses a new Assisted Tabu Search to enable fast neural architecture search. The method shows comparable or better results of a variety of tasks.
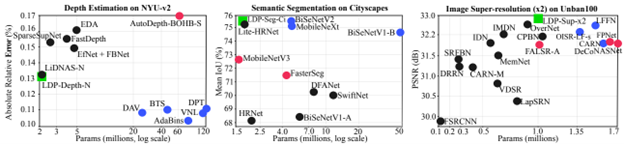
- WPNAS: Neural Architecture Search by jointly using Weight Sharing and Predictor by Huawei and Samsung Research China (https://arxiv.org/pdf/2203.02086v1.pdf). Authors propose a method to jointly use weight-sharing and predictor and use a self-critical policy gradient algorithm with probabilistic sampling to update architecture parameters. They use a few-shot learning-based predictor for subnets and a weakly weight sharing strategy based on the so-called HyperNet which is essentially an RNN-based model that generates offsets for originally shared weights. The method shows comparable to SOTA results on CIFAR and ImageNet datasets.
- ONE-NAS: An Online Neuro Evolution based Neural Architecture Search for Time Series Forecasting by Rochester Institute of Technology (https://arxiv.org/pdf/2202.13471v1.pdf). Authors claim that this work is the first attempt to design and train RNNs for time series forecasting in an online setting. Without any pretraining, the method utilizes populations of RNNs which are continuously updated with new network structures and weights in response to new multivariate input data. The method outperforms traditional statistical time series forecasting, including naive, moving average, and exponential smoothing methods, as well as state-of-the-art online ARIMA strategies.
- BINAS: Bilinear Interpretable Neural Architecture Search by Alibaba (https://arxiv.org/pdf/2110.12399v2.pdf). The paper proposes a bilinear accuracy estimator for architecture search. The bilinear form of the proposed estimator allows the formulation of the latency constrained NAS problem as an Integer Quadratic Constrained Quadratic Programming (IQCQP). Thanks to this, it can be efficiently solved via a simple algorithm with some off-the-shelf components. The method shows comparable results in the close training setup. Code is available at: https://github.com/Alibaba-MIIL/BINAS.
Deep Learning Software
- Neural Network Quantization with AI Model Efficiency Toolkit (AIMET) by Qualcomm (https://arxiv.org/pdf/2201.08442v1.pdf).An overview of DNN optimization toolkit from Qualcomm. The code is open-sourced and contains several state-of-the-art methods from Qualcomm Research.
Deep Learning Hardware
Q2'22: Technology update – low precision and model optimization
Authors
Alexander Kozlov, Alexander Suslov, Pablo Munoz, Vui Seng Chua, Nikolay Lyalyushkin, Yury Gorbachev, Nilesh Jain
Summary
This quarter we observed an increased interest in pruning methods for Transformer-based architectures (BERT, etc.). The main reason for that, as we see it, is a huge success of this architecture in many domains such as NLP, Computer Vision, Speech and Audio processing. NAS methods continue beating handcrafted models on various tasks and benchmarks. As usual, DL model optimization is still a huge area with lots of people involved both from academia and industry.
Papers with notable results
Quantization
- Differentiable Model Compression via Pseudo Quantization Noise by Facebook AI Research (https://arxiv.org/pdf/2104.09987v1.pdf).In this paper, authors propose a DIFFQ method that uses a pseudo quantization noise to approximate quantization at train time, as a differentiable alternative to STE, both with respect to the unquantized weights and number of bits used. With a single penalty level λ, DIFFQ optimizes the number of bits per weight or group of weights to achieve a given trade-off between model size and accuracy. The method outperforms a regular QAT method at a low-bit quantization on different tasks.
- Do All MobileNets Quantize Poorly? Gaining Insights into the Effect of Quantization on Depthwise Separable Convolutional Networks Through the Eyes of Multi-scale Distributional Dynamics by Waterloo Artificial Intelligence Institute (https://arxiv.org/pdf/2104.11849v1.pdf).Authors investigate the impact of quantization on the weight and activation distributional dynamics as information propagates from layer to layer, as well as overall changes in distributional dynamics at the network level. This fine-grained analysis revealed significant dynamic range fluctuations and a “distributional mismatch” between channel wise and layer wise distributions in depth-wise CNNs such as MobileNet that lead to increasing quantized degradation and distributional shift during information propagation. Furthermore, analysis of the activation quantization errors shows that there is greater quantization error accumulation in depth-wise CNNs compared to regular CNNs.
