Model Enable
InternVL2-4B model enabling with OpenVINO
Authors: Hongbo Zhao, Fiona Zhao
Introduction
InternVL2.0 is a series of multimodal large language models available in various sizes. The InternVL2-4B model comprises InternViT-300M-448px, an MLP projector, and Phi-3-mini-128k-instruct. It delivers competitive performance comparable to proprietary commercial models across a range of capabilities, including document and chart comprehension, infographics question answering, scene text understanding and OCR tasks, scientific and mathematical problem solving, as well as cultural understanding and integrated multimodal functionalities.
You can find more information on github repository: https://github.com/zhaohb/InternVL2-4B-OV
OpenVINOTM backend on InternVL2-4B
Step 1: Install system dependency and setup environment
Create and enable python virtual environment
conda create -n ov_py310 python=3.10 -y
conda activate ov_py310
Clone the InternVL2-4B-OV repository from github
git clonehttps://github.com/zhaohb/InternVL2-4B-OV
cd InternVL2-4B-OV
Install python dependency
pip install -r requirement.txt
pip install --pre -U openvino openvino-tokenizers --extra-index-url https://storage.openvinotoolkit.org/simple/wheels/nightly
Step2: Get HuggingFace model
huggingface-cli download --resume-download OpenGVLab/InternVL2-4B --local-dir InternVL2-4B--local-dir-use-symlinks False
cp modeling_phi3.py InternVL2-4B/modeling_phi3.py
cp modeling_intern_vit.py InternVL2-4B/modeling_intern_vit.py
Step 3: Export to OpenVINO™ model
python test_ov_internvl2.py -m ./InternVL2-4B -ov ./internvl2_ov_model -llm_int4_com -vision_int8 -llm_int8_quan -convert_model_only
Step4: Simple inference test with OpenVINO™
python test_ov_internvl2.py -m ./InternVL2-4B -ov ./internvl2_ov_model -llm_int4_com -vision_int8-llm_int8_quan
Question: Please describe the image shortly.

Answer:
The image features a close-up view of a red panda resting on a wooden platform. The panda is characterized by its distinctive red fur, white face, and ears. The background shows a natural setting with green foliage and a wooden structure.
Here are the parameters with descriptions:
python test_ov_internvl2.py --help
usage: Export InternVL2 Model to IR [-h] [-m MODEL_ID] -ov OV_IR_DIR [-d DEVICE] [-pic PICTURE] [-p PROMPT] [-max MAX_NEW_TOKENS] [-llm_int4_com] [-vision_int8] [-llm_int8_quant] [-convert_model_only]
options:
-h, --help show this help message and exit
-m MODEL_ID, --model_id MODEL_ID model_id or directory for loading
-ov OV_IR_DIR, --ov_ir_dir OV_IR_DIR output directory for saving model
-d DEVICE, --device DEVICE inference device
-pic PICTURE, --picture PICTURE picture file
-p PROMPT, --prompt PROMPT prompt
-max MAX_NEW_TOKENS, --max_new_tokens MAX_NEW_TOKENS max_new_tokens
-llm_int4_com, --llm_int4_compress llm int4 weight scompress
-vision_int8, --vision_int8_quant vision int8 weights quantize
-llm_int8_quant, --llm_int8_quant llm int8 weights dynamic quantize
-convert_model_only, --convert_model_only convert model to ov only, do not do inference test
Supported optimizations
1. Vision model INT8 quantization and SDPA optimization enabled
2. LLM model INT4 compression
3. LLM model INT8 dynamic quantization
4. LLM model with SDPA optimization enabled
Summary
This blog introduces how to use the OpenVINO™ python API to run the pipeline of the Internvl2-4B model, and uses a variety of acceleration methods to improve the inference speed.
moondream2 model enabling with OpenVINO
Introduction
moondream2 is a small vision language model designed to run efficiently on edge devices. Although the model has a small number of parameters, it provides high-performance visual processing capabilities. It can quickly understand and process input images and respond to user queries. The model was developed by VikhyatK and is released under the permissive Apache 2.0 license, allowing for commercial use.
