Automatic Device Selection and Configuration with OpenVINO™
OpenVINO empowers developers to write deep learning application code once and deploy it on a wide range of Intel hardware with best-in-class performance. Previously, significant effort had to be spent configuring inference pipelines to squeeze optimal performance out of target hardware, and the effort had to be repeated whenever the application was ported to a new platform. The new Auto Device Plugin (AUTO) and automatic configuration features in OpenVINO make it easier for developers to unlock performance on multiple hardware targets without needing to spend time optimizing their application pipeline.
When an OpenVINO application is deployed in a system, the Auto Device Plugin automatically selects the best hardware target to inference the model with. OpenVINO then automatically configures the application to use optimal pipeline parameters based on the hardware capabilities and model size. Developers no longer need to write code for detecting hardware devices and explicitly configuring batch and stream parameters. High-level configuration is provided through performance hints that allow a developer to prioritize their application for either high throughput or minimal latency. AUTO and automatic device configuration make applications hardware-agnostic, allowing them to easily be ported to new hardware without any code changes.
The diagram in Figure 1 shows how OpenVINO’s features automatically configure an application for optimal performance, regardless of the target hardware. When the deep learning model is loaded, AUTO creates a transparent plugin interface to the available processor devices and automatically selects the most suitable device. OpenVINO configures the batch size and number of processing streams based on the selected hardware target, and the Auto-Batching feature automatically groups incoming data into optimally sized batches. AUTO and automatic configuration operate independently from each other, so developers can use either or both in their application.

AUTO and automatic configuration are available starting in the 2022.1 release of OpenVINO Runtime. To use these features, simply install OpenVINO Runtime on the target hardware. The API uses AUTO by default if no processor device is specified when loading a model. Set a “throughput” or “latency” performance hint when loading the model, and the API automatically configures the inference pipeline. Read on to learn more about AUTO, automatic configuration, performance hints, and how to use them in your application.
Automatic Device Selection
Auto Device Plugin (AUTO) is a “virtual” device that provides a transparent interface to physical devices in the system. When an application is initialized, AUTO discovers the available processors and accelerators in the system (CPUs, integrated GPUs, discrete GPUs, VPUs) and selects the best device, based on a default device priority list or an optional user-provided priority list. It creates an interface between the application and device that executes inference requests in an optimized fashion. It enables an application to always achieve optimal performance in a system without the developer having to know beforehand what devices are available in the system.

Key Features and Benefits
Simple and flexible application deployment
Previously, developers needed to know details about target hardware and configure their application specifically for each device. AUTO removes the need to write dedicated code for specific devices. This enables an application to be written once and deployed to any supported hardware. It also allows the application to run on newer generations of hardware as they are released: the developer only needs to compile the application with the latest version of OpenVINO to run it on new hardware. This provides an instant increase in performance with little development time.
Configurability
AUTO provides a configuration interface that is easy to use at a high level while still providing flexibility. Developers can simply specify “AUTO” as the device to tell the application to select the best device for the given model. They can also control which device is selected by providing a device candidate list and setting priorities for each device.
Developers can also use performance hints to configure their application for latency or throughput. When the performance hint is throughput, OpenVINO will create more streams for parallel inferencing to achieve maximum processing bandwidth. In latency mode, OpenVINO creates fewer streams to utilize as many resources as possible to complete each inference quickly. Performance hints also help determine the optimal batch size for inferencing; this is discussed further in the “Performance Hints” section of this document.
Improved first-inference latency
In applications that use accelerated processors like GPUs or VPUs, the time to first inference may be higher than average because it takes time to compile and load the deep learning model into the accelerator. AUTO solves this problem by starting the first inference with the CPU, which has minimal latency and no delays. As the first inference is being performed, AUTO continues to compile and load the model for the selected accelerator device, and then transparently switches over to that device when it is ready. This significantly reduces time to first inference, and is beneficial for applications that require immediate inference results on startup.
