OpenVINO Blog
Enable 2D Lip Sync Wav2Lip Pipeline with OpenVINO Runtime
Authors: Xiake Sun, Kunda Xu
1. Introduction
Lip sync technologies are widely used for digital human use cases, which enhance the user experience in dialog scenarios.
Wav2Lip is a novel approach to generate accurate 2D lip-synced videos in the wild with only one video and an audio clip. Wav2Lip leverages an accurate lip-sync “expert" model and consecutive face frames for accurate, natural lip motion generation.
In this blog, we introduce how to enable and optimize Wav2Lippipeline with OpenVINOTM.
Here is Wav2Lip pipeline overview:

2. Setup Environment
Download the Wav2lip pytorch model from link and move it to the checkpoints folder.
3. Pytorch to OpenVINOTM Model Conversion
The exported OpenVINOTM model will be saved in the checkpoints folder.
4. Run pipeline inference with OpenVINOTM Runtime
Here are the parameters with descriptions:
--face_detection_path: path of face detection OpenVINOTMIR
--wav2lip_path: path of wav2lip openvinoTM IR
--inference_device: specify the device to run OpenVINOTMinference.
--face: input video with face information
--audio: input audio with voice information
--static: set True to use single frame for face detection for fast inference
The generated video will be saved as results/result_voice.mp4
Here is an example to compare original video and generated video after the Wav2Lip pipeline:
.gif)

5. Conclusion
In this blog, we introduce how to deploy wav2lip pipeline with OpenVINOTM as follows:
- Support Pytorch model to OpenVINOTM model conversion.
- Run and optimize wav2lip pipeline with OpenVINOTM runtime.
OpenVINO Enable Digital Human-TTS (GPT-SoVITs)
Authors : Kunda, Xu / Aidova, Ekaterina
Introduction
GPT-SoVITS is a powerful voice cloning model that supports a small amount of voice conversion and text-to-speech. It supports voice reasoning in Chinese, English, and Japanese.
You only need to provide a 5-second voice sample to experience voice cloning with 80%~95% similarity. If you provide a 1-minute voice sample, you can get close to the effect of a real person and train a high-quality TTS model!
As the founder of RVC Voice Changer (GitHub nickname: RVC-Boss), he recently opened up a cross-language voice cloning project. GPT-SoVITs has attracted highly praised recommendations from the industry as soon as it went online, and it has received 1.4k Stars on GitHub in less than two days. Its voice cloning effect close to that of a real person and the use of Zero-short have made GPT-SoVITs highly recognized in the field of digital humans.
Although there are many tutorials on how to use GPT-SoVITs online, in this article I will show you how to optimize it through OpenVINO and deploy it on the CPU. GPT-SoVITs belongs to the category of TTS tasks, but the difference is that it can add sound features to the generated audio by introducing additional reference audio (ref_wav), making the output audio effect closer to real people speaking.
The pipeline structure of GPT-SoVITs
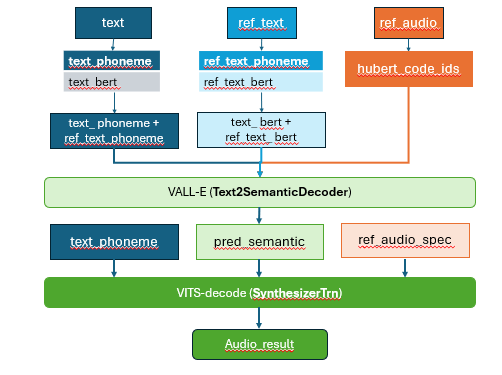
GPT-SOVITS adds a residual quantization layer to the original SOVITS input. Referring to VALL-E, the input of this quantization layer contains the text features and timbre features of the audio. GPT-SoVITs mainly consists of four parts: text preprocessing, audio preprocessing, VALL-E, VITS-decode
- Text preprocessing :
Convert text encoding information (text_ids) into pronunciation encoding information (phoneme_ids)
Use the Bert model to encode the phoneme_ids information, and take the third-to-last layer tensor as the result output
Phoneme features and Bert features are phoneme features for text, similar to pinyin
- Audio preprocessing :
Use the cn_hubert_encoder model to sample the audio information and extract the code_ids of ref_audio
Based on ref_audio, extract the frequency ref_audio_spec frame feature
- VALL-E:
Use for predict pred_sementic and idx, The idx is used to truncate pred_sementic (remove the features of the reference audio)
- VITs-decode :
Use VITS-decode to decode and get the output
HuBert sub model
The HuBert model is designed to extract audio autoencoder features and is developed by the Facebook AI research team. The goal is to learn a universal speech representation that can capture important information in speech signals, such as speech content, speaker identity, emotional state, etc. These representations can be used in a variety of downstream tasks, such as automatic speech recognition (ASR), speaker identification, sentiment analysis, etc.
Reference paper : https://arxiv.org/pdf/2106.07447

VALL-E sub model
VALL-E is a neural codec model developed by Microsoft Research, focusing on the field of speech synthesis. The characteristic of VALL-E is that it can generate synthesized speech that meets the speech characteristics of the target speaker through a small number of target speech samples (such as a 3-second speech clip). VALL-E completes the generation of speech coding based on Encoder. The GPT module of GPT-SOVITS implements the process from text to speech coding. Referring to SOVITS, a residual quantization layer is added to the original entrance. The input of this quantization layer contains the text features and timbre features of the audio.
Reference paper : https://arxiv.org/pdf/2301.02111

SoVITs sub model
The core module of GPT-SOVITS is not much different from SOVITS, which is an end-to-end text-to-speech(TTS) synthesis model. It combines variational inference and adversarial learning to generate high-quality, natural-sounding speech. It is still divided into two parts:
- Generator based on VAE + FLOW
- Discriminator based on multi-scale classifier
Reference paper : https://arxiv.org/pdf/2106.06103
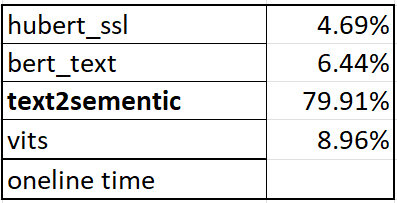
By counting the inference time of the entire GPT-SoVITs pipeline, we found that the two models with the largest time overhead are text2sementic (VALL-E) and vits (VITs-decode). The total overhead of the two models accounts for 87% of the entire pipeline. Therefore, optimizing these two models through OpenVINO is very necessary to improve the performance of the entire pipeline.
You canrefer to the sample code snippet to achieve this. OpenVINO enables HuBert, Bert, VALL-E, VITs 4 sub models, and builds the pipeline based on OpenVINO.
Reference GitHub project : 18582088138/GPT-SoVITS-OpenVINO:[OpenVINO Enable]1 min voice data can also be used to train a good TTS model! (few shot voice cloning) (github.com)
Export HuBert model code snippet
HuBert sub model definition

HuBert sub model convert

Export Bert model code snippet
Bert sub model definition and convert

Export VALL-E model code snippet
Since t2sis defined as three modules in the source code: t2s_encoder, first_stage_decoder, stage_decoder, refer to its source code implementation and convert these three modules into corresponding IR models through OpenVINO.
However, if the generated class model can refer to the Transformer basic model for KV cache optimization, the performance of the t2s model will be further improved, but this requires some code development work, and I will add this function in subsequent work (To Do).
VALL-E sub model definition
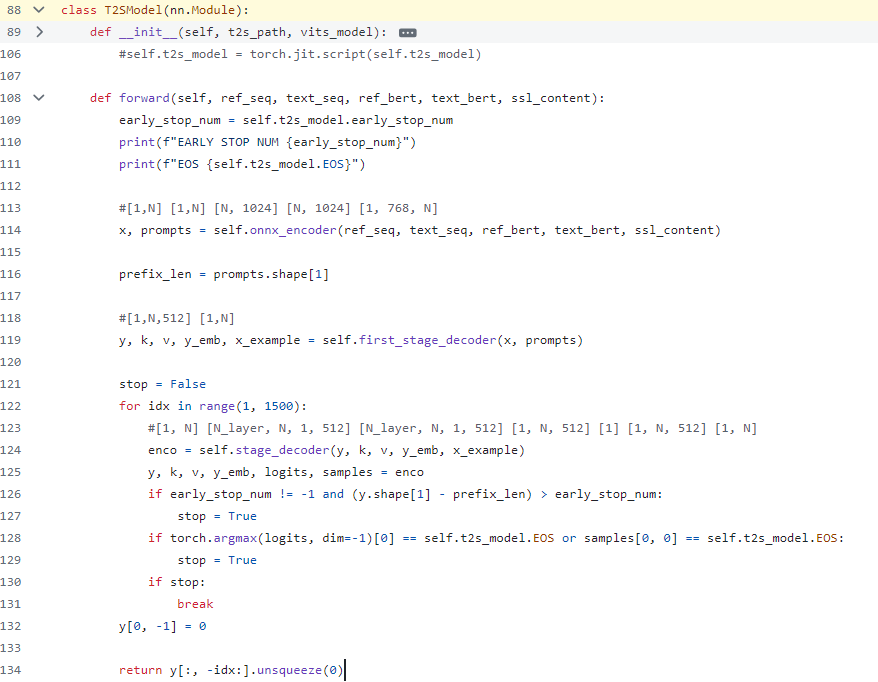
VALL-E sub model convert