You can find more information on github repository: https://github.com/zhaohb/moondream2-ov
OpenVINOTM backend on moondream2
Step 1: Install system dependency and setup environment
Create and enable python virtual environment
conda create -n ov_py310 python=3.10 -y
conda activate ov_py310
Clone themoondream2-ov repository from gitHub
git clone https://github.com/zhaohb/moondream2-ov
cd moondream2-ov
Install python dependency
pip install -r requirement.txt
pip install --pre -U openvino openvino-tokenizers --extra-index-url https://storage.openvinotoolkit.org/simple/wheels/nightly
Step 2: Get HuggingFace model
git lfs install
git clone https://hf-mirror.com/vikhyatk/moondream2
git checkout 48be9138e0faaec8802519b1b828350e33525d46
Step 3: Export OpenVINO™ models and simple inference test with OpenVINO™
python3 test_ov_moondream2.py -m /path/to/moondream2 -o /path/to/moondream2_ov
Question: Describe this image.

Answer:
The image shows a modern white desk with a laptop, a lamp, and a notebook on it, set against a gray wall and a wooden floor.
SDPA Enabling for Custom Model
Authors: Su Yang, Xiake Sun, Fiona Zhao
Introduction
To enable the SDPA fusion on GPU, we firstly need to convert model IR with SDPA Op.
Create new class SdpaAttention in the modeling_ custom_model.py using torch.scaled_dot_product_attention. This Pytorch Op could be matched and converted into OpenVINO SDPA Op.
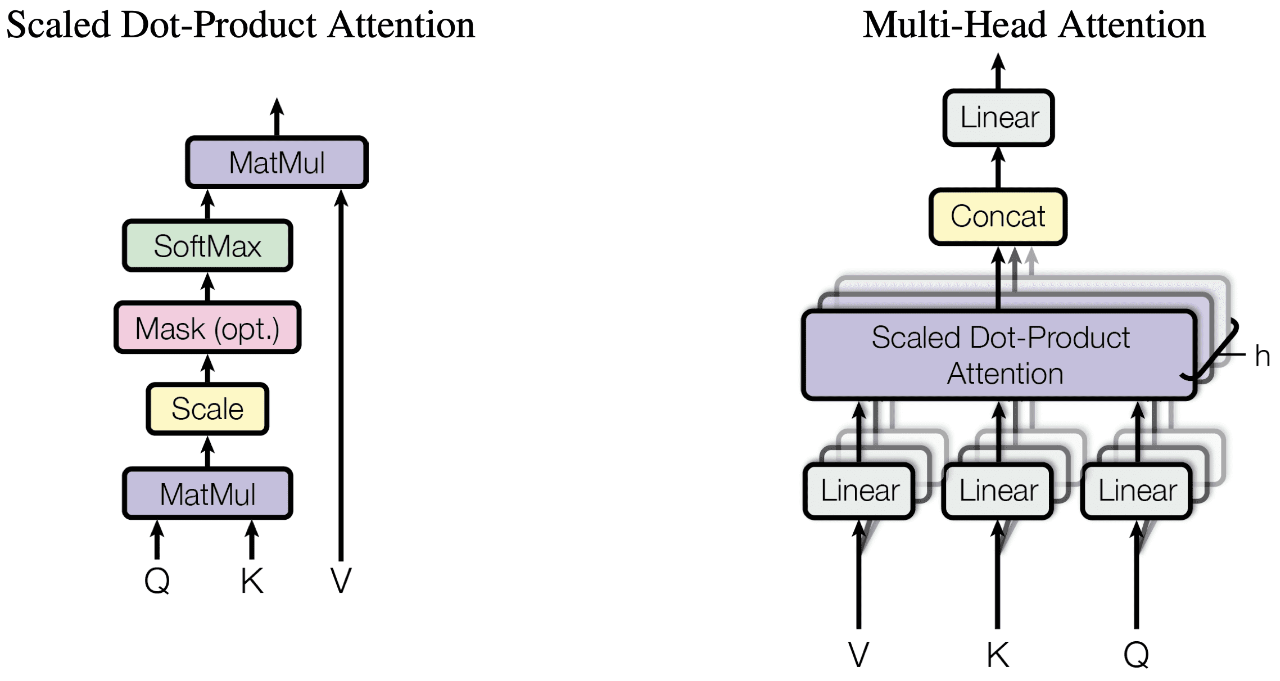
Refer to Phi3SdpaAttention, this module is inherited from Phi3Attention as the weights of the module stay untouched. The only changes are on the forward pass to adapt to SDPA API.
torch.scaled_dot_product_attention
From the equivalent implementation, the target is to replace the related Pytorch Ops(like softmax, matmul and dropout) with torch.nn.functional.scaled_dot_product_attention.