- TENT: Efficient Quantization of Neural Networks on the tiny Edge with Tapered Fixed Point by Neuromorphic AI Lab, University of Texas (https://arxiv.org/pdf/2104.02233v1.pdf).An interesting read for those who are not aware of taper and posit numerical formats. Authors propose a tapered fixed-point quantization algorithm that adapts the numerical format to best represent the layer wise dynamic range and distribution of parameters within a Tiny ML model. They do not provide extensive results but show a superior performance vs. Vanilla fixed-point quantization.
- n-hot: Efficient Bit-Level Sparsity for Powers-of-Two Neural Network Quantization by Sony (https://arxiv.org/pdf/2103.11704v1.pdf).One more method for power-of-two quantization as an alternative to APoT method which also allows reducing the model size. The method uses bit-level sparsity and introduces subtraction of PoT terms. It also applies two-stage long fine-tuning during quantization. This helps to achieve superior results vs. vanilla PoT and APoT methods.
- Network Quantization with Element-wise Gradient Scaling by Yonsei University (https://arxiv.org/pdf/2104.00903v1.pdf).This paper proposes an element-wise gradient scaling (EWGS), a simple alternative to the STE, training a quantized network better than the STE in terms of stability and accuracy. Given a gradient of the discretizer output, EWGS adaptively scales up or down each gradient element, and uses the scaled gradient as the one for the discretizer input to train quantized networks via backpropagation. The method achieves very promising results on CIFAR and ImageNet dataset in low-bit quantization setup (1-2 bits).
- Q-ASR: Integer-only Zero-shot Quantization for Efficient Speech Recognition by Berkeley (https://arxiv.org/pdf/2103.16827v1.pdf).The paper about data-free quantization of the automatic speech recognition models. As usual, the authors use statistics from BatchNorm layers and backpropagation to construct a synthetic dataset. They achieve good results for QuartzNet and JasperDR model that contains BatchNorm.
- Neuro evolution-Enhanced Multi-Objective Optimization for Mixed-Precision Quantization by Intel Labs (https://arxiv.org/pdf/2106.07611v1.pdf).In this paper, authors present a framework for automated mixed-precision quantization that optimizes multiple objectives. The framework relies on Neuro evolution-Enhanced Multi-Objective Optimization (NEMO) to find Pareto optimal mixed-precision configurations for memory and bit-operations objectives. Authors also apply some tricks on top of NEMO to improve the goodness of the Pareto frontier. The method shows state-of-the-art results on several ImageNet models.
- Post-Training Sparsity-Aware Quantization by Israel Institute of Technology(https://arxiv.org/pdf/2105.11010v1.pdf).In this paper, authors propose a complicated quantization scheme that can be done post-training and leverages multiple assumptions, like bit-sparsity of weights and activations, bell-shaped distribution, many zeros in activations. Essentially, the proposed scheme picks the most significant n bits from the 8-bit value representation, while skipping leading zero-value bits. Authors also make projections on the area that requires to implement inference of such quantized models, namely for sysytolic-based architectures and Tensor Cores. They claim SOTA results, for example, for ResNet-50 on ImageNet: -0.18% relative degradation in accuracy, 2× speedup over conventional SA, and an additional 22% SA area overhead. Code is available at https://github.com/gilshm/sparq.
- On the Distribution, Sparsity, and Inference-time Quantization of Attention Values in Transformers by Stony Brook University (https://arxiv.org/pdf/2106.01335v1.pdf).A study about quantization of Transformer-based models (BERT-like). Authors focus on reducing number of bits required to represent information of attentions masks in Self-Attention block. They claim that in many cases it is possible to prune and quantize the mask (to lower bits using non-uniform quantization). The code for the analysis and data are available at https://github.com/StonyBrookNLP/spiqa.
Sparsity
- Accelerated Sparse Neural Training: A Provable and Efficient Method to Find N:M Transposable Masks by Habana and Labs (https://arxiv.org/pdf/2102.08124.pdf). The paper proposed a method to accelerate training using N:M weight sparsity with transposable-fine-grained sparsity mask where the same mask can be used for both forward and backward passes. This mask ensures that both the weight matrix and its transpose follow the same sparsity pattern; thus the matrix multiplication required for passing the error backward can also be accelerated. Experiments show 2x speed-up with no accuracy degradation over vision and language models.