Export VITs sub model code snippet
In the VITs model, the spectrogram torch function has two operators "torch.hann_window" and "torch.stft". Currently, OpenVINO opset does not support these two operators. Therefore, this part temporarily needs to use the operators in torch opset and redefine the original VITs sub-model. After the subsequent operators are successfully supported, OpenVINO will re-enable the VITs model (To Do).
VITs sub model definition
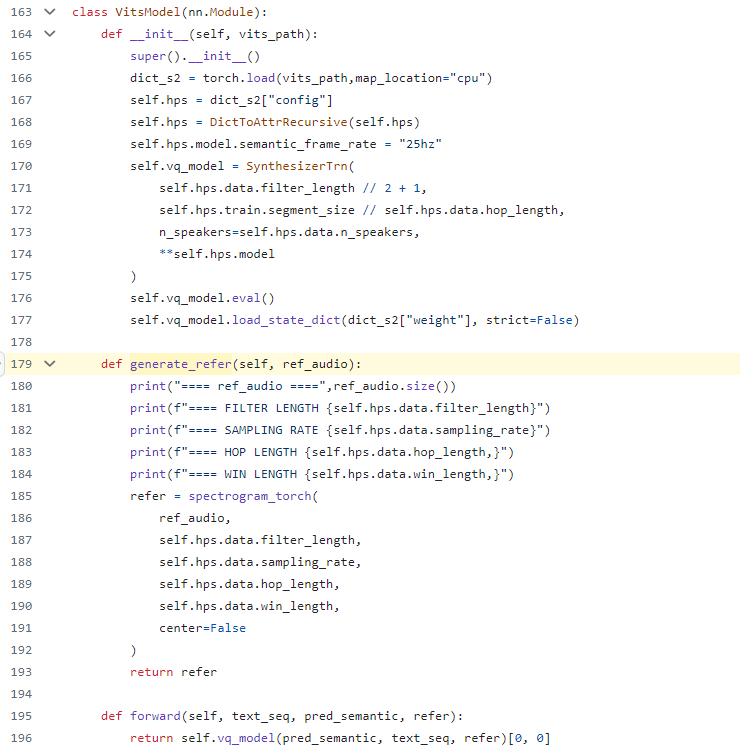
VITs sub model convert

Finally, we rebuilt the GPT-SoVITs pipeline through the four sub models enabled by OpenVINO. You can refer to the implementation of the pipeline:
Reference link : GPT-SoVITS-OpenVINO/GPT_SoVITS/ov_pipeline_gpt_sovit.py at 18582088138/GPT-SoVITS-OpenVINO(github.com)
Enable Personalized Text-to-Speech Pipeline with SAMBERT-HifiGAN via OpenVINO Python API
Authors: Tianmeng Chen, Xiake Sun, Fiona Zhao, Su Yang
Introduction
Personalized Speech Synthesis is the process of using some recording devices around you to record certain voice clips of a particular person, and then letting Text-To-Speech (TTS) technology synthesize the voice, manner of speaking, and emotion of a particular person. SAMBERT-HifiGAN is a complete personalized TTS solution designed by Alibaba Damo Institute, which includes the first part of SAMBERT's acoustic model and the second part of the HifiGAN vocoder.

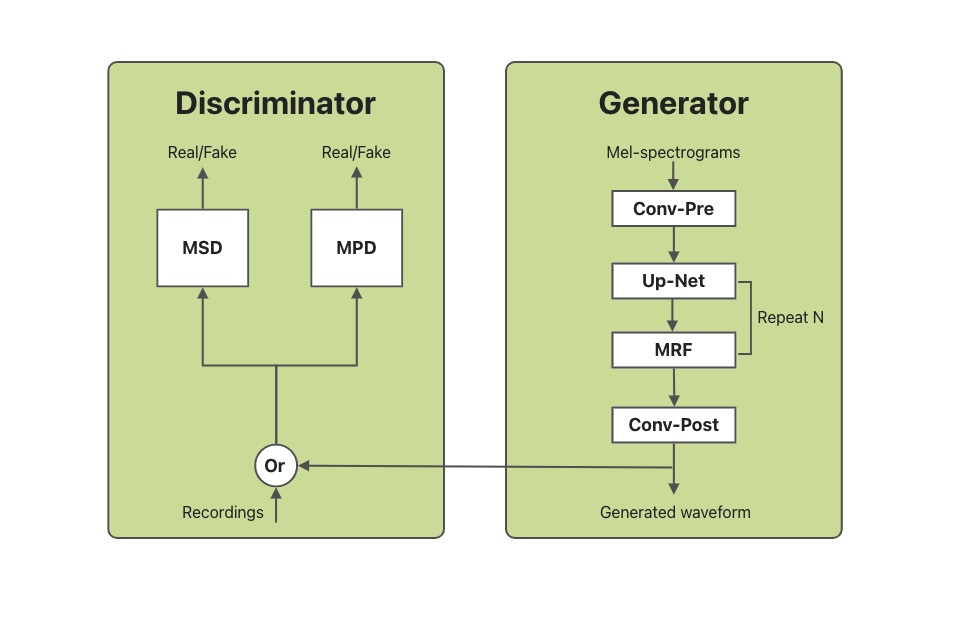
In this blog, we will introduce how to utilize OpenVINOTM Python API to enable the SAMBERT-HifiGAN pipeline. All the project code can be found here.
KAN-TTS by Ali provides a tutorial for training SAMBERT-HifiGAN. A pipeline for personalized speech synthesis based on PyTorch is provided on modelscope, what we will do here is toreplace the PyTorch based part of it with OpenVINOTM. It is worth noting that due to some of the operators in the model, there are some modules that cannot be replaced with OpenVINOTM Python API.
Pre-requisite
Since we need to make changes on the pipeline based on PyTorch backend, the first thing we need to do is to download the KAN-TTS source code and successfully run through the pipeline to get the inputs and outputs of the model as well as the state of the middle layer. Of course we also need the OpenVINOTM environment.
- Get the KAN-TTS source code and create anacondaenvironment.
- Then we install openvino in same environment. Ifyou want specific version of OpenVINOTM, you can install it byyourself through Install OpenVINO™.
- Follow the KAN-TTS practice tutorial of official with readme in ModelScope.
After you finish the pipelining of KAN-TTS, you can get the res folder and ckpt filesspeech_personal_sambert-hifigan_nsf_tts_zh-cn_pretrain_16k .
- Get the OpenVINOTM backend projectsource code and copy the res folder to project folder.
Convert torch modelto openVINOTM model
Converting a torch model to OpenVINOTM requires model inputs. So we usetest.txt as input of SAMBERT and use the res folder as input of HifiGAN.
Aftera few minutes, you will get two converted OpenVINOTM model sambert_encoder.xml sambert_encoder.bin and hifigan_t.xml hifigan_t.bin.
In the code after we load the model and get the inputs, we add the following code to convert the loaded PyTorch backend model to OpenVINOTM backend model and save it.
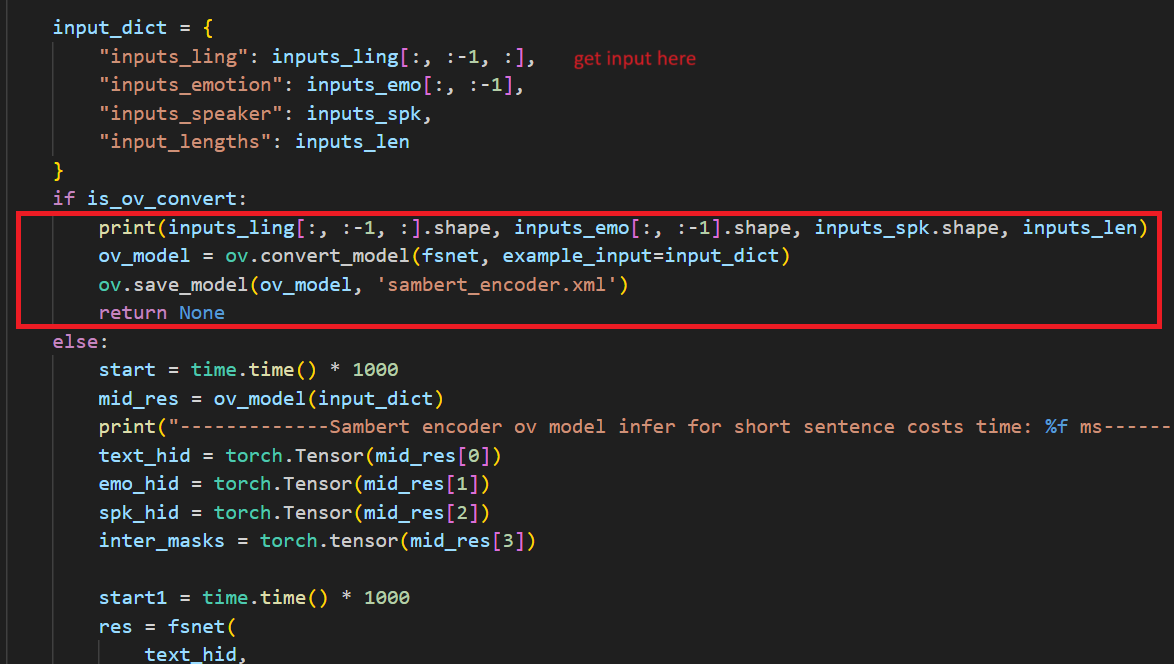
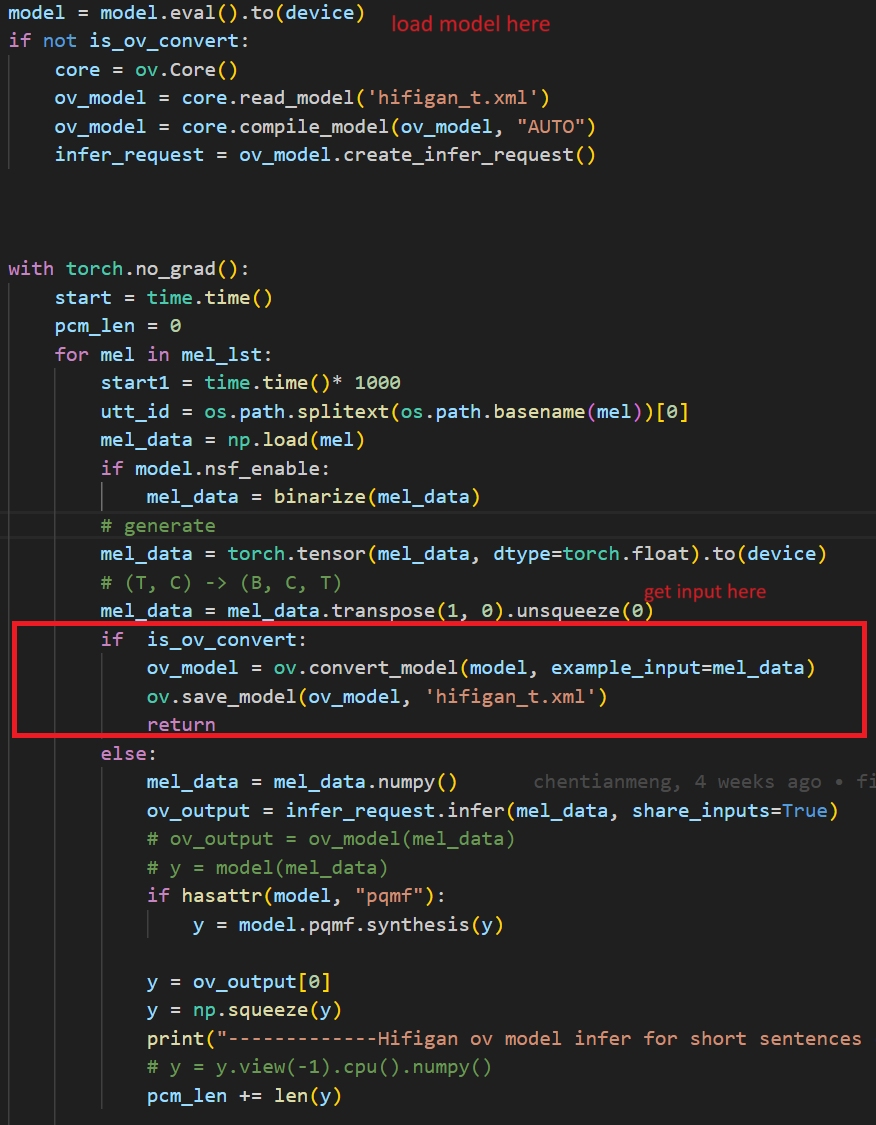
Run the inferencewith OpenVINOTM model
Before running the inference, the res folder should be renamed to allow for comparisons later.
then run the command below.
After a few minutes, you will get the wav file in res/test_male_ptts_syn. For example in test.txt we write a random sentence:

After running pipeline, we will get a 7 seconds wav file under res folder:
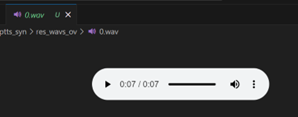
In the code we modified the original pytorch banckend inference code so that pipeline uses openvino backend for inference.


Summary
This blog describes about how to run the SAMBERT-HifiGANpipeline using the OpenVINOTM Python API, please see the source code formore details and modifications.
SDPA Enabling for Custom Model
Authors: Su Yang, Xiake Sun, Fiona Zhao
Introduction
To enable the SDPA fusion on GPU, we firstly need to convert model IR with SDPA Op.
Create new class SdpaAttention in the modeling_ custom_model.py using torch.scaled_dot_product_attention. This Pytorch Op could be matched and converted into OpenVINO SDPA Op.
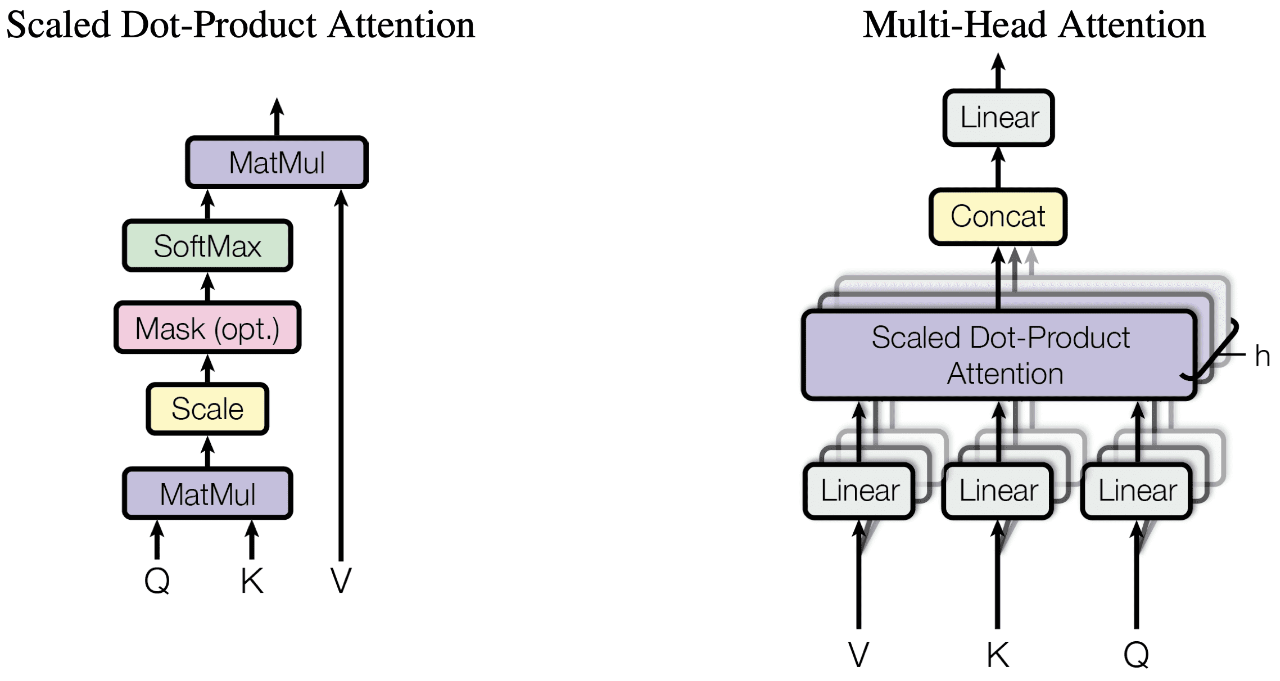
Refer to Phi3SdpaAttention, this module is inherited from Phi3Attention as the weights of the module stay untouched. The only changes are on the forward pass to adapt to SDPA API.
torch.scaled_dot_product_attention
From the equivalent implementation, the target is to replace the related Pytorch Ops(like softmax, matmul and dropout) with torch.nn.functional.scaled_dot_product_attention.