For some custom model, the equivalent code in is as follow:
The corresponding implementation of scaled_dot_product_attention:
SDPA’s Scaling factor with different head_size
For the Pytorch model with different head_size, this scaled_dot_product_attention need to be modified with the scaling factor.
The SDPA Operator has already implemented. The SDPA fusion on GPU supports for head size =128 at OV24.2 release.
The OV24.3 release relax SDPA head size limitations for LLMs from 128 only to a range of 64 to 256, thanks to Sergey’s PR.
Usage
Replace the original modeling_custom_model.py with the new script (with SdpaAttention) in the Pytorch model folder.
Notice:
- After converting model again, check the SDPA layers (“aten::scaled_dot_product_attention”) in the OV IR .xml file.
- Double check the OpenVINO executable graph for the SDPA enabling.
- Don’t forget to check the accuracy of the Pytorch model inference, after any modification with modeling_custom_model.py.
Conclusion
In this blog, we introduce how to use torch.scaled_dot_product_attention to enable the SDPA for custom model.
Performance improvement with SDPA on MTL iGPU is depended on the model structure. SDPA enabling for the custom model is the base for further optimization like Page Attention.
Enable OpenVINO™ Optimization for WeNet
Introduction
The WeNet model provides two-pass approach to unify streaming and non-streaming end-to-end (E2E) speech recognition which is widely used with various HW platforms. In this blog, we provide the OpenVINO™ optimization for WeNet on Intel® platforms.
The public WeNet project is referenced from: wenet-e2e/wenet
The WeNet model can be considered as a pipeline which is split into 3 parts for decoder, CTC and encoder. Refer the model structure in below picture:

We implement the wrapper function of Automatic Speech Recognition (ASR) model class with OpenVINO™ runtime API programming for these 3 models’ data preparation and inference. Please refer the integrated OpenVINO™ optimization in official project: wenet-e2e/wenet/runtime/openvino
OpenVINO™backend on WeNet
In this project, you do not require to download OpenVINO™ and build the library with WeNet project manually. It’s already fully integrated with OpenVINO™ runtime library for downloading, program compiling and linking. If your operating system is not one of OpenVINO™ runtime library supported, the script will download OpenVINO™ source from Github, and build with CPU plugin to support.
At present, this repository already optimized and validated by OpenVINO™ 2022.3.0 version. Check the operating system which can support OpenVINO™ runtime library directly:
- Windows* 10
- CentOS 7, Red Hat* Enterprise Linux* 8
- Ubuntu* 18.04, 20.04
- Debian 9.13 for X86
- macOS* 10.15
Step 1: Get pretrained ONNX model (Optional)
If you already have the exported ONNX model for WeNet test, you can skip this step.
For users to get pretrained model from WeNet project, you can refer this link:
https://github.com/wenet-e2e/wenet/blob/main/docs/pretrained_models.en.md
Export to 3 ONNX models, including encoder.onnx, ctc.onnx and decoder.onnx by export_onnx_cpu script.
Step 2: Convert ONNX model to OpenVINO™ Intermediate Representation (IR)
Make sure your python environment already installed OpenVINO™ runtime library.
Convert these three ONNX models into IR by OpenVINO™ Model Optimizer command:
Step 3: Build WeNet with OpenVINO™ backend
Please refer system requirement to check if the hardware platform available by OpenVINO™. It will download and install OpenVINO™ library during the CMake configuration.
