Docker
Deploy AI Workloads with OpenVINO™ Model Server across CPUs and GPUs
Authors: Xiake Sun, Kunda Xu
1. Introduction
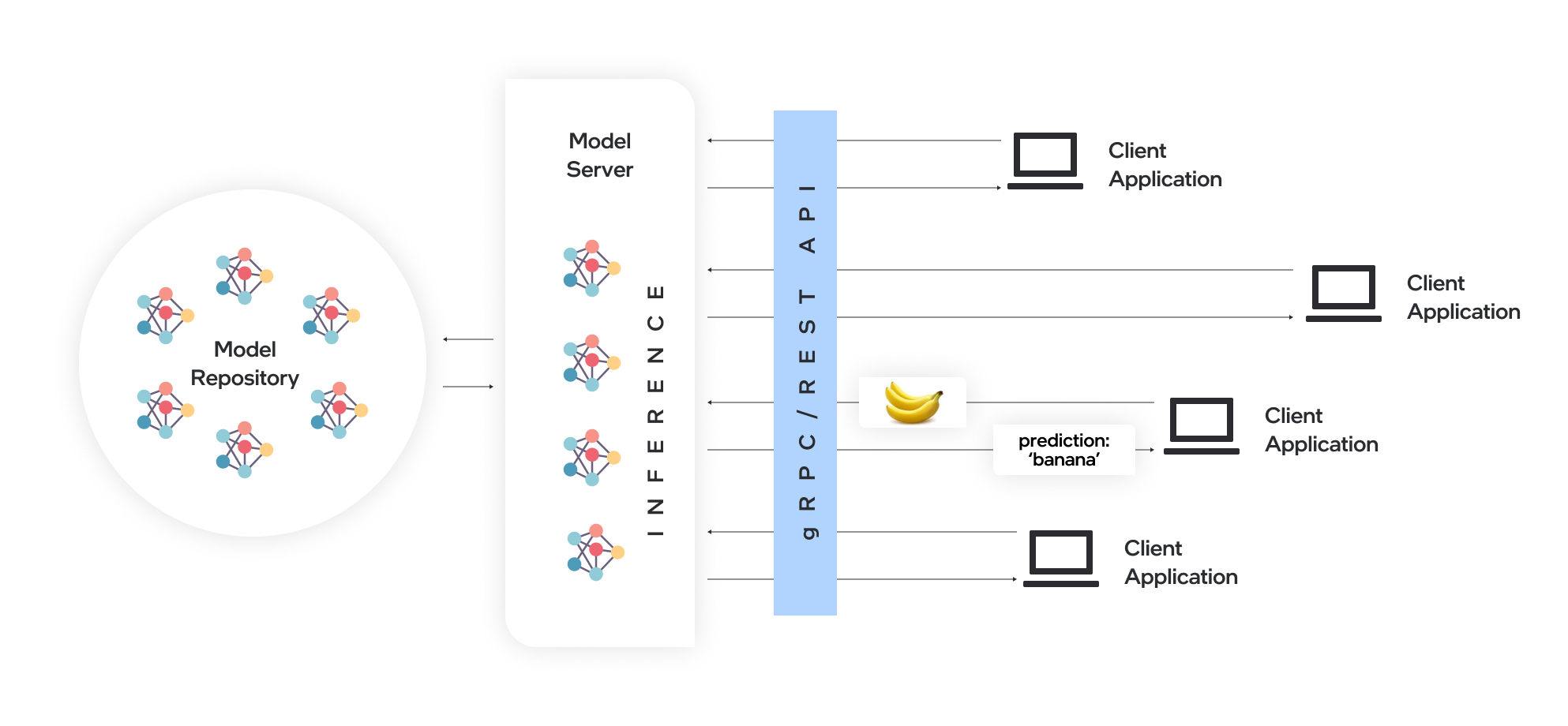
OpenVINO™ Model Server (OVMS) is a high-performance system for serving models. Implemented in C++ for scalability and optimized for deployment on Intel® architectures, the model server uses the same architecture and API as TensorFlow Serving and KServe while applying OpenVINO™ for inference execution. Inference service is provided via gRPC or REST API, making deploying new algorithms and AI experiments easy.