- Post-training deep neural network pruning via layer-wise calibration by Intel (https://arxiv.org/abs/2104.15023v1). The paper introduces a method for accurate unstructured model pruning in the post-training scenario. The method is based on a layer-wise tuning (knowledge distillation) approach when the knowledge from the original model is distilled to the optimizing counterpart in a layer-wise fashion. Authors also propose a way of data-free accurate pruning. The method is available here.
- Carrying out CNN Channel Pruning in a White Box by Tencent and China universities (https://arxiv.org/pdf/2104.11883v1.pdf). The paper proposes a method to model the contribution of each channel to differentiating categories. The authors developed a class-wise mask for each channel, implemented in a dynamic training manner w.r.t. the input image’s category. On the basis of the learned class-wise mask, they perform a global voting mechanism to remove channels with less category discrimination. The method shows comparable results vs. other Filter Pruning criterions but it performance is worse than RL or evolutionary-based method, e.g. LeGR.
- Rethinking Network Pruning— under the Pre-train and Fine-tune Paradigm by Moffett AI (https://arxiv.org/pdf/2104.08682v1.pdf).The paper proposes a method for sparse pruning Transformer-based models. The method exploits the magnitude-based criterium to prune unimportant weights and uses knowledge distillation supervision from the original fine-tuned model. The knowledge distillation is based on MSE loss and connects multiple layers from the original model with the same layers in the pruning counterpart. The method shows good results on the tasks from GLUE benchmark: 95% of weights are pruned while preserving accuracy on most of the tasks.
- MLPruning: A Multilevel Structured Pruning Framework for Transformer-based Models by Berkeley University (https://arxiv.org/pdf/2105.14636v1.pdf). A method to optimize Transformer-based architectures (BERT) that consists of three different levels of structured pruning: 1) Head pruning for multi-head attention; 2) Row pruning for general fully-connected layers; and 3) block-wise sparsity pruning for all weight matrices. To benefit from block sparsity, authors use block-sparse MatMul kernel from Triton SW. They achieve good results on QQP/MNLI/SQuAD, with up to ~3.69xspeedup. Code is available here.
Filter Pruning
- EZCrop: Energy-Zoned Channels for Robust Output Pruning by University of Hong Kong (https://arxiv.org/pdf/2105.03679v2.pdf).The paper introduces a method to interpret channel importance metric in the spatial domain as an energy perspective in the frequency domain. It proposes a computationally efficient FFT-based metric for channel importance. The method slightly outperforms the accuracy of some recent state-of-the-art methods but more computationally efficient at the same time.
- Visual Transformer Pruning by Huawei (https://arxiv.org/pdf/2104.08500v2.pdf).The paper provides a method that identifies the impacts of channels in each layer and then executes pruning accordingly. By encouraging channel-wise sparsity in the Transformer, important channels automatically emerge. A great number of channels with small coefficients can be discarded to achieve a high pruning ratio without significantly compromising accuracy. Authors show that it is possible to prune ~40% of ViT-B/16 model while staying at ~1% of accuracy degradation on ImageNet.
- Convolutional Neural Network Pruning with Structural Redundancy Reduction by The University of Tennessee and 2Sun Yat-sen University (https://arxiv.org/pdf/2104.03438v1.pdf).The paper provides a theoretical analysis of network pruning with statistical modeling from a perspective of redundancy reduction. It also proposes a layer-adaptive channel pruning approach based on structural redundancy reduction which builds a graph for each convolutional layer of a CNN to measure the redundancy existed in each layer (a non-usual approach). The method could prune 55.1% of ResNet-50 FLOPS while staying at ~1% of accuracy drop on ImageNet.
- Model Pruning Based on Quantified Similarity of Feature Maps by University of Science and Technology Beijing (https://arxiv.org/pdf/2105.06052v1.pdf).The paper proposes a new complex criterion to prune filters from any type of convolutional operation. It uses Structural Similarity or Peak Signal to Noise Ratio to find the score of the filters. Despite the fact the paper provides results only on CIFAR dataset, the paper still interesting because it allows pruning filters without fine-tuning while preserving the accuracy. It means that this method can be potentially applied in the post-training scenario to highly redundant models.