Some users may cannot easily download OpenVINO™ binary package from server due to firewall or proxy issue. If you failed to download by CMake script, you can download OpenVINO™ package by your selves and put the package to below path:
If you already have OpenVINO™ runtime which is manually built before the WeNet building, you can put the runtime library to below path:
Step 4: Simple inference test
You may run the inference test like below with the speech input audio file (.wav) and model unit file (.txt):
The information of OpenVINO™ integration and results will be print out:
Extend OpenVINO™ to run PyTorch models with custom operations
Authors: Anna Likholat, Nico Galoppo
The OpenVINO™ Frontend Extension API lets you register new custom operations to support models with operations that OpenVINO™ does not support out-of-the-box. This article explains how to export the custom operation to ONNX, add support for it in OpenVINO™, and infer it with the OpenVINO™ Runtime.
The full implementation of the examples in this article can be found on GitHub in the openvino_contrib.
Export a PyTorch model to ONNX
Let's imagine that we have a PyTorch model which includes a new complex multiplication operation created by user (this operation was taken from DIRECT):
We'd like to export the model to ONNX and preserve complex multiplication operations as single fused nodes in the ONNX model graph, so that we can replace those nodes with custom OpenVINO operations down the line. If we were to export MyModel which directly calls the function above from its forward method, then onnx.export() would inline the PyTorch operations into the graph. This can be observed in the figure of the exported ONNX model below.

To prevent inlining of native PyTorch functions during ONNX export, we can wrap the function in a sub-class of torch.autograd.Function and define a static symbolic method. This method should return ONNX operators that represent the function's behavior in ONNX. For example:
You can find the full implementation of the wrapper class here: complex_mul.py
So now we're able to export the model with custom operation nodes to ONNX representation. You can reproduce this step with the export_model.py script:
The resulting ONNX model graph now has a single ComplexMultiplication node, as illustrated below:
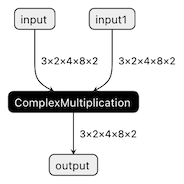
Enable custom operation for OpenVINO with Extensibility Mechanism
Now we can proceed with adding support for the ComplexMultiplication operation in OpenVINO. We will create an extension library with the custom operation for OpenVINO. As described in the Custom OpenVINO Operations docs, we start by deriving a custom operation class from the ov::op::Op base class, as in complex_mul.hpp.
1. Implement Operation Constructors
Implement the default constructor and constructors that optionally take the operation inputs and attributes as parameters. (code)
2. Override methods
2.1 validate_and_infer_types() method
Validates operation attributes and calculates output shapes using attributes of the operation: complex_mul.cpp.
2.2 clone_with_new_inputs() method
Creates a copy of the operation with new inputs: complex_mul.cpp.
2.3 has_evaluate() method
Defines the contstraints for evaluation of this operation: complex_mul.cpp.
2.4 evaluate() method
Implementation of the custom operation: complex_mul.cpp
3. Create an entry point
Create an entry point for the extension library with the OPENVINO_CREATE_EXTENSIONS() macro, the declaration of an extension class might look like the following:
This is implemented for the ComplexMultiplication operation in ov_extension.cpp.
4. Configure the build
Configure the build of your extension library using CMake. Here you can find the template of such script:
Also see an example of the finished CMake script for module with custom extensions here: CMakeLists.txt.
5. Build the extension library
Next we build the extension library using CMake. As a result, you'll get a dynamic library - on Linux it will be called libuser_ov_extensions.so, after the TARGET_NAME defined in the CMakeLists.txt above.
Deploy and run the custom model
You can deploy and run the exported ONNX model with custom operations directly with the OpenVINO Python API. Before we load the model, we load the extension library into the OpenVINO Runtime using the add_extension() method.
Now you're ready to load the ONNX model, and infer with it. You could load the model from the ONNX file directly using the read_model() method:
Alternatively, you can convert to an OpenVINO IR model first using Model Optimizer, while pointing at the extension library:
Note that in this case, you still need to load the extension library with the add_extension() method prior to loading the IR into your Python application.
The complete sequence of exporting, inferring, and testing the OpenVINO output against the PyTorch output can be found in the custom_ops test code.