For some custom model, the equivalent code in is as follow:
The corresponding implementation of scaled_dot_product_attention:
SDPA’s Scaling factor with different head_size
For the Pytorch model with different head_size, this scaled_dot_product_attention need to be modified with the scaling factor.
The SDPA Operator has already implemented. The SDPA fusion on GPU supports for head size =128 at OV24.2 release.
The OV24.3 release relax SDPA head size limitations for LLMs from 128 only to a range of 64 to 256, thanks to Sergey’s PR.
Usage
Replace the original modeling_custom_model.py with the new script (with SdpaAttention) in the Pytorch model folder.
Notice:
- After converting model again, check the SDPA layers (“aten::scaled_dot_product_attention”) in the OV IR .xml file.
- Double check the OpenVINO executable graph for the SDPA enabling.
- Don’t forget to check the accuracy of the Pytorch model inference, after any modification with modeling_custom_model.py.
Conclusion
In this blog, we introduce how to use torch.scaled_dot_product_attention to enable the SDPA for custom model.
Performance improvement with SDPA on MTL iGPU is depended on the model structure. SDPA enabling for the custom model is the base for further optimization like Page Attention.
OpenVINO Optimization-LLM Distributed
Author : Kunda,Xu / Zhai, Xiuchuan / Sun, Xiaoxia / Li, Tingqian / Shen, Wanglei
Introduction
With the continuous development of deep learning technology, large models have become key technologies in many fields, such as LLM, Multimodal, etc. The training of large models requires a lot of computing resources, and the reasoning and deployment of LLM also require a lot of resources, which promotes the widespread application of distributed parallel technology. Distributed parallel technology can distribute a large model to multiple computing nodes and accelerate the training and reasoning process of the model through parallel computing. It mainly includes strategies such as Model Parallel(MP), Data Parallel(DP), Pipeline Parallel(PP), and Tensor Parallel(TP).
In this blog we will focus on OpenVINO's distributed optimization of LLM by tensor parallel.
Tensor parallel is a technique used to fit a large model in multiple device. For example, when multiplying the input tensors with the first weight tensor, the matrix multiplication is equivalent to splitting the weight tensor column-wise, multiplying each column with the input separately, and then concatenating the separate outputs. These outputs are then transferred from the CPUs/GPUs and concatenated together to get the final result.

Taking the implementation of Fully Connect(FC) layer tensor parallel as an example, we introduce OpenVINO's distributed optimization of LLM. In the statistics of the inference time overhead of LLM tasks, we found that due to the large parameter size of LLM, the FC layer will take up a large time overhead. By optimizing the latency of the FC layer, the LLM first token/second token latency can be effectively reduced.
Fully connect layer refers to a neural network in which each neuron applies a linear transformation to the input vector through a weights matrix. As a result, all possible connections layer-to-layer are present, meaning every input of the input vector influences every output of the output vector.
In OpenVINO, SplitFC is used to split the FC layer into two device nodes to implement tensor parallelism.
SplitFC detects FC CPU operation with and without compressed weights. And then splits the FC into several small FCs by output channel according to sub stream number. The goal is that the executor can dispatch the split FCs to different numa nodes in the system. As a result, the split FCs can be executed at the parallel level.

The following describes how to use the distributed features in OpenVINO to deploy the model on two CPU nodes to improve the performance of LLM.
Step 1. setup environment
Step 2. clone openvino.genai source code and install requirement
Step 3. prepare OpenVINO IR LLM model
The conversion script for preparing benchmarking models, convert.py allows to reproduce IRs stored on shared drive. Make sure the prerequisty requirements.txt has already install.
Step 4. LLM run pipe OpenVINO genai benchmark on single socket

Step 5. LLM run pipe OpenVINO genai benchmark distributed on double sockets

As can be seen from the figure above, nstream=1, nireq=1,LLM runs on two sockets, and from the printout of OV_config, we can see that MODEL_DISTRIBUED_POLICY has turned on the TENSOR_PARALLEL feature;
Using OpenVINO distributed feature can significantly reduce the first token/second token latency for general LLM models.
MiniCPM-V-2 model enabling with OpenVINO
Introduction
MiniCPM is an End-Side LLM developed by ModelBest Inc. and TsinghuaNLP. MiniCPM-V is a series of end-side multimodal LLMs (MLLMs) designed for vision-language understanding. The models take image and text as inputs and provide high-quality text outputs. MiniCPM-V 2.0 is an efficient version with promising performance for deployment. The model is built based on SigLip-400Mand MiniCPM-2.4B, connected by a perceiver resampler. On this blog, we provide the OpenVINO™ optimization for MiniCPM-V 2.0 on Intel® platforms.
You can find more information on GitHub repository:https://github.com/OpenBMB/MiniCPM-V
OpenVINO™backend on Minicpm-V-2
Step 1: Install system dependency and setup environment
Create and enable python virtual environment
Clone the MiniCPM-V repository from GitHub
Chage the current directory to the MiniCPM-V OpenVINO™ Runtime folder
Install python dependency
Step2: Export to OpenVINO™ models
Step3: Simple inference test with OpenVINO™
Question: Describe the content of the image
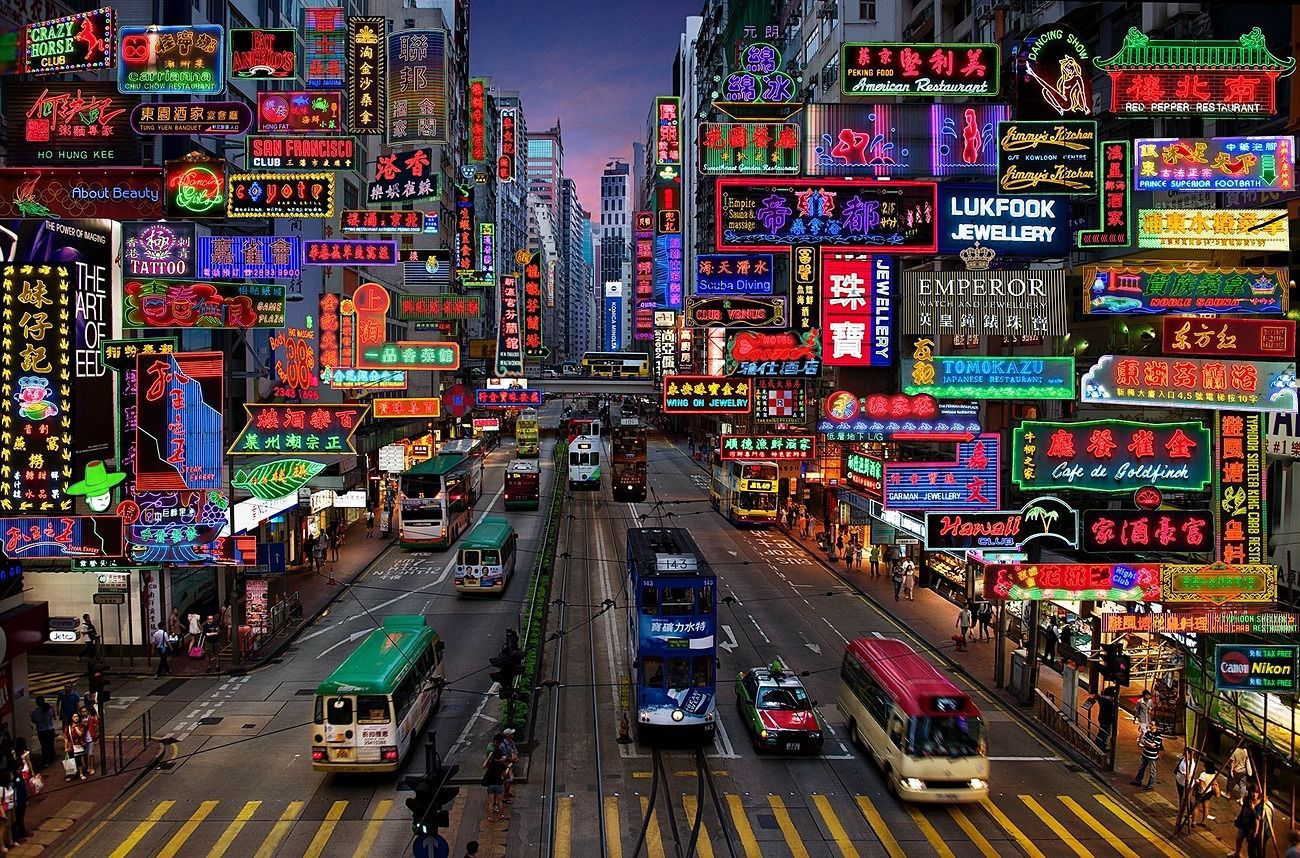
Answer:
The image captures the vibrant and bustling atmosphere of abusy city street in Hong Kong. The street is lined with an array of neon signsand billboards, each one advertising a different business or establishment. Thesigns are in a variety of languages, including English, Chinese, and Japanese,reflecting the multicultural nature of the city.
The street itself is a hive of activity with several busesand a tram making their way through the traffic. The vehicles are in motion,adding a dynamic element to the scene.
The sky above is a beautiful gradient of colors,transitioning from a deep blue at the top to a lighter shade at the bottom.This suggests that the photo was taken during either sunrise or sunset, castinga warm glow over the cityscape.
The image also contains several text elements, including thenames of various establishments and the brand names of products. These textsadd another layer of information to the scene, providing insights into thenature of the businesses and the products they offer.
Overall, the image provides a vivid snapshot of life in HongKong, capturing the city's vibrant energy and the diverse range of businessesand products that make up its bustling streets.
Q2'24: Technology Update – Low Precision and Model Optimization
Authors
Alexander Kozlov, Nikita Savelyev, Vui Seng Chua, Souvikk Kundu, Nikolay Lyalyushkin, Andrey Anufriev, Pablo Munoz, Alexander Suslov, Liubov Talamanova, Yury Gorbachev, Nilesh Jain, Maxim Proshin
Summary
This quarter we see an increasing interest in KV-cache optimization of Large Language and Vision Models. This actually expected as KV-cache is getting a bottleneck after the weight compression problem is solved to some degree. We also believe that KV-cache optimization will continue being a hot topic as it is also involved in the Video Generations scenario where we see a lot of work going on nowadays.
Highlights
- QServe: W4A8KV4 Quantization and System Co-design for Efficient LLM Serving by MIT, NVIDIA, UMass Amherst, MIT-IBM Watson AI Lab (https://arxiv.org/pdf/2405.04532). A regular work from Song Han Lab which is a comprehensive study of deep LLM optimization and a reference design of a tool for LLM serving. The LLM optimization part includes: W4A8 and 4-bit KV-cache quantization approach; Progressive quantization of weights, to comply with 8-bit compute after dequantizing4-bit weights to 8-bits; SmoothAttention method, to reduce the error of 4-bit quantization of Key cache that is compatible with RoPE operation and can be fused into a preceding Linear layer; Progressive quantization of weights, to comply with 8-bit compute after dequantizing4-bit weights to 8-bits. The inference part contains tips and tricks to design efficient inference kernels and execution pipelines on the Nvidia GPUs. The method shows superior results comparing to competitive solutions and demonstrates the ability to substantially reduce LLM serving costs. Some code and pre-compiled binaries are available here: https://github.com/mit-han-lab/qserve.
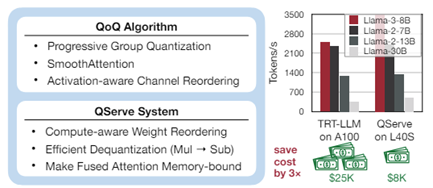
- ZipCache: Accurate and Efficient KV Cache Quantization with Salient Token Identification by Houmo AI and Chinese universities (https://arxiv.org/pdf/2405.14256).Authors present a KV cache quantization method for LLMs. First, they construct a strong baseline for quantizing KV cache. Through the proposed channel-separable token-wise quantization scheme, the memory overhead of quantization parameters is substantially reduced compared to fine-grained group-wise quantization. To enhance the compression ratio, they propose a normalized attention score. The quantization bit-width for each token is adaptively assigned based on their saliency. The authors also develop an approximation method that decouples the saliency metric from full attention scores compatible with FlashAttention. Experiments demonstrate that the method achieves good compression ratios at fast generation speed, for example, when evaluating Mistral-7B model on GSM8k dataset, the method is capable of compressing the KV cache by 4.98×,with only a 0.38% drop in accuracy.
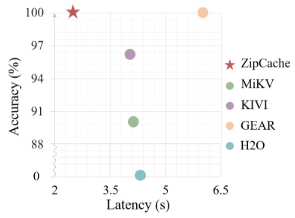
- BitsFusion: 1.99 bits Weight Quantization of Diffusion Model by Snap Inc. and Rutgers University (https://arxiv.org/pdf/2406.04333). The paper provides a thorough analysis of UNet weight-only quantization of Stable Diffusion 1.5 model. The authors propose an approach for mixed-precision quantization of diffusers. They quantize different layers into different bits according to their quantization error. The authors also introduce several techniques to initialize the quantized model to improve performance, including time embedding pre-computing and caching, adding balance integer, and alternating optimization for scaling factor initialization. Finally, they propose a two-stage Quantization-aware training where distillation is used at the first stage. The quantized model achieves very good results on various benchmarks. Code will be released here: https://github.com/snap-research/BitsFusion.