Docker is the recommended way to deploy OpenVINO™ Model Server. Pre-built container images are available on Docker Hub and Red Hat Ecosystem Catalog.
In this blog, we will introduce how to leverage OpenVINO™ Model Server to deploy AI workload across various hardware platforms, including Intel® CPU, Intel® GPU, and Nvidia GPU.
2. OpenVINO™ Model Server Pre-built Docker Image for Intel® CPU
Pull the latest pre-built OVMS docker image hosted in Docker Hub:
Verify OVMS docker image and OpenVINO™ backend version:
Here is an example output of the command line above:

Download a model and create an appropriate directory structure. For example, a person-vehicle-bike-detection model from Intel’s Open Model Zoo:
where a model directory structure looks like that:

After the model repository preparation, let’s start OVMS to host a person-vehicle-bike-detection-2000 model in the Model Server with Intel® CPU as target device.
The parameter “--target_device CPU” specified workload to allocate on Intel® CPU. “--port 30001” set up the gRPC server port as 30001, and “--rest_port 30001” set up the REST server port as 30002. The parameter “--model_path” specified the model directory path in the docker image, while “--model_name” specified which model to host in the model server.
3. Build OpenVINO™ Model Server Benchmark Client
OpenVINO™ Model Server provides a useful tool - Benchmark Client to generate traffic and measure the performance of the model served in OpenVINO™ Model Server. In this blog, you could use Benchmark Client to verify OpenVINO™ model server functionality quickly.
To build the docker image and tag it as benchmark_client as follow:
Here is an example to use benchmark_client to generate 8 requests and send them via gRPC API, then receive the severed model performance data:
In the output, "window_netto_frame_rate" measures the overall performance of a service - how many frames per second the model server processed. Please note, model serving example above was set up with default parameters, see the performance tuning section for more details.
4. Build OpenVINO™ Model Server from Source Code
Download the model server source code as follows:
OVMS provides a “Makefile” to build the docker image with environment parameters, which you can pass via the command line for the building process.
- BASE_OS: base OS docker image used to build OVMS docker image, current supported values are “ubuntu” (by default) and “redhat”.
- OV_USE_BINARY: control whether to use a pre-built OpenVINO™ binary package for building OVMS docker image. If "OV_USE_BINARY=1", OVMS use a pre-built OpenVINO™ binary package. If "OV_USE_BINARY=0", OpenVINO™ will be built from source code during OVMS building process.
- DLDT_PACKAGE_URL: If "OV_USE_BINRAY=1", "DLDT_PACKAGE_URL" is used to set the URL path to the pre-built OpenVINO™ binary package
- GPU: control whether to enable OVMS support for Intel® GPU. By default, “GPU=0” disables OVMS support for Intel® GPU. If "GPU=1", OVMS support for intel® GPU will be enabled.
- NVIDIA: control whether to enable OVMS support for Nvidia GPU. By default, "NVIDIA=0" disables OVMS support for Nvidia GPU. If "NVIDIA=1", OVMS support for Nvidia GPU will be enabled, which requires building OpenVINO from the source code.
- OV_SOURCE_BRANCH: If "OV_USE_BINARY=0", "OV_SOURCE_BRANCH" is used to set the target branch or commit hash of OpenVINO source code. The default value is “master”
- OV_CONTRIB_BRANCH: If "NVIDIA=1", "OV_CONTRIB_BRANCH" is used to set the target branch or commit hash of OpenVINO contrib source code. The default value is “master"
Here is an example of building OVMS with the "releases/2022/3" branch of OpenVINO™ GitHub source code with target device Intel® CPU.
Built docker image will be available in the host as “openvino/model_server:latest”.
5. Build OpenVINO™ Model Server with Intel® GPU Support
Since OpenVINO™ 2022.3 release, OpenVINO™ added full support for Intel’s integrated GPU, Intel’s discrete graphics cards, such as Intel® Data Center GPU Flex Series, and Intel® Arc™ GPU for DL inferencing workloads in the intelligent cloud, edge, and media analytics workloads. OpenVINO™ Model Server 2022.3 also added support for Intel® GPU. The pre-built OpenVINO™ Model Server docker image with GPU driver for Intel® GPU is available in Docker Hub:
Here is an example of building OVMS with Intel® GPU support based on the OpenVINO™ source code:
The default GPU driver (version 22.8 for RedHat 8.7 or version 22.35 for Ubuntu 20.04) will be installed during the building process. Built docker image will be available in the host as “openvino/model_server:latest-gpu”.