See Also
OpenVINO™ optimize Fairseq S2T model
OpenVINO™ Optimize Fairseq S2T Model
Introduction
Fairseq is a sequence modeling toolkit that allows researchers and developers to train custom models for translation, summarization, language modeling and other text generation tasks.
There are 2 steps to generate model ready for OpenVINO™ acceleration:
1. Use torch.export.onnx function convert the “.pt” model to “.onnx” model;
2. Use OpenVINO™ MO toolkit convert the “.onnx” model to “IR” model.
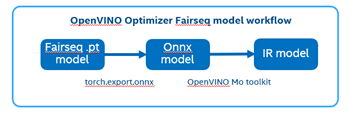
The following graph is the Fairseq framework inference workflow, it defines the model structure by “Model Config”, composes “Model Definition List” through multiple subgraph models, and dynamically loads the submodules in the model inference runtime.
Such as in the S2T task, model consists of two parts: Encoder and Decoder.
· Encoder is for extracting feature information from audio file.
· Decoder is for decoding the feature information to generate text information.
Fairseq Inference workflow
The length of audio information will affect the length of the feature information, and the length of the feature information will affect the Decoder submodule loop’s times. Therefore, the structure of the S2T model is dynamically defined according to the length of the input audio.

To optimize Fairseq framework model there’re 4 challenges need to be solved:
- Fairseq define submodules for various function, include variable in model layer define.
- Model structure is dynamically loaded in runtime and can’t export a whole torch model graph.
- Encoder and Decoder part models’ input shapes are dynamic, depending on input data size.
- Decoder part loop times depends by input sequence lengths.
OpenVINO™ optimize Fairseq workflow
So that we should use some optimization tricks to solve these problems, to make sure the pipeline optimized by OpenVINO™.
- Divide model into Encoder and Decoder two parts, and separately export to onnx model,
- Because of the model structure define by input seq_len, should export dynamic shape onnx model.
- Convert onnx to IR model by OpenVINO™ MO toolkit.
- Replace the Fairseq S2T task pipeline Encoder and Decoder into IR model.
- Loading Inference Engine to run pipeline the pipeline on OpenVINO™.
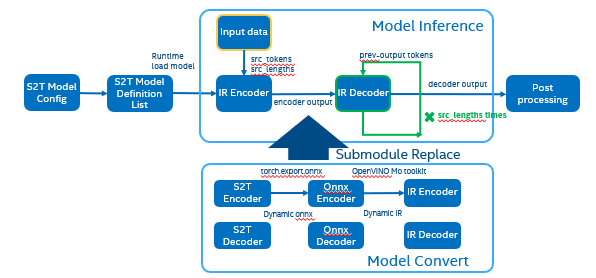
Requirement
- Fairseq is a sequence modeling toolkit that allows researchers and developers to train custom models for translation, summarization, language modeling and other text generation tasks
- OpenVINO™ is an open-source toolkit for optimizing and deploying AI inference which can boost deep learning performance in computer vision, automatic speech recognition, natural language processing and other common task.
- Python version >=3.8
- PyTorch version >=1.10.0
Reference: GitHub: Fairseq-OpenVINO
Quick Start Demo
Step 1. Install fairseq and requirement
#Install OpenVINO™
Reference: Install OpenVINO by source code for Linux
Reference: Install OpenVINO by release package
Step 2. Download audio file and pre-train model file
In this blog we refer the “S2T Example: STon CoVoST” as sample, Preparation dataset and pre-train model can follow the Fairseq original step. Also, you can use “torch audio” to convert audio file to build customer dataset.
Step 3. Modify code to export onnx
Torch model export to onnx, We should adjust the contents in fairseq/sequence_generator.py +781 line "self.save_onnx = True" , +782 line "self.openvino_engine = False" The encoder.onnx and decoder.onnx will save in models
Encoder part model export to dynamic onnx

Decoder part model export to dynamic onnx

Step 4. Convert Model to IR
Convert encoder.onnx and decoder.onnx to encoder.xml and decoder.xml
Step 5. OpenVINO™ Inference Engine optimize S2T pipeline
OpenVINO™ Inference S2T pipeline We should adjust the contents in fairseq/sequence_generator.py +781 line "self.save_onnx = False" , +782 line "self.openvino_engine =True" Use the converted the model to run OpenVINO™ Inference S2T pipeline.