- Applying t-Distributions to Explore Accurate and Efficient Format[KA1] s for LLMs by Cornell University and Google (https://arxiv.org/abs/2405.03103). The paper investigates non-uniform quantization data formats by profiling the distributions of weight and activation across 30 models, including both LLM and non-LLM models. The authors discovered that Student’s t-Distribution is a better fit than the Gaussian distribution due to its flexible parameterization, which can resemble Gaussian, Cauchy, or other distributions observed indifferent neural networks. The authors derived Student Float (SF4) using a similar design process to Normal Float (NF4). SF4 outperforms NF4, FP4, and Int4 in accuracy retention across most cases and model architectures, making it a strong drop-in replacement for lookup-based datatypes like NF4. The paper proposes using SF4as a reference to extend supernormal support for existing datatypes like E2M1(one variant of FP4) and APoT4,by reassigning negative zero to a useful value, which is otherwise wasted. Additionally, the paper examines the Pareto frontier of datatypes in terms of model accuracy and MAC chip area, concluding that APoT4 and its supernormal extension are Pareto optimal for a set of models smaller than 7B parameters.
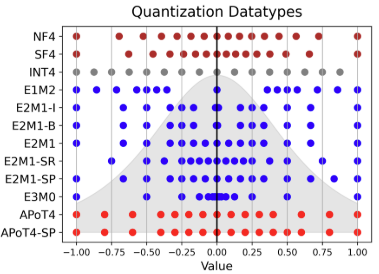
- ShiftAddLLM: MatMul-free LLM via Inference Time Reparameterization by Intel, Google Deep Mind, Google, Georgia Tech (https://arxiv.org/pdf/2406.05981).Authors developed an inference time reparameterization for traditional LLMs layers with MatMul ops to convert them to layers with Shift-Add and LUT query-based operations only. Specifically, authors quantize each weight matrix into binary matrices paired with group-wise scaling factors. The associated multiplications are reparameterized into (1) shifts between activations and scaling factors and (2) queries and adds according to the binary matrices. To reduce accuracy loss, they present a multi-objective optimization method to minimize both weight and output activation reparameterization errors. Additionally, based on varying sensitivity across layers to reparameterization, they develop an automated bit allocation strategy to further reduce memory usage and latency. The code is available at: https://github.com/GATECH-EIC/ShiftAddLLM.
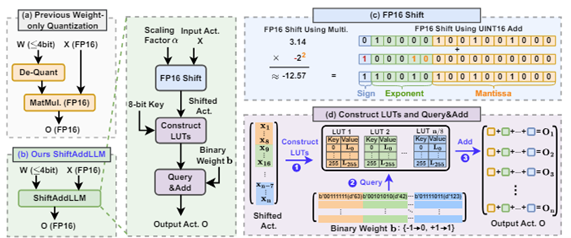
Quantization
- QServe: W4A8KV4 Quantization and System Co-design for Efficient LLM Serving by MIT, NVIDIA, UMass Amherst, MIT-IBM Watson AI Lab (https://arxiv.org/pdf/2405.04532). A regular work from Song Han Lab which is a comprehensive study of deep LLM optimization and a reference design of a tool for LLM serving. The LLM optimization part includes: W4A8 and 4-bit KV-cache quantization approach; Progressive quantization of weights, to comply with 8-bit compute after dequantizing4-bit weights to 8-bits; SmoothAttention method, to reduce the error of 4-bit quantization of Key cache that is compatible with RoPE operation and can be fused into a preceding Linear layer; Progressive quantization of weights, to comply with 8-bit compute after dequantizing4-bit weights to 8-bits. The inference part contains tips and tricks to design efficient inference kernels and execution pipelines on the Nvidia GPUs. The method shows superior results comparing to competitive solutions and demonstrates the ability to substantially reduce LLM serving costs. Some code and pre-compiled binaries are available here: https://github.com/mit-han-lab/qserve.
- LQER: Low-Rank Quantization Error Reconstruction for LLMs by Imperial College London London and University of Cambridge (https://arxiv.org/pdf/2402.02446). The paper combines quantization and low-rank approximation techniques to achieve accurate and efficient LLM optimization. The method employs MXINT4 datatype (int4 + shared exponent for 4 elements) for weight quantization while quantizing activation into 8 or 6 bits with per-token scaling factors. The method also introduces 8-bit LoRA adapters to restore accuracy after weight quantization. It does not use any kind of fine-tuning. Instead, it introduces the error decomposition into two low-rank matrices. The method achieves very accurate results in W4A8 and W4A6 settings, especially on Llama-2 model family.
- LLM-QBench: A Benchmark Towards the Best Practice for Post-training Quantization of Large Language Models by Beihang University, SenseTime Research, and Nanyang Technological University (https://arxiv.org/pdf/2405.06001).The paper focuses on identifying the most effective practices for quantizing LLMs, with the goal of balancing performance with computational efficiency. Fora fair analysis, the authors develop a quantization toolkit LLMC and design four crucial principles considering the inference efficiency, quantized accuracy, calibration cost, and modularization. By benchmarking on various models and datasets with over 500 experiments, three takeaways corresponding to calibration data, quantization algorithm, and quantization schemes are derived. Finally, a best practice of LLM PTQ pipeline is constructed. All the benchmark results and the toolkit can be found at https://github.com/ModelTC/llmc.
- SKVQ: Sliding-window Key and Value Cache Quantization for Large Language Models Houmo AI by Houmo AI and Chinese universities (https://arxiv.org/pdf/2405.06219). The paper addresses the problem of extremely low bit-width KV cache quantization. To achieve this, it proposes a method that rearranges the channels of the KV cache in order to improve the similarity of channels in quantization groups and applies clipped dynamic quantization at the group level. Additionally, the method ensures that the most recent window tokens in the KV cache are preserved with high precision. This helps maintain the accuracy of a small but important portion of the KV cache. Evaluation on LLMs demonstrates that the method surpasses previous quantization approaches, allowing for quantization of the KV cache to 2-bit keys and 1.5-bit values with minimal loss of accuracy. The code is available at https://github.com/cat538/SKVQ.
- Integer Scale: A Free Lunch for Faster Fine-grained Quantization of LLMs by Meituan (https://arxiv.org/pdf/2405.14597). The paper proposes a scheme to use integer scales when computing dot products of W4A8quantized LLMs. It allows keeping group scales for weights in the integer precision as well and using INT32 buffer as the accumulator of partial dot products. An additional floating point scale is required and applied to the super-group of dot products between weights and activations. It brings the proposed method close to the known double quantization approach. The paper provides extensive evaluation data for Llama2 and Llama3 models showing close results to the baseline floating-point scales.
- Mitigating Quantization Errors Due to Activation Spikes in GLU-Based LLMs by Hanyang University (https://arxiv.org/pdf/2405.14428).The paper aims at reducing the accuracy degradation of fully-quantized LLM models (both weights and activations are quantized). Authors propose two empirical methods, Quantization-free Module (QFeM) and Quantization-free Prefix (QFeP), to isolate the activation spikes during quantization that cause most of the accuracy drop. Essentially, they propose a way to identify what layers are more error-prone and keep these layers in the floating-point precision. The code is available at https://github.com/onnoo/activation-spikes.
- AdpQ: A Zero-shot Calibration Free Adaptive Post Training Quantization Method for LLMs by Huawei Noah Lab and McGill University (https://arxiv.org/pdf/2405.13358).This paper presents a novel zero-shot adaptive PTQ method for LLMs that does not require any calibration data. Inspired by Adaptive LASSO regression model, the authors proposed approach that tackles the challenge of outlier activations by separating salient weights using an adaptive soft-thresholding method. Guided by Adaptive LASSO, this method ensures that the quantized weights distribution closely follows the originally trained weights and eliminates the need for calibration data entirely. The method achieves good results at much faster quantization time.
- PTQ4SAM: Post-Training Quantization for Segment Anything by Beihang University (https://arxiv.org/pdf/2405.03144).A practical study on quantization of the Segment Anything model. The authors observe a challenging bimodal distribution for quantization and analyze its characteristics. To overcome it, they propose a Bimodal Integration (BIG)strategy, which automatically detects it and transforms the bimodal distribution to normal distribution equivalently. They also present the Adaptive Granularity Quantization which represents diverse post-Softmax distributions accurately with appropriate granularity. Experiments show that the method can achieve good results even in low-bit quantization settings (6 or4 bits). Code is available at https://github.com/chengtao-lv/PTQ4SAM.