How Automatic Device Selection Works
To choose the best device for inference, AUTO discovers which hardware targets are available in the system and matches the model to the best supported device, using the following process:
- AUTO discovers which devices are available using the Query Device API. The query reads an internal file that lists installed hardware plugins, confirms the hardware modules are present by communicating with them through drivers, and returns a list of available devices in the system.
- AUTO checks the precision of the input model by reading the model file.
- AUTO selects the best available device in the device priority table (shown in Table 1 below) that is capable of supporting the model’s precision.
- AUTO attempts to compile the model on the selected device. If the model doesn’t compile (for example, if the device doesn’t support all the operations required by the model), AUTO tries to compile it on the next best device until compilation is successful. The CPU is the final fallback device, as it supports all operations and precisions.
By default, AUTO uses the device priority list shown in Table 1. Developers can customize the table to provide their own device priority list and limit the devices that are available to run inferencing. AUTO will not try to run inference on devices that are not provided in the device list.
Table 1. Default AUTO Device Priority List
As mentioned, AUTO reduces the first inference latency by compiling and loading the model to the CPU first. As the model is loaded to the CPU and first inference is performed, AUTO steps through the rest of the process for selecting the device and compiling the model to that device. This way, devices that require a long time for model compilation do not impede inference as the application is being initialized.
AUTO also provides a model priority feature that enables developers to control which models are loaded to which devices when there are multiple models running on a system with multiple devices. Developers can set “MODEL_PRIORITY” as “HIGH”, “MEDIUM”, or “LOW” to configure which models should be allocated to the best resource. This allows developers to ensure models that are critical for an application are always loaded to the fastest device for processing, while less critical models are loaded to slower devices.
For example, consider a medical imaging application with models for segmenting and/or classifying injuries in X-ray images running on a system that has both a GPU and a CPU. The segmentation model is set to HIGH priority because it takes more processing power to inference, while the classification model is set to MEDIUM priority. If both models are loaded at the same time, the segmentation model will be loaded to the GPU (the higher priority device) and the classification model will be loaded to the CPU (the lower priority device). If only the classification model is loaded, it will be loaded to the GPU since the GPU isn’t occupied by the higher-priority model.
Automatic Device Configuration
The performance of a deep learning application can be improved by configuring runtime parameters to fully utilize the target hardware. There are several factors to take into consideration when optimizing inference for a certain device, such as batch size and number of streams. (See Runtime Inference Optimizations in OpenVINO documentation for more information.) The optimal configuration for these parameters depends on the architecture and memory of the target hardware, and they need to be re-determined when porting an application from one device to another.
OpenVINO provides features that automatically configure an application to use optimal runtime parameters to achieve the best performance on any supported hardware target. These features are enabled through performance hints, which allow a user to specify whether their application should be optimized for latency or throughput. The automatic configuration eliminates the time and effort required to determine optimal configurations. It makes it simple to port to new devices or write one application to work on multiple devices. OpenVINO’s automatic configuration features currently work with CPU and GPU devices, and support for VPUs will be added in a future release.
Performance Hints
OpenVINO allows users to provide high-level "performance hints" for setting latency-focused or throughput-focused inference modes. These performance hints are “latency” and “throughput.” The hints cause the runtime to automatically adjust runtime parameters, such as number of processing streams and inference batch size, to prioritize for reduced latency or high throughput. Performance hints are supported by CPU and GPU devices, and a future release of OpenVINO will add support for VPUs.
The performance hints do not require any device-specific settings and are portable between devices. Parameters are automatically configured based on whichever device is being used. This allows users to easily port applications between hardware targets without having to re-determine the best runtime parameters for the new device.
Latency performance hint
Latency is the amount of time it takes to process a single inference request and is usually measured in milliseconds (ms). In applications where data needs to be inferenced and acted on as quickly as possible (such as autonomous driving), low latency is desirable. When applications are run with the “latency” performance hint, OpenVINO determines the optimal number of parallel inference requests for minimizing latency while still maximizing the parallelization capabilities of the hardware. It automatically sets the number of processing streams to achieve the best latency.