OpenVINO™ Inference Engine initialization

Encoder part inference by OpenVINO™


Decoder part inference by OpenVINO™

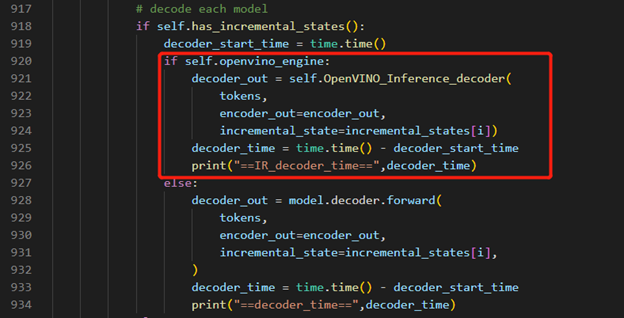
Inference Result

MiniCPM-V-2 model enabling with OpenVINO
Introduction
MiniCPM is an End-Side LLM developed by ModelBest Inc. and TsinghuaNLP. MiniCPM-V is a series of end-side multimodal LLMs (MLLMs) designed for vision-language understanding. The models take image and text as inputs and provide high-quality text outputs. MiniCPM-V 2.0 is an efficient version with promising performance for deployment. The model is built based on SigLip-400Mand MiniCPM-2.4B, connected by a perceiver resampler. On this blog, we provide the OpenVINO™ optimization for MiniCPM-V 2.0 on Intel® platforms.
You can find more information on GitHub repository:https://github.com/OpenBMB/MiniCPM-V
OpenVINO™backend on Minicpm-V-2
Step 1: Install system dependency and setup environment
Create and enable python virtual environment
Clone the MiniCPM-V repository from GitHub
Chage the current directory to the MiniCPM-V OpenVINO™ Runtime folder
Install python dependency
Step2: Export to OpenVINO™ models
Step3: Simple inference test with OpenVINO™
Question: Describe the content of the image
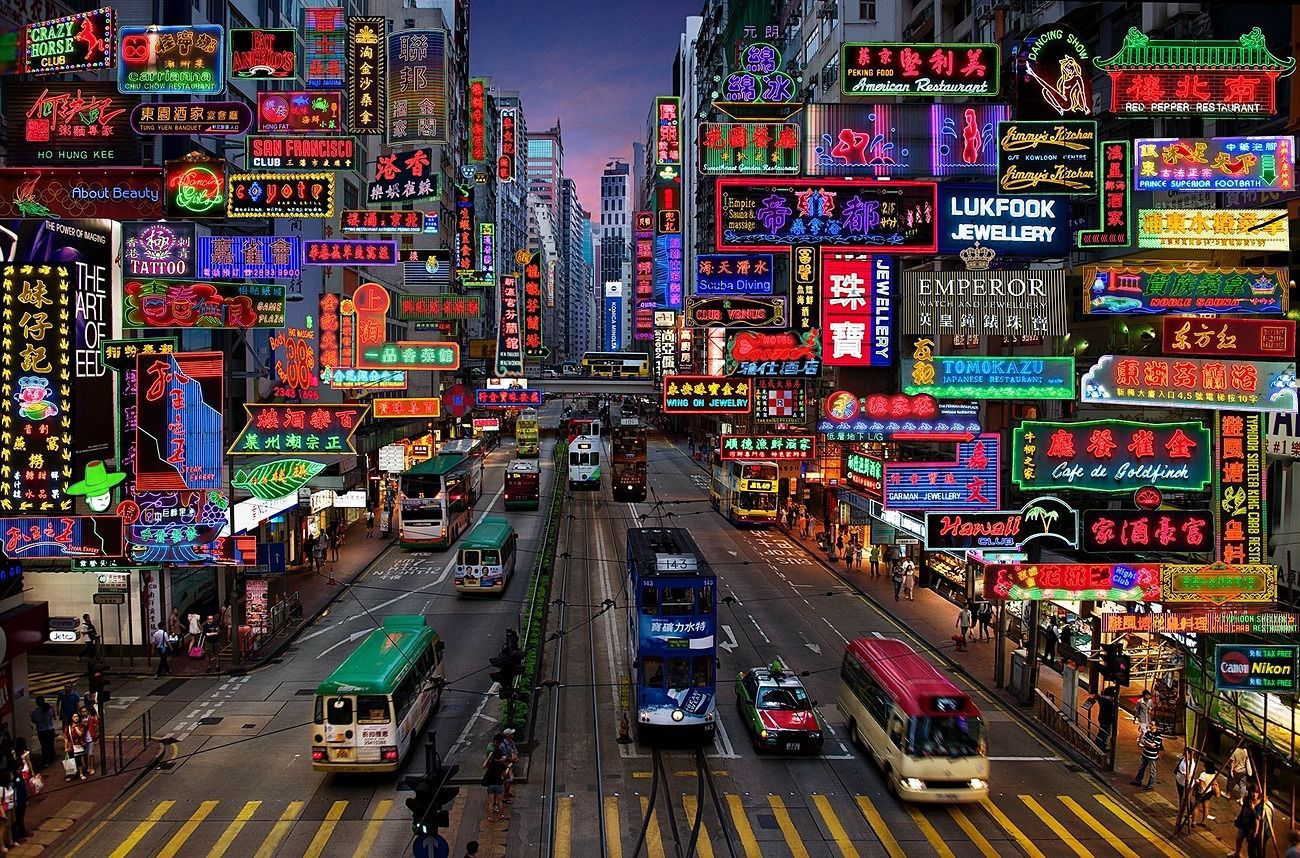
Answer:
The image captures the vibrant and bustling atmosphere of abusy city street in Hong Kong. The street is lined with an array of neon signsand billboards, each one advertising a different business or establishment. Thesigns are in a variety of languages, including English, Chinese, and Japanese,reflecting the multicultural nature of the city.
The street itself is a hive of activity with several busesand a tram making their way through the traffic. The vehicles are in motion,adding a dynamic element to the scene.
The sky above is a beautiful gradient of colors,transitioning from a deep blue at the top to a lighter shade at the bottom.This suggests that the photo was taken during either sunrise or sunset, castinga warm glow over the cityscape.
The image also contains several text elements, including thenames of various establishments and the brand names of products. These textsadd another layer of information to the scene, providing insights into thenature of the businesses and the products they offer.
Overall, the image provides a vivid snapshot of life in HongKong, capturing the city's vibrant energy and the diverse range of businessesand products that make up its bustling streets.
Enable OpenVINO™ Optimization for GroundingDINO
Authors: Wenyi Zou, Xiake Sun
Introduction
GroundingDINO introduces a language-guided query selection module to enhance object detection using input text. This module selects relevant features from image and text inputs and uses them as decoder queries. In this blog, we provide the OpenVINO™ optimization for GroundingDINO on Intel® platforms.