- QNCD: Quantization Noise Correction for Diffusion Models by Kuaishou Technology (https://arxiv.org/pdf/2403.20137). Authors identify two primary quantization challenges for Duffusion models: intra and inter quantization noise. Intra quantization noise, exacerbated by embeddings in the resblock module, extends activation quantization ranges, increasing disturbances in each single denoising step. Besides, inter quantization noise stems from cumulative quantization deviations across the entire denoising process, altering data distributions step-by-step. Authors propose embedding-derived feature smoothing for eliminating intra quantization noise and a runtime noise estimation module for dynamically filtering inter quantization noise. Experiments demonstrate that the method achieves good results in W4A8 and W8A8 quantization settings on ImageNet (LDM-4). Code is available at: https://github.com/huanpengchu/QNCD.
- SliM-LLM: Salience-DrivenMixed-Precision Quantization for Large Language Models by The ETH Zürich, University of Hong Kong, and Beihang University (https://arxiv.org/pdf/2405.14917).The paper focuses on the problem of ultra-low bit weight quantization of LLMs. Specifically, it proposes the method relies on two novel techniques: (1)Salience-Determined Bit Allocation utilizes the clustering characteristics of salience distribution to allocate the bit-widths of each quantization group. This increases the accuracy of quantized LLMs and maintains the inference efficiency high; (2) Salience-Weighted Quantizer Calibration optimizes the parameters of the quantizer by considering the element-wise salience within the group. The method is evaluated in two setups for quantization parameters tuning: greedy search and gradient based search. Evaluation shows good results on Llama 1/2/3 models. Code is available at https://github.com/Aaronhuang-778/SliM-LLM.
- LCQ: Low-Rank Codebook based Quantization for Large Language Models by Nanjing University (https://arxiv.org/pdf/2405.20973). The paper proposes a method for LLM optimization using customized low-ranking codebooks the rank of which can be larger than one, for quantization. A gradient-based optimization algorithm is proposed to optimize the parameters of the codebook. The method also adopts a double quantization strategy for compressing the parameters of the codebook, which can reduce the storage cost of the codebook. Experiments show that achieves better accuracy than existing methods with a negligibly extra storage cost.
- P2 -ViT: Power-of-Two Post-Training Quantization and Acceleration for Fully Quantized Vision Transformer by Nanjing University and Sun Yat-sen University (https://arxiv.org/pdf/2405.19915). The paper introduces a Power-of-Two (PoT) post-training quantization and acceleration framework for ViT models. The authors analyze ViTs’ properties and develop a dedicated quantization scheme. This scheme incorporates techniques such as adaptive PoT rounding and PoT Aware smoothing, allowing for the efficient quantization of ViTs with PoT scaling factors. By doing this, computationally expensive floating-point multiplications and divisions with in the re-quantization process can be traded with hardware-efficient bitwise shift operations. Furthermore, we introduce a coarse-to-fine automatic mixed-precision quantization methodology for better accuracy-efficiency trade-offs. Finally, authors build a dedicated accelerator engine to better everage our algorithmic properties for enhancing hardware efficiency. Code is available at: https://github.com/shihuihong214/P2-ViT.
- QJL: 1-Bit Quantized JLTransform for KV Cache Quantization with Zero Overhead by New York University and Adobe Research (https://arxiv.org/pdf/2406.03482).The paper studies problems of KV-cache quantization of LLMs, specifically the Key part as it is more error-prone when lowering its precision. Authors propose an approach that consists of a Johnson-Lindenstrauss (JL) transform followed by sign-bit quantization for Key cache. They introduce an asymmetric estimator for the inner product of two vectors and demonstrate that applying the method to one vector and a standard JL transform without quantization to the other provides an unbiased estimator with minimal distortion. They also developed a CUDA-based implementation for optimized computation. When applied across various LLMs and NLP tasks to quantize the KV cache to only 3 bits, the method demonstrates a more than fivefold reduction in KV cache memory usage without an insignificant accuracy drop. Codes will be available at https://github.com/amirzandieh/QJL.
- ViDiT-Q: Efficient and Accurate Quantization of Diffusion Transformers for Image and Video Generation by Tsinghua University, Infinigence AI, 3Microsoft, and Shanghai Jiao Tong University (https://arxiv.org/pdf/2406.02540).The paper tackles the problems of accurate quantization of diffusion vision transformer models. Essentially, authors apply dynamic 8-bit per-token quantization to activations. They also propose to smooth activation with a Smoothquant-like approach but with different α factors tuned to each iteration of the diffusion process. Finally, authors propose to select a per-layer weight bit-width (e.g.W4A8, W6A6, or W8A8) depending on the sensitivity and position of the layer in the Transformer block. All these tricks lead to very good accuracy results in the image and video generation tasks.
- Instance-Aware Group Quantization for Vision Transformers by Yonsei University and Articron (https://arxiv.org/pdf/2404.00928). In this paper an approach for instance-aware group quantization for ViTs(IGQ-ViT) is introduced. According to the approach, channels of activation maps are dynamically split into multiple groups where each group has its own set of quantization parameters. Authors also extend their scheme to quantize softmax attentions across tokens. IGQ-ViT demonstrates superior accuracy results across image classification, object detection and instance segmentation task. Authors claim that performance overhead induced by dynamic quantization is no more than 4% compared to layer-wise quantization.
- Reg-PTQ: Regression-specialized Post-training Quantization for Fully Quantized Object Detector by Beihang University (https://openaccess.thecvf.com/content/CVPR2024/papers/Ding_Reg-PTQ_Regression-specialized_Post-training_Quantization_for_Fully_Quantized_Object_Detector_CVPR_2024_paper.pdf). In this paper authors explore full quantization of object detection models contrary to most existing approaches which quantize only detection backbones and keep detection head in original precision. Based on the findings, the reason behind poor quantization of detector heads is that they are optimized to solve regression tasks. Specifically, authors argue that (1) regressors are more sensitive to perturbation compared to classifiers, (2) minimizing quantization error does not necessarily result in optimal scaling factors for regressor and(3) regressors weights follow non-uniform distribution contrary to classifiers. To tackle these problems a novel Reg-PTQ method is introduced. Based on the results it achieves 7.6x and 5.4x reduction in computation and storage consumption under INT4 precision with little performance degradation.
- Towards Accurate Post-training Quantization for Diffusion Models (https://openaccess.thecvf.com/content/CVPR2024/papers/Wang_Towards_Accurate_Post-training_Quantization_for_Diffusion_Models_CVPR_2024_paper.pdf). In this paper authors propose a method for accurate post-training quantization of diffusion models. The main idea is to split diffusion timesteps for each layer into groups where each group corresponds to its own set of quantization parameters. Such split is obtained by minimizing some optimization objective on a calibration dataset. Besides this, a special timestep selection method is employed for sampling timesteps for calibration. Overall, the method demonstrates superior generation quality results over such baselines as LSQ, PTQ4DM and Q-Diffusion.
Pruning/Sparsity
- Effective Interplay between Sparsity and Quantization: From Theory to Practice by Google and EcoCloud (https://arxiv.org/pdf/2405.20935). Authors provide the theoretical analysis of how sparsity and quantization interact. Mathematical proofs establish that applying sparsity before quantization (S → Q) is the optimal sequence for compression. Authors demonstrate that sparsity and quantization are not orthogonal operations. Combining them introduces additional errors beyond the sum of their individual errors. They validate theoretical findings through experiments covering a diverse range of models, including prominent LLMs (OPT, LLaMA) and ViTs. The code will be published at: https://github.com/parsa-epfl/quantization-sparsity-interplay.