- Greedy Layer Pruning: Decreasing Inference Time of Transformer Models by DeepOpinion(https://arxiv.org/pdf/2105.14839v1.pdf).In this paper, authors propose a method to layer pruning (GLP) is introduced to(1) outperform current state of-the-art for layer-wise pruning of Transformer-based architectures without knowledge distillation with long fine-tuning. They focus more on providing an optimization algorithm that requires a modest budget from the resource and price perspective. The method achieves good results on GLUE benchmark and requires only $300 for all 9 tasks.
- Width transfer: on the(in)variance of width optimization by Facebook(https://arxiv.org/pdf/2104.13255.pdf).This work reduces computational overhead in width optimization algorithms(MorphNet, AutoSlim, and DMCP), which in contrast to pruning, improves accuracy by reorganizing width of layers without changing FLOPS. The algorithm uniformly shrinks model's channels and depth, optimizes width on a part of a dataset with smaller images, then the optimized projected network is extrapolated to match original FLOPS and dimensions. Authors can achieve up to 320x overhead reduction without compromising the top-1. Major cons: still the additional cost of width optimization is comparable with initial training time.
Neural Architecture Search
- How Powerful are Performance Predictors in Neural Architecture Search? by Abacus.AI, Bosch and universities(https://arxiv.org/pdf/2104.01177.pdf).The first large-scale study of performance predictors by analyzing 31techniques ranging from learning curve extrapolation, to weight-sharing, supervised learning, “zero-cost” proxies. The code is available at https://github.com/automl/naslib.
- Dynamic-OFA: Runtime DNN Architecture Switching for Performance Scaling on Heterogeneous Embedded Platforms by University of Southampton (https://arxiv.org/pdf/2105.03596v2.pdf). Dynamic-OFA, extends OFA to quickly switch architecture in runtime. Sub-network architectures are sampled from OFA for both CPU and GPU at the offline stage. These architectures have different performance (e.g. latency, accuracy) and are stored in a look-up table to build a dynamic version of OFA without any additional training required. Then, at runtime, Dynamic-OFA selects and switches to optimal sub-network architectures to fit time-varying available hardware resources The approach is up to 3.5x (CPU), 2.4x (GPU) faster for similar ImageNetTop-1 accuracy, or 3.8% (CPU), 5.1% (GPU) higher accuracy at similar latency.
- RHNAS: Realizable Hardware and Neural Architecture Search by Intel Labs (https://arxiv.org/pdf/2106.09180v1.pdf). The paper introduces a NN-HW co-design method that integrates RL-based hardware optimizers with differentiable NAS. It overcomes the challenges associated with sparse validity- a failure point for existing differentiable co-design works. The authors also benchmark RL-based hardware optimizer and use Bayesian hyperparameter optimization to identify the best hyper-parameters for a fair study of a range of standard RL algorithms. The method discovers realizable NN-HW designs with 1.84×lower latency and 1.86× lower energy delay product (EDP) on ImageNet over the default hardware accelerator design.
- NAS-BERT: Task-Agnostic and Adaptive-Size BERT Compression with Neural Architecture Search by MSRA and China universities (https://arxiv.org/pdf/2105.14444v1.pdf). In this paper, authors apply NAS on the pre-training task to search for efficient lightweight NLP models, which can deliver adaptive model sizes given different requirements of memory or latency and apply for different down stream tasks. They also apply block-wise search, progressive shrinking and performance approximation to reduce the search cost and improve the search accuracy. The proposed method demonstrates comparable results on GLUE and SQuAD benchmarks.
- FNAS: Uncertainty-Aware Fast Neural Architecture Search by SenseTime (https://arxiv.org/pdf/2105.11694v3.pdf).This paper proposes FNAS method that consists of three main modules: uncertainty-aware critic, architecture knowledge pool, and architecture experience buffer, to speed up RL-based neural architecture search by ∼10×.Authors show that knowledge of neural architecture search processes can be transferred, which is utilized to improve sample efficiency of reinforcement learning agent process and training efficiency of each sampled architecture. Method shows comparable results on several CV tasks.
- Generative Adversarial Neural Architecture Search by Huawei (https://arxiv.org/pdf/2105.09356v2.pdf).Quite unusual approach to NAS based on the idea of generative adversarial training. The method iteratively fits a generator to previously discovered to architectures, thus increasingly focusing on important parts of a large search space. Authors propose an adversarial learning approach, where the generator is trained by reinforcement learning based on rewards provided by a discriminator, thus being able to explore the search space without evaluating a large number of architectures. This method can be used to improve already optimized baselines found by other NAS methods, including EfficientNet and ProxylessNAS.