Here is an example to launch the OVMS docker image with Intel® GPU as target device:
The parameter “--target_device GPU” specified workload to allocate on Intel® GPU. The parameter “--device /dev/dri” is used to pass the device context. The parameter “--group-add=$(stat -c"%g" /dev/dri/render\* | head -n 1) -u $(id -u):$(id -g)” is used to ensure the model server process security context account with correct permissions to run inference on Intel® GPU.
Here is an example to verify the severed model performance on Intel® GPU with benchmark_client:
6. Build OpenVINO™ Model Server with Nvidia GPU Support
OpenVINO™ Model Server can also support Nvidia GPU cards by using NVIDIA plugin from the GitHub repo openvino_contrib. Here is an example of building OVMS with Nvidia GPU support step by step:
First, pull the Nvidia docker base image with the GPU driver, e.g.,“docker.io/nvidia/cuda:11.8.0-runtime-ubuntu20.04”, please ensure to install same GPU driver version in the local host environment.
Install Nvidia Container Toolkit to expose the GPU driver to docker and restart docker.
Build OVMS docker image with Nvidia GPU support.“NVIDIA=1” enables to build OVMS with Nvidia GPU support, and “OV_USE_BINARY=0” enables building OpenVINO from the source code. Besides, “OV_SOURCE_BRANCH=releases/2022/3” refer to the OpenVINO™ GitHub "releases/2022/3" branch, while “OV_CONTRIB_BRANCH=releases/2022/3” refer to the OpenVINO contrib GitHub "releases/2022/3" branch.
Built docker image will be available in the host as “openvino/model_server-cuda:latest”.
Here is an example to launch the OVMS docker image with Nvidia GPU as target device:
The parameter “--target_device NVIDIA” is specified to allocate workload on NVIDIA GPU. The parameter “--gpu all” flag is used to access all GPU resources available in the host system.
Here is an example to verify the severed model performance on Nvidia GPU with benchmark_client:
7. Migration from Triton Inference Server to OpenVINO™ Model Server
KServe, as a robust and extensible cloud-native model server for Kubernetes, is widely adopted by model servers including Triton Inference Server. Since the 2022.3 release, OpenVINO™ Model Server added KServer API that supports REST and gRPC calls. Therefore, OVMS with Nvidia GPU support is fully compatible to receive requests from Triton Inference Client and run inference on Nvidia GPU.
Here is an example to pull the Triton Inference Server docker image:
Then you could use perf_client tools in the docker image to send generated workload as requests to OVMS via KServe API with gRPC port, then receive measured performance data on Nvidia GPU.
The simple example above shows how smoothly developers can migrate their own AI service workload from Triton Inference Server to OpenVINO™ Model Server without any change from the client.
Intel® DL Streamer Optimize Media-AI pipeline on Intel® Data Center Flex dGPU by Docker
Authors Kunda Xu, Wenyi Zou
Introduction
In this blog is about How to use DL-streamer to build a complete Media-AI pipeline (Including: Video Access, Media Decode, AI Inference, Media Encode and Result Export). And the pipeline will be accelerated by OpenVINO™ and optimize to run on Flex dGPU(Intel® Data Center Flex dGPU)
Requirement
- DL-streamer
Intel® Deep Learning Streamer (Intel® DL Streamer)Pipeline Framework is an easy way to construct media analytics pipelines using Intel® Distribution of OpenVINO™ Toolkit. It leverages the open source media framework GStreamer to provide optimized media operations and Deep Learning Inference Engine from OpenVINO™ Toolkit to provide optimized inference.
- OpenVINO
OpenVINO™ is an open-source toolkit for optimizing and deploying AI inference which can boost deep learning performance in computer vision, automatic speech recognition, natural language processing and other common task.
- Docker (Optional)
Docker is an open-source platform that enables developers to build, deploy, run, update, and manage containers—standardized, executable components that combine application source code with the operating system (OS) libraries and dependencies required to run that code in any environment.