The public GroundingDINO project is referenced from: GroundingDINO
The GroundingDINO refer the model structure in below picture:
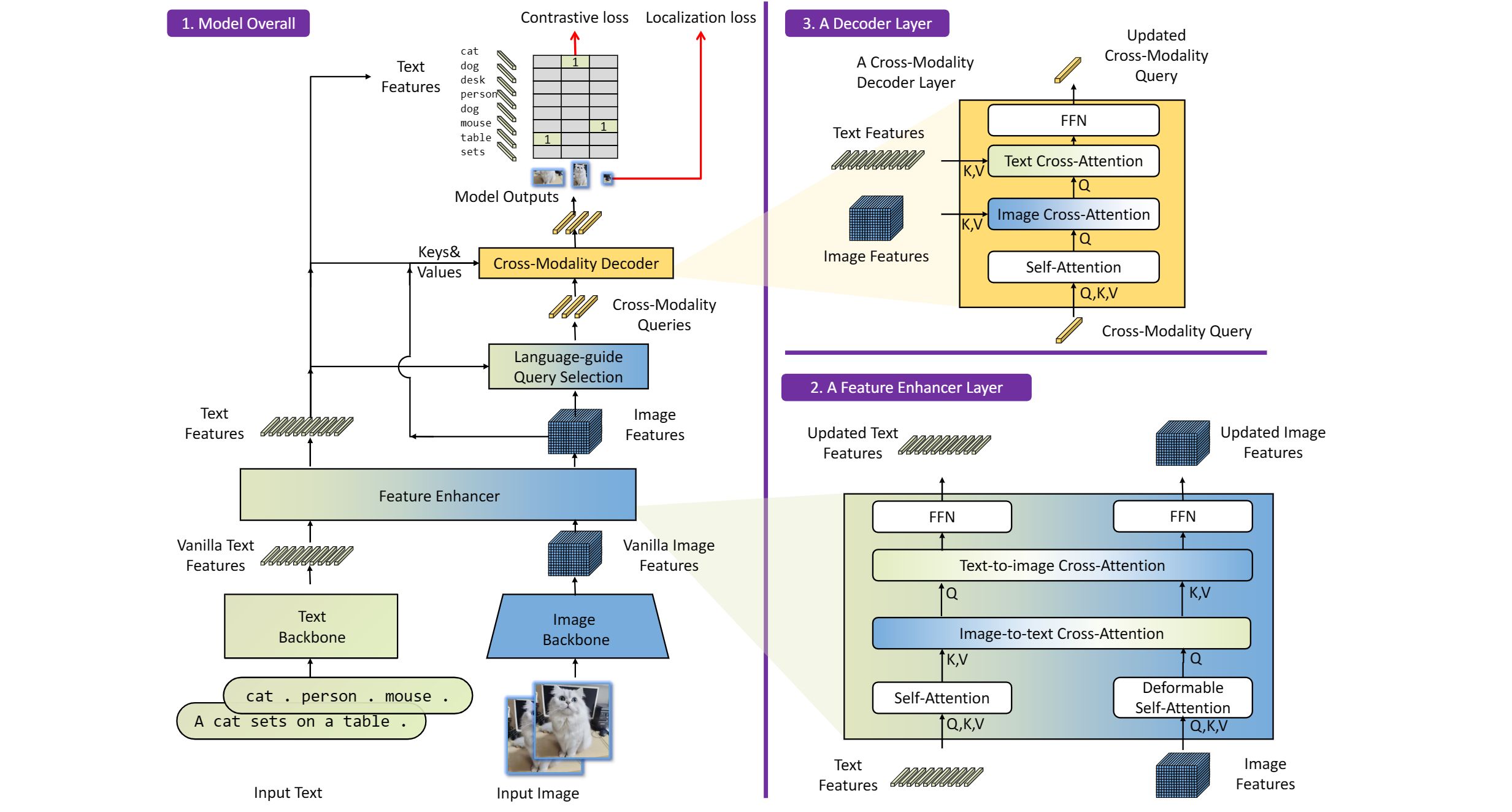
OpenVINO™ backend on GroundingDINO
In this project, you do not require to download OpenVINO™ and build the library with GroundingDINO project manually. It’s already fully integrated with OpenVINO™ runtime library for downloading, program compiling and linking.
At present, this repository already optimized and validated by OpenVINO™ 2023.1.0.dev20230811 version. Check the operating system which can support OpenVINO™ runtime library directly:
- Ubuntu 22.04 long-term support (LTS), 64-bit (Kernel 5.15+)
- Ubuntu 20.04 long-term support (LTS), 64-bit (Kernel 5.15+)
- Ubuntu 18.04 long-term support (LTS) with limitations, 64-bit (Kernel 5.4+)
- Windows* 10
- Windows* 11
- macOS* 10.15 and above, 64-bit
- Red Hat Enterprise Linux* 8, 64-bit
Step 1: Install system dependency and setup environment
Create and enable python virtual environment
Clone the GroundingDINO repository from GitHub
Change the current directory to the GroundingDINO folder
Install python dependency
Install the required dependencies in the current directory
Download pre-trained model weights
Step 2: Export to OpenVINO™ models
Step 3: Simple inference test with PyTorch and OpenVINO™
Inference with PyTorch
Inference with OpenVINO™