- Prompt-prompted Mixture of Experts for Efficient LLM Generation by CMU (https://arxiv.org/pdf/2404.01365). Authors introduce GRIFFIN, a training-free MoE that selects unique FF experts at the sequence level for efficient generation across a plethora of LLMs with different non-ReLU activation functions. This is possible due to a critical observation that many trained LLMs naturally produce highly structured FF activation patterns within a sequence, which we call flocking. Despite the method’s simplicity, it shows with 50% of the FF parameters, GRIFFIN maintains the original model’s performance with little to no degradation on a variety of classification and generation tasks, all while improving latency (e.g. 1.25× speed-up in Llama 213B on an NVIDIA L40). Code is available at https://github.com/hdong920/GRIFFIN.
- Sparse maximal update parameterization: A holistic approach to sparse training dynamics by Cerebras Systems (https://arxiv.org/pdf/2405.15743).This paper addresses the common issue in sparse training where hyper parameters from dense training are reused, leading to suboptimal convergence, and requiring extensive tuning for different sparsity ratios. The researchers introduce a novel sparse training methodology called Sparse Maximal Update Parameterization (SuPar), which extends the maximal update parameterization (uP)to sparse training. SuPar involves reparameterizing (see Table 1) weight initialization and learning rates relative to changes in sparsity, effectively preventing exploding or vanishing signals and maintaining stable activation, gradient, and weight update scales across varying sparsity levels and model widths. SuPar reparameterization is remarkable, it allows zero-shot hyperparameter transfer, i.e. practitioners can now tune small proxy models(dense/sparse) and transfer optimal HPs directly to models at scale for any model sparsity, thus enhancing the efficiency and reducing the cost of sparse model development. Experiments demonstrate that SμPar sets the Pareto frontier best loss across all sparsities and widths, including large dense model with width equal to GPT-3 XL.
- Sparse Expansion and Neuronal Disentanglement by MIT, IST Austria, Neural Magic (https://arxiv.org/pdf/2405.15756). Sparse Expansion is an approach of converting dense LLMs to mixture of sparse experts to attain inference efficiency. The method begins with applying dimensionality reduction (PCA) on the inputs of FFN linear layers, followed by a k-means clustering. The intuition is that tokens within a cluster share a sparse expert better without significant distortion. SparseGPT is then used to create a sparse expert for each cluster group. During inference, the PCA and k-means models act as routers, directing tokens to the appropriate sparse expert based on their cluster. While this increases the overall model size, acceleration is achieved through the conditional execution of experts and the sparse execution of these experts, with minimal cost for the routers. The paper includes layer-wise speedup benchmarks and shows that Sparse Expansion outperforms other one-shot sparsification approaches in perplexity for the same inference FLOP budget per token. A significant portion of the paper is dedicated to the concept of neuron entanglement, explaining, and quantifying the efficacy of sparse expansion.
- MULTIFLOW: Shifting Towards Task-Agnostic Vision-Language Pruning by University of Trento and Cisco Research (https://arxiv.org/pdf/2404.05621). Authors highlight that existing techniques for pruning of Visual-Language models(VLMs) are task-specific and propose a task-agnostic method for pruning VLMs. The proposed Multimodal Flow Pruning framework has the following properties: (1) the importance of a weight is computed based on saliency of the neurons it connects; and (2) parameters are pruned considering features of which modality they are used to compute allowing to avoid pruning too much from a single modality and too little from another. Experiments show that the proposed MULTIFLOW method outperforms recent more sophisticated competitors.
Other methods
- Flash Diffusion: Accelerating Any Conditional Diffusion Model for Few Steps Image Generation by Jasper Research (https://arxiv.org/pdf/2406.02347). The paper proposes a LoRA-compatible distillation method aiming at reducing the number of sampling steps required to generate high-quality samples from a trained diffusion model. Authors emphasize the versatility of the method through an extensive experimental study across various tasks (text-to-image, image inpainting, super-resolution, face-swapping), diffusion model architectures (SD1.5, SDXL and Pixart-α) and illustrate its compatibility with adapters. The method is relatively lightweight and can optimize SD1.5 model with 2 Nvidia H100 80GB with 13 hours of fine-tuning. Code is available at https://github.com/gojasper/flash-diffusion.
- GaLore: Memory-Efficient LLM Training by Gradient Low-Rank Projection by California Institute of Technology, Meta AI, University of Texas at Austin, and Carnegie Mellon University (https://arxiv.org/pdf/2403.03507). The paper introduces a Gradient Low-Rank Projection (GaLore), a training strategy that allows full-parameter learning but is more memory-efficient than common low-rank adaptation methods such as LoRA. The idea is to use PCA after a number of training steps to obtain a gradient projection matrix and use it to get a low-rank gradient matrix that is used for weights update. The approach reduces memory usage by up to 65.5% in optimizer states while maintaining both efficiency and performance for pre-training. 8-bit GaLore further reduces optimizer memory by up to 82.5% and total training memory by 63.3%, compared to a BF16 baseline. It demonstrates the feasibility of pre-training a 7B model on consumer GPUs with 24GB memory. The code is available at: https://github.com/jiaweizzhao/GaLore.
- MiniCache: KV Cache Compression in Depth Dimension for Large Language Models by ZIP Lab of Monash and Zhejiang University (https://arxiv.org/pdf/2405.14366).The authors propose a training-free KV cache compression technique by merging KV tokens across every two consecutive transformer layers, based on the observation that KV tokens are highly similar across depth, especially from the middle to the last transformer layers. Specifically, a pair of K/V projections from two consecutive layers can be encoded into respective scaling factors and a shared directional vector computed via Spherical Linear Interpolation(SLERP). To address the information loss from merging dissimilar tokens, the algorithm uses angular-based distance to filter KV positions for retention. The algorithm is straightforward, involving calibration of only two hyperparameters, and it has demonstrated to enhance a 4X compressed KV cache by4-bit quantization to over 5X compression while retaining reasonable accuracy of instruction-tuned Mistral, LLama2-7B across benchmarks.
- Scalable MatMul-free Language Modeling by University of California, Soochow University, LuxiTech (https://arxiv.org/pdf/2406.02528). Authors develop a MatMul-free language model by using additive operations in dense layers and element-wise Hadamard products for self-attention-like functions. Specifically, ternary weights eliminate MatMul in dense layers, similar to BNNs. To remove MatMul from self-attention, they optimize the Gated Recurrent to rely solely on element-wise products and show that this model competes with state-of-the-art Transformers while eliminating all MatMul operations. To quantify the hardware benefits of lightweight models, the authors provide an optimized GPU implementation in addition to a custom FPGA accelerator. By using fused kernels in the GPU implementation of the ternary dense layers, training is accelerated by 25.6% and memory consumption is reduced by up to 61.0% over an unoptimized baseline on GPU. Furthermore, by employing lower-bit optimized CUDA kernels, inference speed is increased by 4.57 times, and memory usage is reduced by a factor of 10 when the model is scaled up to 13B parameters. The code is available at https://github.com/ridgerchu/matmulfreellm.
- Unlocking Efficiency in Large Language Model Inference: A Comprehensive Survey of Speculative Decoding by Hong Kong Polytechnic, Peking University, Microsoft Research Asia and Alibaba (https://arxiv.org/abs/2401.07851). While LLMs are proliferating over the past two years, Speculative Decoding (SD) has emerged as a crucial paradigm to accelerate autoregressive generation. This survey is among the first to provide a comprehensive introduction and overview of the state of the art in SD, highlighting key developments in this space. A main contribution of this work is the introduction of Spec-Bench, a unified benchmark for evaluating SD methods across standardized subtasks such as multi-turn conversation, summarization, RAG, translation, question answering, and mathematical reasoning. The codes and benchmarks for various SD methods on RTX 3090 and A100 GPUs are accessible for further exploration and validation.
- Speculative Decoding via Early-exiting for Faster LLM Inference with Thompson Sampling Control Mechanism by Meituan and Meta AI (https://arxiv.org/pdf/2406.03853).The paper introduces an early-exiting framework for generating draft tokens, which allows a single LLM to fulfill the drafting and verification stages. The model is trained using self-distillation. The authors conceptualize the generation length of draft tokens as a multi-armed bandit problem and propose a control mechanism based on Thompson Sampling, which leverages sampling to devise an optimal strategy. They conducted experiments on three benchmarks and showed that the method can significantly improve the model’s inference speed.