Install DL-Streamer and OpenVINO™ via Docker
Images for Intel® Data Center GPU Flex Series
Images 2023.0.0-ubuntu22-gpu682* are intended for Intel® Data Center GPU Flex Series and include
3. Drivers for Intel® Data Center GPU Flex Series, drivers version 682.14
Two images are listed below, images -devel additionally contain samples and development files
Runtime image that includes GStreamer* Pipeline Framework elements, elements built with Intel® oneAPI DPC++/C++ Compiler
Developer image that builds on runtime image containing samples, development files and a model downloader, built with Intel® oneAPI DPC++/C++ Compiler
Taking “dlstreamer:2023.0.0-ubuntu22-gpu682-dpcpp” docker images as a sample to show how to pull the docker image from docker hub.
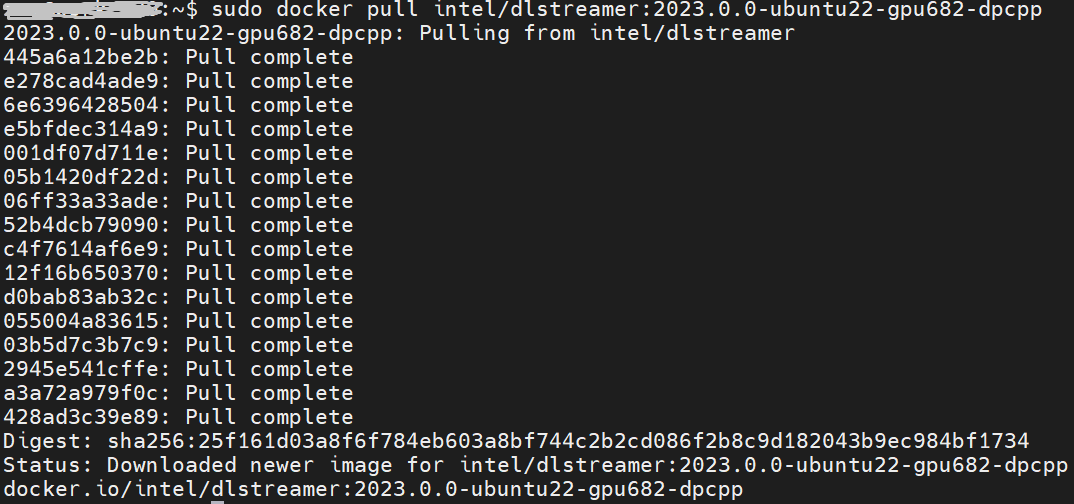
DL-Streamer Media-AI pipeline quick start example
Make sure the pre-requirement had already installed, there is a very basic introduction to using object detection models(yolov5) to build a DL-streamer pipeline.
Step 1.Download video and yolov5s model file
Download video
Download yolov5s-416_INT8 model from pipeline-zoo-models
Step 2.Enter Docker and copy the files into docker container
Create and enter the docker container
Open another terminal for file copy into container ,copy video and model into docker container
Step 3. Run an object detection Media-AI pipeline
By the following script, we can run pipeline the Media-AI objection detection on the Flex dGPU in the docker container.