- LightTrack: Finding Lightweight Neural Networks for Object Tracking via One-Shot Architecture Search by MSRA and China universities (https://arxiv.org/pdf/2104.14545v1.pdf).In this paper, authors propose a method uses neural architecture search (NAS)to design more lightweight and efficient object tracker. It can find trackers that achieve superior performance compared to handcrafted SOTA trackers while using much fewer model Flops and parameters. For example, on Snapdragon 845Adreno GPU, LightTrack runs 12× faster than Ocean, while using 13×fewer parameters and 38× fewer Flops. Code is available here.
Other Methods
- A Full-stack Accelerator Search Technique for Vision Applications by Google Brain (https://arxiv.org/pdf/2105.12842.pdf).This paper proposes a hardware accelerator search framework (FAST) that defines a broad optimization environment covering key design decisions within the hardware-software stack, including hardware data path, software scheduling, and compiler passes such as operation fusion and tensor padding. The method shows promising results on improving Perf/TDP metric when optimizing several CV workloads.
Deep Learning Software
- MLPerf Inference v1.0 has been released: https://mlcommons.org/en/news/mlperf-inference-v10/.
- Nvidia included OpenVINO in the Triton Inference Server as the CPU inference SW. See the MLPerf Inferece v1.0 in the blogpost.
- HAGO by OctoML, Amazon and Washington University (https://arxiv.org/pdf/2103.14949v1.pdf)- automated post-training quantization framework. It is built on top of TVM and provides a set of general quantization graph transformations based on a user-defined hardware specification (similar to OpenVINO POT) and implements a search mechanism to find the optimal quantization strategy.
- Archai by Microsoft (https://github.com/microsoft/archai) is a platform for Neural Network Search (NAS)that allows you to generate efficient deep networks for your applications.
Deep Learning Hardware
- Visual Search Engine by Moffett AI (https://moffett.ai/visualsearch/)-sparse processing on Xilinx FPGA
- NAAS: Neural Accelerator Architecture Search by MIT (Han Lab) (https://arxiv.org/pdf/2105.13258v1.pdf).The paper proposes a NAAS method that holistically searches the neural network architecture, accelerator architecture and compiler mapping in one optimization loop. NAAS composes highly matched architectures together with efficient mapping. As a data-driven approach, NAAS rivals the human design Eyeriss by 4.4×EDP reduction with 2.7% accuracy improvement on ImageNet under the same computation resource, and offers 1.4× to 3.5× EDP reduction than only sizing the architectural hyper-parameters.
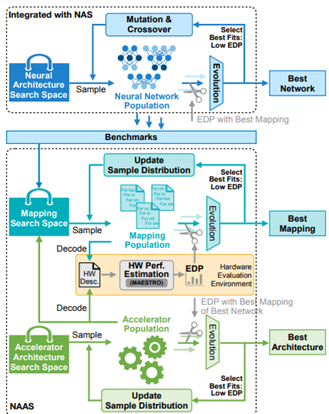
Q3'22: Technology update – low precision and model optimization
Authors
Alexander Kozlov, Pablo Munoz, Vui Seng Chua, Nikolay Lyalyushkin, Yury Gorbachev, Nilesh Jain
Summary
We would characterize this quarter as “let’s go beyond INT8inference”. This quote is about “ANT”, a paper that you can find in theHighlights and that introduces 4-bit data type for accurate model inferencewhich fits well with the current HW architectures. There is also a lot of hypearound FP8 precisions that are already available in the latest Nvidia Hopperarchitecture and are being planned to be added into the next generations of Intel HW.
Highlights
- ANT: Exploiting Adaptive Numerical Data Type for Low-bit Deep Neural Network Quantization by Microsoft Research and universities of China and US (https://arxiv.org/pdf/2208.14286.pdf). A very interesting read about a new data type for model inference which authors called flint and which combines the advantages of float and int. They proposed an encoding/decoding scheme for this type as well as the implementation of computational primitives that are based on the existing DL HW architectures. Authors also evaluate the computational efficiency of the type and show the accuracy of using it for inference on a diverse set of models.