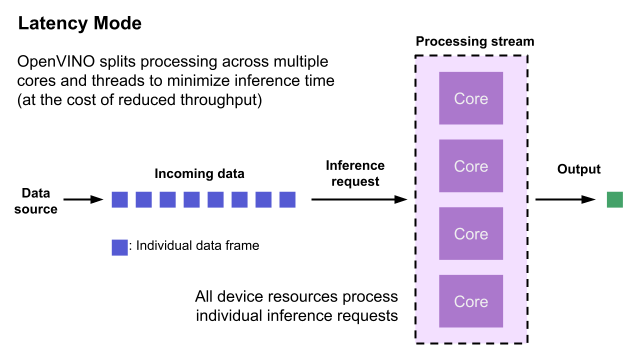
To achieve the fastest latency, the processor device should process only one inference request at a time so all the compute resources are available for calculation. However, devices with multiple cores (such as multi-socket CPUs or multi-tile GPUs) can deliver multiple streams with the same latency as they would with a single stream. OpenVINO automatically checks the compute demands of the model, queries capabilities of the device, and selects the number of streams to be the minimum required to get the best latency. For CPUs, this is typically one stream for each socket. For GPUs, it’s typically one stream per tile.
Throughput performance hint
Throughput is the amount of data an inferencing pipeline can process at once, and it is usually measured in frames per second (FPS) or inferences per second. In applications where large amounts of data needs to be inferenced simultaneously (such as multi-camera video streams), high throughput is needed. To achieve high throughput, the runtime should focus on fully saturating the device with enough data to process. When applications are run with the “throughput” performance hint, OpenVINO maximizes the number of parallel inference requests to utilize all the threads available on the device. On GPU, it automatically sets the inference batch size to fill up the GPU memory available.
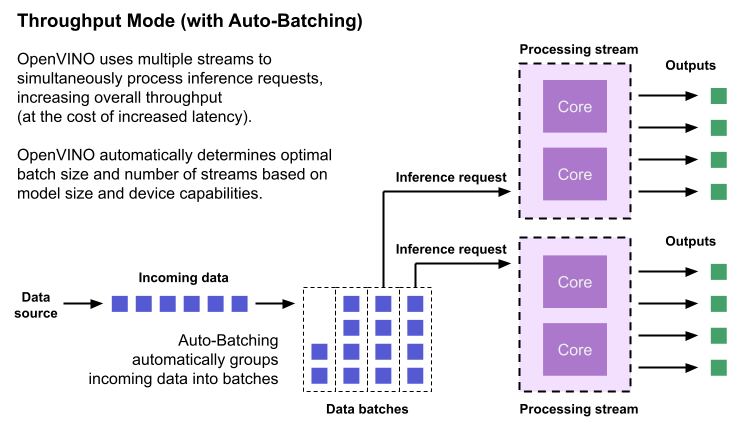
To configure the runtime for high throughput, OpenVINO automatically sets the number of streams to use based on the architecture of the device. For CPUs, it creates as many streams as there are cores available. For GPUs, it uses a combination of batch size and parallel streams to fully utilize the GPU’s memory and compute resources. To determine the optimal configuration on GPUs, OpenVINO will first check if the network supports batching. If it does, it loads the network with a batch size of one, determines how much memory is used for the single-batch network, and then scales the batch size and streams up to fill the entire GPU.
Batch size can also be explicitly specified in code when the model is loaded. This can be useful in applications where the number of incoming data sources is known and constant. For example, in an application that processes four camera streams, specify a batch size of four so that each set of frames from the cameras is processed in a single inference request. More information on batch configuration is given in the Auto-Batching section below.