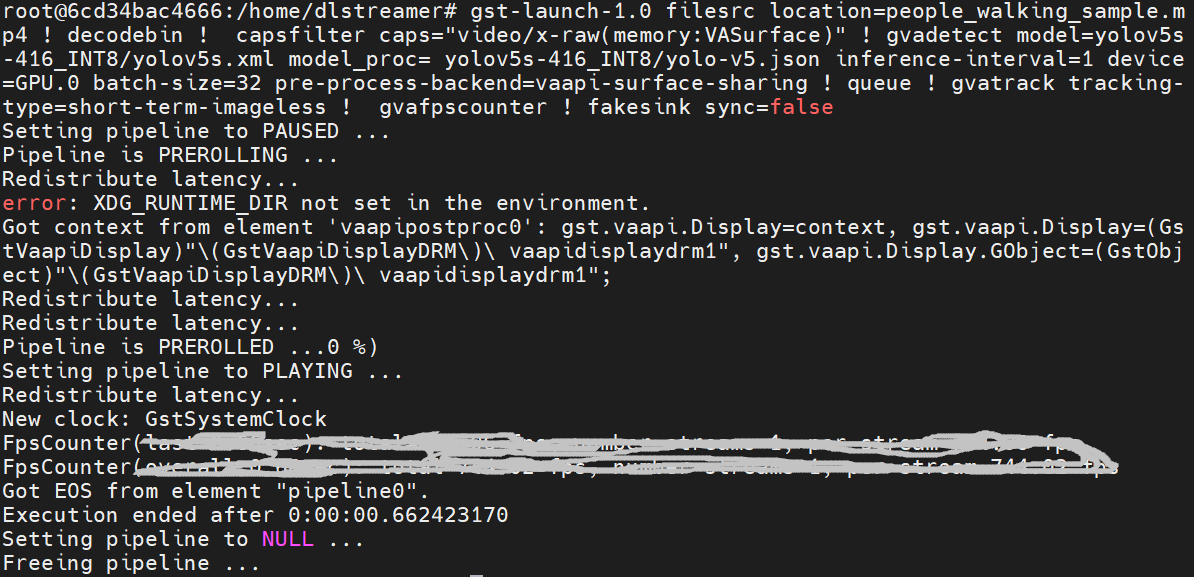
If want to encode the detection result and save as video file, can use the follow script
The encoded video file will save in the container and can be copied out in new terminal.

PS. Instruction about DL-streamer CLI parameter
decodebin: Auto-magically constructs a decoding pipeline using available decoders and demuxers via auto-plugging.
vaapipostproc: Consists in various post processing algorithms to be applied to VA surfaces. For e.g. scaling, deinterlacing (bob, motion-adaptive, motion-compensated), noise reduction or sharpening.
gvadetect: Performs object detection on a full-frame or region of interest (ROI) using object detection models such as YOLO v3-v5, MobileNet-SSD, Faster-RCNN etc. Outputs the ROI for detected objects.
gvatrack: Performs object tracking using zero-term, zero-term-imageless, or short-term-imageless tracking algorithms. Zero-term tracking assigns unique object IDs and requires object detection to run on every frame. Short-term tracking allows to track objects between frames, there by reducing the need to run object detection on each frame. Imageless tracking forms object associations based on the movement and shape of objects, and it does not use image data.
gvafpscounter: Measures frames per second across multiple streams in a single process.
Tuning Tips
Users can refer the different platform using case which were supported by OpenVINO™ and the device profiling API to realize performance tuning of your inference program between CPU, iGPU, dGPU. It will also be helpful to developer finding out the place where has the potential space of performance improvement.
Build OpenVINO™ Docker Image on CentOS 7
Authors: Xiake Sun, Su Yang
For OpenVINO™ 2022.1 on CentOS 7.6, we provide the dockerfile based on the docker_ci and usage instructions in this blog.
At present, OpenVINO™ officially only provides a docker image of OpenVINO™ 2021.3 for CentOS 7 on DockerHub. To deploy a docker image with the OpenVINO™ toolkit, OpenVINO™ provides the DockerHub CI named docker_ci. However, docker_ci doesn’t support CentOS 7.
Here are the steps to build the OpenVINO™ 2022.1.0 Docker Image on CentOS 7.6 with Python 3.6. In this instruction, we use Python 3.6 in the docker image as an example. If upgrading the version, the user needs to solve problems with yum and related tools.
Setup of OpenVINO™
0. Build OpenVINO™ 2022.1.0 with CMake 3.18.4 and GCC 8.3.1
Install docker on CentOS 7 and setup proxy (if any):
https://docs.docker.com/engine/install/centos/
https://docs.docker.com/engine/install/linux-postinstall/#manage-docker-as-a-non-root-user
https://docs.docker.com/config/daemon/systemd/
1. Download CMake 3.18.4
2. Install devtoolset-8 and Setup Environment
3. Build OpenVINO™ 2022.1.0 docker image with CMake 3.18.4 and GCC 8.3.1
4. Compress pre-built OpenVINO™ 2022.1.0 install package as .tgz
Setup of Docker Image
1. Build OpenVINO™ dev docker image for CentOS 7
2. Start Docker image
3. Test docker image with OpenVINO™ tools