- LayerSkip: Enabling Early Exit Inference and Self-Speculative Decoding by Meta, University of Toronto, Carnegie Mellon University, University of Wisconsin-Madison, Dana-Farber Cancer Institute (https://arxiv.org/pdf/2404.16710).Authors research the idea of early exit in LLMs for speculative decoding. First, during training, they apply layer dropout, with low dropout rates for earlier layers and higher dropout rates for later layers, and an early exit loss where all transformer layers share the same exit. Second, during inference, they show that this training recipe increases the accuracy of early exit at earlier layers, without adding any auxiliary layers or modules to the model. Third, they present a self-speculative decoding solution where we exit at early layers and verify and correct with remaining layers of the model. They run experiments on different Llama model sizes on different types of training: pretraining from scratch, continual pretraining, finetuning on specific data domain, and finetuning on specific task, and show speedups of up to 2.16× on summarization for CNN/DM documents, 1.82× on coding, and 2.0× on TOPv2 semantic parsing task.
Software
- INT4 Decoding GQA CUDA Optimizations for LLM Inference by Meta(https://pytorch.org/blog/int4-decoding).The authors provide a comprehensive study and ten practical steps, including KV-cache quantization, to improve the performance of Grouped-query Attention. All these optimizations result in performance improvements of up to 1.8x on the NVIDIA A100 GPU and 1.9x on the NVIDIA H100 GPU.
- torchao: PyTorch Architecture Optimization by Meta (https://github.com/pytorch/ao). PyTorch library for quantization and sparsity. Currently-available features contain full models quantization, INT8, INT4, MXFP4,6,8 weight-only quantization and efficient model fine-tuning with GaLore method.
- Introducing Apple’s On-Device and Server Foundation Models by Apple (https://machinelearning.apple.com/research/introducing-apple-foundation-models). Apple has established a set of pre-trained and optimized models for its HW. The claim is that 3B LLM model can be run at 30t/s on iPhone 15 Pro. In terms of optimizations that are being used, authors claim weight palletization to 2 and 4 bits, quantization of embeddings and activations and efficient Key-Value (KV) cache update. They use their own AXLearn library built on top of JAX and XLA for model pre-training and fine-tuning.
- BitBLAS by Microsoft (https://github.com/microsoft/BitBLAS).A library to support mixed-precision BLAS operations on GPUs. BitBLAS aims to support efficient mixed-precision DNN model deployment, especially the quantization in large language models (LLMs), for example, the 𝑊4𝐴16 in GPTQ, the 𝑊2𝐴16 in BitDistiller, the 𝑊2𝐴8 in BitNet-b1.58.
Optimizing Speech Emotion Recognition: SpeechBrain Meets OpenVINO™
Authors: Pradeep Sakhamoori, Ravi Panchumarthy
Introduction
Want to analyze emotions in speech recordings but keep your AI application lean and mean? This blog post dives into combining the power of SpeechBrain's pre-trained emotion recognition models with OpenVINO™ for efficient inference. We'll explore how to leverage SpeechBrain's"emotion-recognition-wav2vec2-IEMOCAP" model and optimize it for blazing-fast performance using OpenVINO™.
Getting Started with SpeechBrain
SpeechBrain is a powerful open-source toolkit for developing Conversational AI technologies, including speech recognition, speaker recognition, and emotion recognition. In this blog post, we'll explore using SpeechBrain's pre-trained "emotion-recognition-wav2vec2-IEMOCAP" model to classify emotions in speech recordings and optimize this model for efficient inference using the OpenVINO™toolkit.
SpeechBrain's Emotion Recognition Model and the IEMOCAP Dataset:
The "emotion-recognition-wav2vec2-IEMOCAP"model is fine-tuned on the IEMOCAP dataset, which contains approximately 12hours of audiovisual data with recordings of dialogues by 10 speakers portraying various emotions, including angry, excited, fear, sad, surprised, frustrated, happy, disappointed, and neutral.
The model is based on the wav2vec2 architecture, combining convolutional and residual blocks. The embeddings are extracted using attentive statistical pooling, and the system is trained with Additive Margin Softmax Loss.
Loading Custom Models with SpeechBrain's foreign_class Function:
The ‘foreign_class’ function in SpeechBrain is a utility that allows you to load and use custom PyTorch models within the SpeechBrain ecosystem. It provides a convenient way to integrate external or custom-built models into SpeechBrain's inference pipeline without modifying the core SpeechBrain codebase.
Here’s how you can load and use the “emotion-recognition-wav2vec2-IEMOCAP”model with foreign_class:
classifier = foreign_class(
source="speechbrain/emotion-recognition-wav2vec2-IEMOCAP",
pymodule_file="custom_interface.py",
classname="CustomEncoderWav2vec2Classifier"
)
# Initialize wav2vec2 torch model
torch_model = classifier.mods["wav2vec2"].model
# Run Inference
out_prob, score, index, text_lab = classifier.classify_file("speechbrain/emotion-recognition-wav2vec2-IEMOCAP/anger.wav")
print(f"Emotion Recognition with SpeechBrain PyTorch model: {text_lab}")
- source: This argument specifies the source or location of the pre-trained model checkpoint. In this case, "speechbrain/emotion-recognition-wav2vec2-IEMOCAP"refers to a pre-trained model checkpoint on the Hugging Face Hub.
- pymodule_file: This argument is the path to a Python file containing the definition of your custom PyTorch model class. In this example, "custom_interface.py" is the Python file name defining theCustomEncoderWav2vec2Classifier class.
- classname: This argument specifies the name of the custom PyTorch model class defined in the pymodule_file. In this case,"CustomEncoderWav2vec2Classifier" is the name of the class that extends SpeechBrain's Pretrained class and implements the necessary methods for inference.
- classifier.classify_file: This is the Inference function call for emotion classification on an audio file.
Optimizing with OpenVINO™
To enhance the performance of our emotion recognition model, we leverage the OpenVINO™ toolkit. OpenVINO™ empowers developers to write code once and deploy it across diverse Intel® hardware and environments. This includes on-premises, on-device, cloud, and browser deployments. You can also configure performance optimization parameters based on the use case, hardware, and target performance (latency/throughput). For more details, refer to OpenVINO runtime optimizations.
Refer to the OpenVINO SpeechBrain notebook for full code implementation.
Following are the key steps to optimize the model using OpenVINO™:
Step 1: Convert the model to OpenVINO format:
Below is a code snippet illustrating the conversion of the SpeechBrain PyTorch model to OpenVINO IR format using openvino.convert_model Python API.
import openvino as ov
ov_model = ov.convert_model(torch_model,example_input=input_tensor)
Step 2: Run Inference with OpenVINO™ Inference Engine:
After converting the model to OpenVINO format, compile the converted model for your target device and run inference. Below is a sample inference code snippet from the OpenVINO SpeechBrain notebook. For details on Inference Devices and Modes, see optimize-inference.
# OpenVINO Compiled model
compiled_model = core.compile_model(ov_model, device.value)
# Perform model inference
output_tensor = compiled_model(wavs)[0]
Conclusion:
Integrating SpeechBrain’s pre-trained models with custom interfaces and optimizing them using OpenVINO™ can significantly enhance the efficiency of your AI applications. This approach not only improves model performance but also ensures seamless deployment across different hardware platforms. By following the steps outlined above, you can build a robust SpeechBrain Emotion Recognition Model optimized with OpenVINO™ runtime that is both powerful and efficient.
Call to Action:
- Explore OpenVINO SpeechBrain notebook for detailed code implementation.
- Explore OpenVINO documentation.
- Consider giving a star to OpenVINO toolkit and OpenVINO notebooks repositories if you find them helpful.
Notices and Disclaimers:
Performance varies by use, configuration, and other factors. Learn more at www.intel.com/PerformanceIndex.Performance results are based on testing as of dates shown in configurations and may not reflect all publicly available updates. No product or component can be absolutely secure. Intel technologies may require enabled hardware, software or service activation.
The products described may contain design defects or errors known as errata which may cause the product to deviate from published specifications. Current characterized errata are available on request.