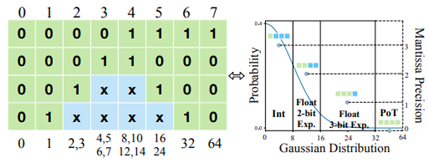
- LLM.int8(): 8-bit Matrix Multiplication for Transformers at Scale by the collaboration of Facebook, HuggingFace and universities (https://arxiv.org/pdf/2208.07339v1.pdf). The main idea of the proposed method is to split matrix multiplication operation (MatMul) which is the main operation of Transformer-based models into two separate MatMuls. The one is quantized to 8-bits and another is kept to FP16 precision. The result of both operations is summed. This mixed-precision decomposition for MatMul is based on a magnitude criterium. The authors achieved good results in accelerating of Transformer models on Nvidia GPUs. Code is available at: https://github.com/TimDettmers/bitsandbytes.
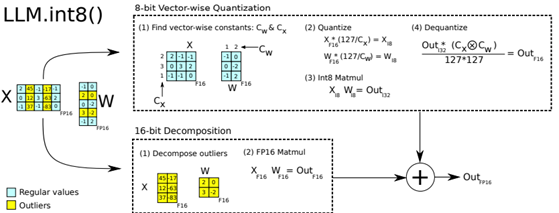
- CPrune: Compiler-Informed Model Pruning for Efficient Target-Aware DNN Execution by University of Colorado Boulder and Electronics and Telecommunications Research Institute (https://arxiv.org/pdf/2207.01260.pdf). The paper proposes a method, which incorporates the information extracted during the compiler optimization process into creating a target-oriented compressed model fulfilling accuracy requirements. This information also reduces the search space for parameter tuning. The code is available at: https://github.com/taehokim20/CPrune.
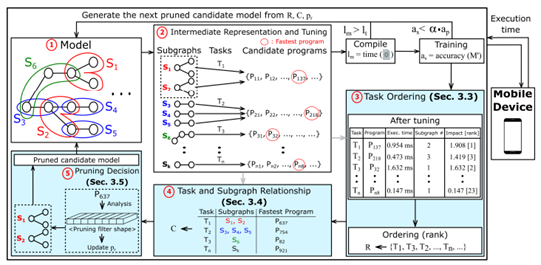
- UniNet: Unified Architecture Search with Convolution, Transformer, and MLP by MMLab and SenseTime (https://arxiv.org/pdf/2207.05420.pdf). Authors construct the search space and study the learnable combination of convolution, transformer, and MLP integrating it into an RL-based search algorithm. They conclude that: (1) placing convolutions in the shallow layers and transformers in the deep layers, (2) allocating a similar amount of FLOPs for both convolutions and transformers, and (3) inserting a convolution-based block to downsample for convolutions and a transformer-based block for transformers. The best model achieves 87.4% top1 on ImageNet outperforming Swin-L. Code will be available at https://github.com/Sense-X/UniNet.

Papers with notable results
Quantization
- I-ViT: Integer-only Quantization for Efficient Vision Transformer Inference by universities of China (https://arxiv.org/pdf/2207.01405.pdf). Authors propose efficient approximations of non-linear functions of Transformer architecture, namely Softmax, GeLU, and LayerNorm. These approximations are used to get the integer-only computational graph. They applied the proposed method to several vision Transformer models and get close to 4x speedup when going from FP32 to INT8 computations. To get the quantized model authors used a straightforward quantization-aware training method. For all the models they got a little worse or even better accuracy.
- Sub 8-Bit Quantization of Streaming Keyword Spotting Models for Embedded Chipsets by Alexa, Amazon (https://arxiv.org/pdf/2207.06920.pdf). Some practical work on the quantization of the Keyword Spotting language models. Authors used a 2-stage QAT algorithm: for the 1st-stage, they adapt a non-linear quantization method on weights, while for the 2nd-stage, we use linear quantization methods on other components of the network. The method has been used to improve the efficiency on ARM NEON architecture, where authors obtain up to 3 times improvement in CPU consumption and more than 4 times improvement in memory consumption.
- CADyQ: Content-Aware Dynamic Quantization for Image Super-Resolution by universities of South Korea and Nvidia (https://arxiv.org/pdf/2207.10345.pdf). A practical study of applying low bit-width mixed-precision quantization to Super Resolution models. Authors proposed a pipeline of selecting different bit-width for each patch and layer of the model by adding a lightweight bit selector module that is conditioned on the estimated quantization sensitivity. They also introduce a new to find a better balance between the computational complexity and overall restoration performance. The method shows good accuracy and performance results measured on T4 GPU using 8-bit and 4-bit arithmetic. Code is available at: https://github.com/Cheeun/CADyQ.