Auto-Batching
Auto-Batching is a new feature of OpenVINO that performs on-the-fly grouping of data inference requests in an application. As the application makes individual inference requests, Auto-Batching transparently collects them into a batch. When the batch is full (or when a timeout limit is reached), OpenVINO executes inference on the whole batch. In short, it takes care of batching data efficiently so the developer doesn’t have to worry about it.
The Auto-Batching feature is controlled by the configuration parameter “ALLOW_AUTO_BATCHING”, which is enabled by default. Auto-Batching is activated when all of the following are true:
- ALLOW_AUTO_BATCHING is true
- The model is loaded to the target device with the throughput performance hint
- The target device supports batching (such as GPU)
- The model topology supports batching
When Auto-Batching is activated, OpenVINO automatically determines the optimal batch size for an application based on model size and hardware capabilities. Developers can also explicitly specify the batch size when loading the model. While the inference pipeline is active, individual inference requests are gathered into a batch and then executed when the batch is full.
Auto-Batching also has a timeout feature that is configurable by the developer. If there aren’t enough individual requests collected within the developer-specified time limit, batch execution will fall back to just using individual inference requests. For example, a developer may specify a timeout limit of 500 ms and a batch size of 16 for a video processing inference pipeline. Once 16 frames are gathered, a batch inference request is made. If only 13 frames arrive before the 500 ms timeout is hit, the application will perform individual inference requests on each of the 13 frames. While the timeout feature makes the pipeline robust to interruptions in incoming data, hitting the timeout limit heavily reduces the performance. To avoid this, developers should make sure there is enough incoming data to fill the batch within the time limit in typical conditions.
Auto-Batching, when combined with OpenVINO's automatic configuration features that determine optimal batch size and number of streams, provides a powerful benefit to the developer. The developer can utilize the full power of the target device with only using one line of code. Best of all, when an application is used on a different device, it will automatically reconfigure itself to achieve optimal performance with zero effort from the developer.
How to Use AUTO and Performance Hints
Using AUTO and automatic configuration with performance hints only requires one line of code. The functionality centers around the “ie.compile_model” method, which is used to compile a model and load it into device memory. The method accepts various configuration parameters that allow a user to provide high-level control over the pipeline.
Here are several Python examples showing how to configure a model and pipeline with the ie.compile_model method. The first example also shows how to import the OpenVINO Core model, initialize it, and read a model before calling ie.compile_model.
Example 1. Load a model on AUTO device
Example 2. Load a model on AUTO device with performance hints
Example 3. Provide a list of device candidates which AUTO may use when loading a model
Example 4. Load multiple models with HIGH, MEDIUM, and LOW priorities
Example 5. Load a model to GPU and use Auto-Batching with an explicitly set batch size
For a more in-depth example of how to use AUTO and automatic configuration, please visit the Automatic Device Selection with OpenVINO Jupyter notebook in the OpenVINO notebooks repository. It provides an end-to-end example that shows:
- How to download a model from Open Model Zoo and convert it to OpenVINO IR format with Model Optimizer
- How to load a model to AUTO device
- The improvement in first inference latency when using AUTO device
- How to perform asynchronous inferencing on data batches in throughput or latency mode
- A performance comparison between throughput and latency modes
The OpenVINO Benchmark App also serves as a useful tool for experimenting with devices and batching to see how performance changes under various configurations. The Benchmark App supports automatic device selection and performance hints for throughput or latency.
Where to Learn More
To learn more please visit auto device plugin and automatic configuration pages in OpenVINO documentation. They provide more information about how to use and configure them in an application.
OpenVINO also provides an example notebook explaining how to use AUTO and showing how it improves performance. The notebook can be downloaded and run on a development machine where OpenVINO Developer Tools have been installed. Visit the notebook at this link: Automatic Device Selection with OpenVINO.
To learn more about OpenVINO toolkit and how to use it to build optimized deep learning applications, visit the Get Started page. OpenVINO also provides a number of example notebooks showing how to use it for basic applications like object detection and speech recognition on the Tutorials page.