- Bitwidth-Adaptive Quantization-Aware Neural Network Training: A Meta-Learning Approach by universities of South Korea (https://arxiv.org/pdf/2207.10188.pdf). The paper proposes a method of bitwidth-adaptive quantization aware training (QAT) where meta-learning is effectively combined with QAT by redefining meta-learning tasks to incorporate bitwidths. The method trained model to be quantized to any candidate bitwidth with minimal inference accuracy drop. The paper provides some insight on how optimization can be done in the scenarios such as Iterative Learning, task adaptation, etc.
- Efficient Activation Quantization via Adaptive Rounding Border for Post-Training Quantization by Microsoft Research and universities of Shanghai (https://arxiv.org/pdf/2208.11945.pdf). The authors explore the benefits of adjusting rounding schemes of providing a new perspective for the post-training quantization. They design a border function that produces unbiased elementwise errors and makes it can adjust to specific activations to generate adaptive rounding schemes. They experiment with ImageNet models and get promising results for 4-bit and even 2-bit quantization schemes in the post-training setup.
- FP8 Quantization: The Power of the Exponent by Qualcomm AI Research (https://arxiv.org/pdf/2208.09225.pdf). This paper investigated the PTQ and QAT efficacy of FP8 schemes by varying bit-length of Mantissa (M) and Exponent(E) and exponent bias flexibility (per channel/tensor) across a wide range of convolutional and transformer topologies and tasks. The authors concluded that multi-FP8 formats are required for translating FP-trained deep networks due to model-specific optimal dynamic range and precision trade-off. Networks (BERT, ViT, SalsaNext, HRNet) with outlying dynamic ranges require more exponent bits whereas convnets require more mantissa bits for precision. FP8 formats are also more friendly for PTQ as compared to Int8.
Pruning
- CAP: instance complexity-aware network pruning by universities of China (https://arxiv.org/pdf/2209.03534.pdf). Authors exploit the difference of instance complexity between the datase samples to boost the accuracy of pruning method. They introduce a new regularizer on the soft masks of filters, the masks of important filters are pushed towards 1 and those of redundant filters are pushed towards 0, thus a sweet spot can be easily found to separate the two parts of filters. It helped to achieve compelling results in sparsity, e.g. prune 87.75% FLOPs of ResNet50 with 0.89% top-1 accuracy loss.
- Sparse Attention Acceleration with Synergistic In-Memory Pruning and On-Chip Recomputation by Google Brain and University of California (https://arxiv.org/pdf/2209.00606.pdf). The paper proposes a HW accelerator that leverages the inherent parallelism of ReRAM crossbar arrays to compute attention scores in an approximate manner. It prunes the low attention scores using a lightweight analog thresholding circuitry within ReRAM, enabling it to fetch only a small subset of relevant data to on-chip memory. To mitigate potential negative repercussions for model accuracy, the accelerator re-computes the attention scores for the few-fetched data in digital. The combined in-memory pruning and on-chip recompute of the relevant attention scores enables transforming quadratic complexity to a merely linear one. This yields 7.5x speedup and 19.6x energy reduction when total 16KB on-chip memory is used.
- OPTIMAL BRAIN COMPRESSION: A FRAMEWORK FOR ACCURATE POST-TRAINING QUANTIZATION AND PRUNING by IST Austria & Neural Magic (https://arxiv.org/pdf/2208.11580.pdf). The paper introduces a compression framework that covers both weight pruning and quantization in a post-training setting. At the technical level, the approach is based on the first exact and efficient realization of the classical Optimal Brain Surgeon (OBS) framework at the scale of modern DNNs, which we further extend to cover weight quantization. Experimental results show it can enable the accurate joint application of both pruning and quantization at post-training.
Neural Architecture Search
- You Only Search Once: On Lightweight Differentiable Architecture Search for Resource-Constrained Embedded Platforms by universities of Singapore (https://arxiv.org/pdf/2208.14446.pdf). The paper introduces an accurate predictor to estimate the latency of the architecture (𝑎𝑟𝑐ℎ). The arch is encoded with a sparse matrix 𝛼 ∈ {0, 1} 𝐿×𝐾, where the element indicates that the 𝑘-th operator is reserved for the 𝑙-th layer of 𝑎𝑟𝑐ℎ. The latency predictor is an MLP model (3 FC layers) where the input is a flattened 𝛼. The authors also propose a lightweight differentiable search method to reduce the optimization complexity to the single-path level. They compare with other popular methods such as OFA, MNAS, FBNAS, etc., and report superior results. The code is available here: https://github.com/stepbuystep/LightNAS.
- SenseTime Research 2 Shanghai AI Lab 3Australian National University by SenseTime Research Shanghai AI Lab and Australian National University (https://arxiv.org/pdf/2207.13955.pdf). Authors employ NAS for searching for a representative model based on the cosFormer architecture. They propose a new usage of attention, namely mixing Softmax attention and linear attention in the Transformer, and define a new search space for attention search in the NAS framework. The proposed mixed attention achieves a better balance between accuracy and efficiency, i.e., having comparable performance to the standard Transformer while maintaining good efficiency.
- NASRec: Weight Sharing Neural Architecture Search for Recommender Systems by Meta AI, Duke University, and University of Houston (https://arxiv.org/pdf/2207.07187.pdf). Authors propose a paradigm to scale up automated modeling of recommender systems. The method establishes a supernet with minimal human priors, overcoming data modality and architecture heterogeneity challenges in the recommendation domain. Authors advance weight-sharing NAS to the recommendation domain by introducing single-operator any-connection sampling, operator balancing interaction modules, and post-training fine-tuning. The method outperforms both manually crafted models and models discovered by NAS methods with smaller search cost.
- Tiered Pruning For Efficient Differentiable inference-aware Neural Architecture search by NVidia (https://arxiv.org/pdf/2209.11785.pdf). Authors propose three pruning techniques to improve the cost and results of Differentiable Neural Architecture Search (DNAS). Instead of evaluating all possible parameters, they evaluate just two which converge to a single optimal one (e.g. to optimal number of channels in Inverted Residual Blocks). Progressively remove blocks from the search space which are rarely chosen during SuperNet training. Skip connection is not present in the search space at the beginning of search and is inserted after removing the penultimate block of the layer in its place. The proposed algorithm establishes a new state-of-the-art Pareto frontier for NVIDIA V100 in terms of inference latency for ImageNet Top-1 image classification accuracy.
- When, where, and how to add neurons to ANNs (https://arxiv.org/pdf/2202.08539v2.pdf). Authors propose an novel approach to search for neural architectures using structural learning, and in particular neurogenesis. A framework is introduced in which triggers and initializations are used for studying the various facets of neurogenesis: when, where, and how to add neurons during the learning process. The neurogenesis strategies, termed Neural Orthogonality (NORTH*), combine, “layer-wise triggers and initializations based on the orthogonality of activations or weights to dynamically grow performant networks that converge to an efficient size”. The paper offers relevant insights that can be used in more broader Neural Architecture Search frameworks.
Other
- On-Device Training Under 256KB Memory by MIT (https://arxiv.org/pdf/2206.15472.pdf). Authors propose Quantization-Aware Scaling to calibrate the gradient scales and stabilize quantized training. To reduce the memory footprint, they introduce Sparse Update to skip the gradient computation of less important layers and sub-tensors. The algorithm is implemented by a lightweight training system, Tiny Training Engine, which prunes the backward computation graph to support sparse updates and offload the runtime auto-differentiation to compile time. Method is available at: https://github.com/mit-han-lab/tinyengine.
Deep Learning Software
- Efficient Quantized Sparse Matrix Operations on Tensor Cores (https://arxiv.org/pdf/2209.06979.pdf). A high-performance sparse-matrix library for low-precision integers on Tensor cores. Magicube supports SpMM and SDDMM, two major sparse operations in deep learning with mixed precision. Experimental results on an NVIDIA A100 GPU show that Magicube achieves on average 1.44x (up to 2.37x) speedup over the vendor-optimized library for sparse kernels, and 1.43x speedup over the state-of-the-art with a comparable accuracy for end-to-end sparse Transformer inference.
- A BetterTransformer for Fast Transformer Inference. PyTorch introduced the support of new operations that improve inference of Transformer models and can “take advantage of sparsity in the inputs to avoid performing unnecessary operations on padding tokens”.
Deep Learning Hardware
- NVIDIA, Arm, and Intel Publish FP8 Specification for Standardization as an Interchange Format for AI (blog post). The precision is already available in the latest Nvidia Hopper architecture and is planned in all the Intel HW.